Human computer interaction – 2023 Winning project
Can Word-level Quality Estimation Inform and Improve Machine Translation Post-editing?
Introduction
Machine Translation (MT) has become an integral part of modern translation workflows, and post-editing (PE) of MT outputs is a common practice in the language services industry. Translators often work with Computer-Aided Translation (CAT) tools that incorporate MT suggestions, improving productivity while maintaining high-quality standards.
In this context, quality estimation (QE) methods have been proposed to further direct the translators’ focus to machine-translated outputs needing revision. QE techniques are mainly applied to text paragraphs or sentences to obtain a score showing the overall translation quality of the MT output, similar to fuzzy matches in translation memories (TMs). Such coarse-grained QE methods are nowadays frequently employed in CAT workflows to determine whether an MT proposal should be presented to the translator or discarded. However, the potential of more fine-grained quality estimation techniques remains largely unexplored in professional settings.
To our knowledge, Unbabel’s Quality Intelligence API is the only industrial solution in this area.
Beyond segment-level assessment, word-level quality estimation presents a promising avenue for directing post-editors’ attention to specific problematic regions within a sentence. For example, consider the following machine-translated sentence:
“The company announced its new products line, which will be available in stores from start of next month.”
A word-level QE system might detect “products” and “from start of” as ungrammatical, prompting the post-editor to verify these specific phrases and correct potential errors.
While this task is vastly more challenging from a machine learning perspective due to its fine-grained nature, it could substantially benefit the post-editing process. Despite the potential advantages, little research has been done to assess the practical usefulness of word-level QE for professional translators. This project aims to bridge that gap by investigating how translation error predictions can be effectively presented in a translation interface and how their quality influences post-editors productivity and experience.
Exploiting the Internals of MT Models for Error Prediction
Word-level QE annotations are typically produced by models trained on large amounts of annotated data to mimic the choices of professional quality annotators, an approach commonly referred to as supervised word-level QE. While effective, this method is limited by the availability of high-quality training data and may not generalize well across different domains or language pairs. On the other hand, unsupervised word-level QE methods leverage internal information produced by the neural machine translation (NMT) model used to generate the outputs. Traditionally, much of this internal information is ignored during the translation generation process. However, recent studies have shown that so-called ‘model internals’ can be highly useful in identifying translation issues such as additions, omissions and hallucinations without additional training data or external QE models (Guerreiro et al., 2022, Dale et al., 2023) .
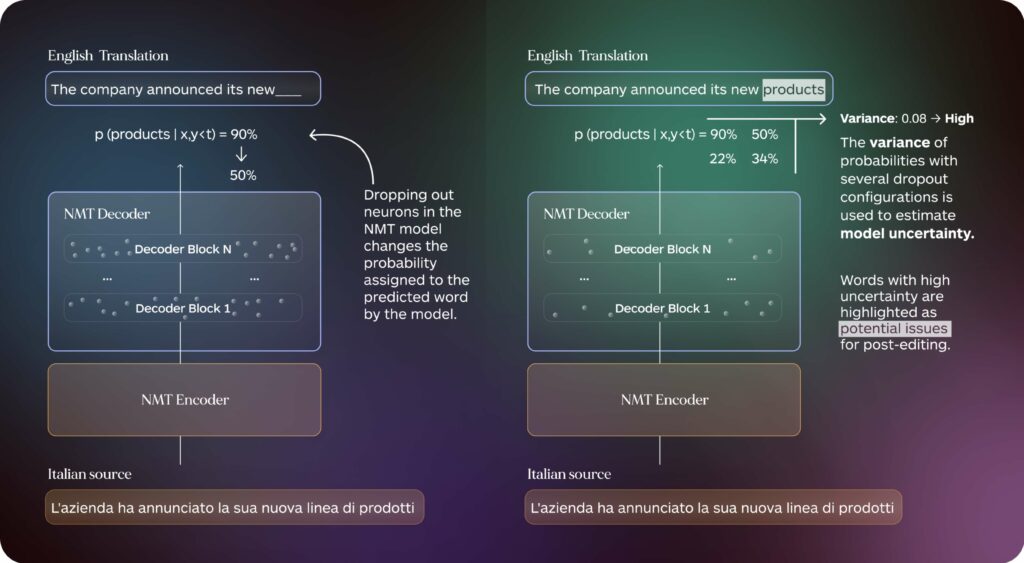
Figure 1: Overview of the process for creating unsupervised word-level QE highlights we used in our study.
This project aims to compare the accuracy of supervised and unsupervised approaches in detecting potential issues in MT outputs and to assess their downstream impact on post-editing productivity and enjoyability. More broadly, our research aims to improve the efficiency and satisfaction of human-machine collaboration in translation workflows.
Study Design
Setup
Our study, nicknamed “Quality Estimation for Post-Editing” (QE4PE), involved 24 professional translators, 12 per translation direction, post-editing a collection of 50 English documents machine-translated into Italian and Dutch. Texts were translated using NLLB 3.3B, a strong open-source multilingual machine translation system by Meta AI. The documents employed for our evaluation were selected from a mix of biomedical research abstracts and social media posts collected for the Workshop on Machine Translation’s (WMT) 2023 evaluation campaign.
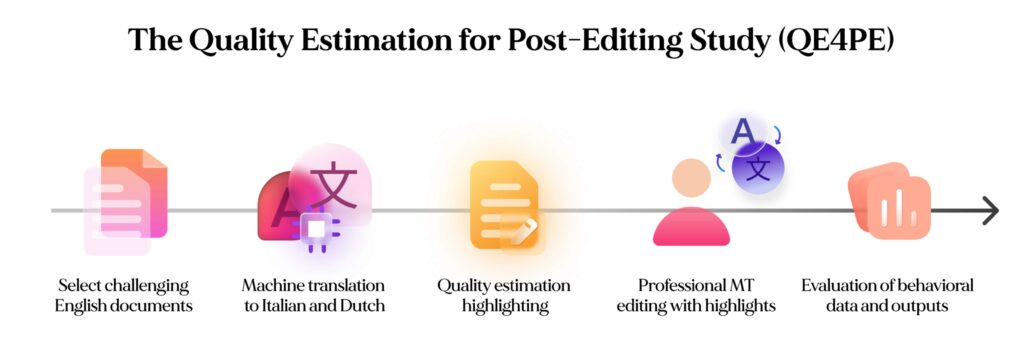
Figure 2: A schematic overview of the QE4PE study.
To evaluate the effectiveness of word-level QE, we developed a simple online interface that supports the editing of highlighted texts. This interface allowed us to present machine-translated content to the participants with QE-based highlights at two degrees of severity (minor and major), enabling us to track and analyze their interactions, editing patterns, and overall performance across different conditions.
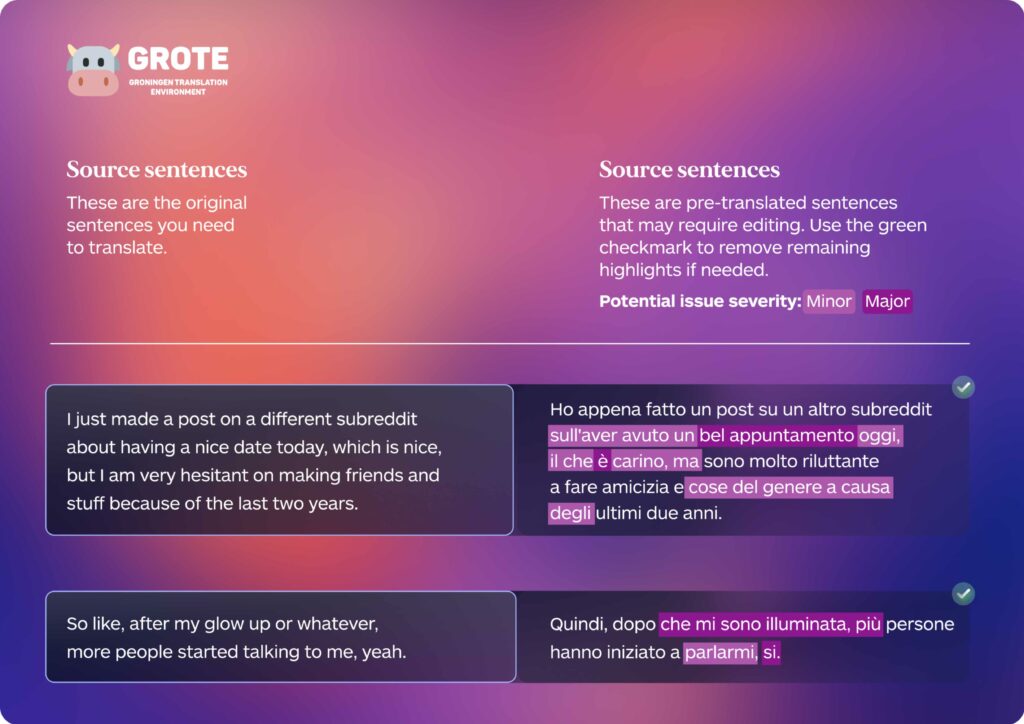
Figure 3: Example of the GroTE web interface used for this study, showing two machine-translated passages with highlighted spans marking potential issues.
Editing Modalities
After a pre-task aimed at familiarizing translators with the interface, the QE highlights and the type of data, we conducted the main task in four different settings to assess the downstream impact of quality highlights:
- No Highlights: The MT outputs requiring post-editing are presented without any highlighting. This setting acts as a baseline.
- Oracle Highlights: Highlights are added over spans in the MT output that were post-edited by at least 2 out of 3 professional translators in a previous editing stage. This can be regarded as a best-case scenario for systems trained on human edits.
- Supervised Highlights: Highlights are produced by XCOMET-XXL, the current state-of-the-artsystem trained for word-level QE.
- Unsupervised Highlights: Highlights are produced by selecting the spans for which the NLLB MT model showed higher uncertainty according to Monte Carlo Dropout probability variance, a popular technique to estimate uncertainty using model internals.
By comparing the results across these settings, we aim to quantify the impact of different QE approaches on post-editing productivity, the quality of highlights and final translations, and the translators’ subjective experience. This comprehensive evaluation will provide valuable insights into the practical benefits of integrating word-level QE into professional translation workflows.
Preliminary Results
While we are still in the process of completing our analyses on the collected data, here we present some initial findings based on the assessment of post-editing productivity, quality, and enjoyability across the four study conditions.
Productivity: Do Highlights Make Post-editors Faster?
We measured the average time translators spent editing machine-translated documents across the four settings to evaluate the impact of different QE highlights on post-editing productivity.
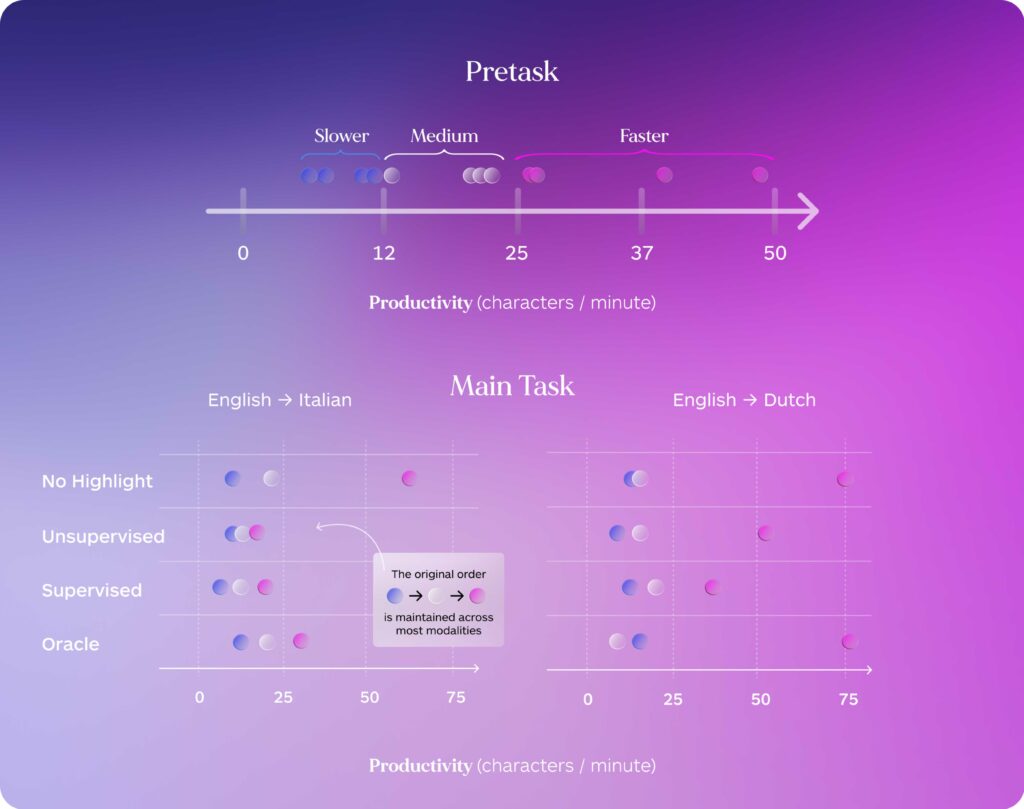
Figure 4: Top: Translators’ editing speed is initially assessed on a pretask, and each post-editor (represented by a colored dot) is assigned to an editing modalities according to their speed to maintain balanced groups. Bottom: Productivity across editing modalities for the two tested translation directions. Translators tend to maintain their productivity ordering (Slower → Medium → Faster) across all editing modalities, suggesting the effect of individual speed remains predominant, regardless of editing modality.
Our preliminary results show that highlight modality is less predictive of post-editing productivity compared to the individual editing speed of each translator. Figure 4 illustrates how translators that are found to be faster on a smaller subset of translations edited before the main task (Pretask) remain faster than their colleagues regardless of the editing modality. This highlights the need to account for individual editing speed differences when evaluating the productivity impact of word-level quality estimation.
Quality: Do Highlights Help Translators Detect Errors?
While a manual assessment of translation quality is currently ongoing, as a preliminary step we use the referenceless XCOMET metric as a proxy to evaluate the accuracy and fluency of the post-edited translations.
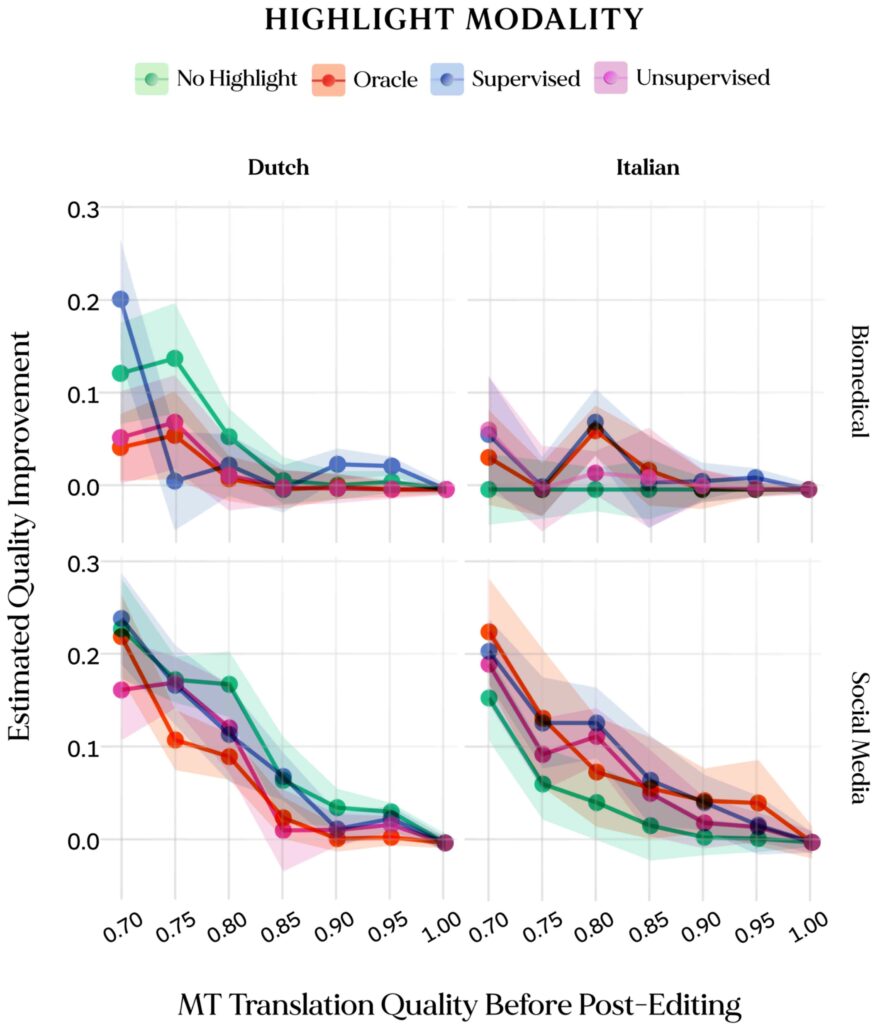
Figure 5: Translation quality improvements for post-edited texts starting from MT outputs of variable quality (x axis). Initial MT quality and post-editing improvements were estimated with XCOMET-XXL across two target languages (columns) and domains (rows). Scores are the median across all post-editors per modality (n=3 for each target language and modality combination).
Our preliminary investigation in the effect of editing modalities on output quality shows that overall quality of post-edited outputs across all highlight modalities remains in line with regular post editing without highlights, leading to improved quality only in some settings (e.g. in the Social Media and Biomedical domains for the English -> Italian translation direction).
From Figure 5, we also observe that, according to our proxy metric, quality gains from post-editing are consistently positive across all settings. We also note that gains for lower-quality MT outputs are especially prominent in the Social Media domain, with diminishing gains for higher-quality translations across all settings.
This suggests that gains stemming from the use of QE highlights might not be observed at a macroscopic level, but might become relevant for tricky cases with less evident errors.To validate this hypothesis, we evaluate a small set of examples containing manually-crafted critical errors that were inserted in some of the MT outputs, and were frequently highlighted by several word-level QE techniques. On this subset, we find that translators having access to highlights were 16-25% more likely to correct the critical errorsthan translators editing without highlights, suggesting that highlights can help attract the editor’s attention to problematic elements in the translation.
Enjoyability: Do Translators Like to Work with Word-Level Highlights?
We administered a post-task questionnaire to assess translators’ subjective experience, including their satisfaction with the various highlight types and overall ease of the post-editing process.
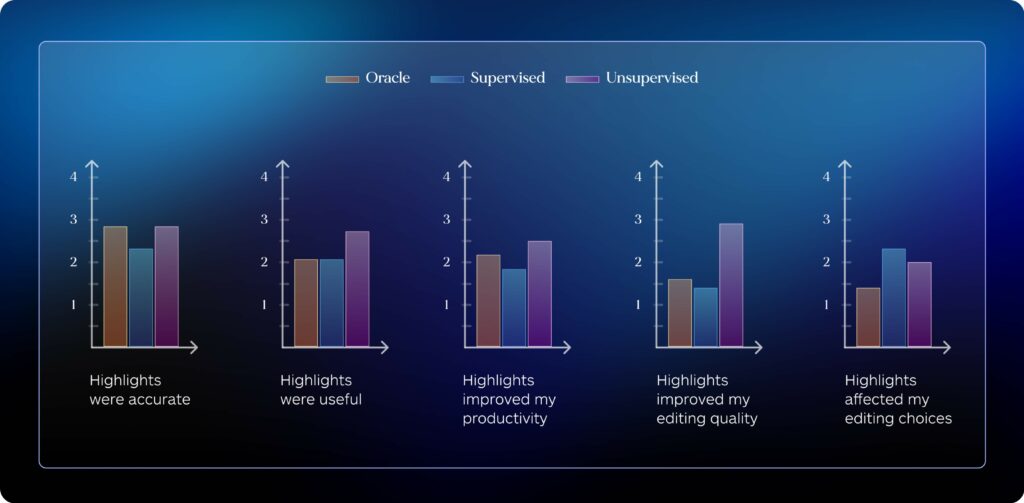
Figure 6: Ratings per editing modality for statements about highlights collected in the post-task questionnaire. Scores are averaged across all post-editors in each modality across both translation directions (n = 6 per modality), ranging between 1 (Strongly disagree) and 5 (Strongly agree).
While some translators found the presence of highlights useful (e.g. one translator stated that highlights “[…] helped me a lot, making the editing process faster and somehow easier.”), the general sentiment was that highlights were “[…] too confusing […]” and “[…] more of an eye distraction […]”, noting that generally they were “[…] not quite accurate enough to rely on them as a suggestion”. Importantly, these comments were found prevalent across all editing modalities, including editing with highlights based on previous human post-edits, despite the quality results highlighted in the previous section. This suggests that even error highlights obtained from several human editors might not fully capture problematic elements or more general mistakes (as one translator puts it, “[…] sometimes it happened that you had to edit not just the highlighted word but the entire sentence”), despite the high quality of initial translations.
The median XCOMET-XXL QE score of MT outputs across both translation directions was 0.952, with a standard deviation of 0.11
Interestingly, unsupervised highlights produced from MT model internals were generally rated more useful than their counterparts, including those derived from previous human post-edits (Human Edits in Figure 6). This suggests that the difference in quality between various word-level QE approaches might not be immediately evident, and improved usability might outweigh accuracy gains in the eyes of post-editors.
Conclusion
This concludes our introduction to the QE4PE and our preliminary findings regarding the impact of word-level highlights on productivity, quality and enjoyability for MT post-editing. Our preliminary findings paint a nuanced picture, showing the potential of word-level QE in improving the quality of post-edited outputs while also underscoring the need for further usability improvements to make this technique viable in professional translation workflows. Importantly, the findings of our study pertain to a specific translation direction for which high-quality MT is available, and our evaluation focused specifically on professional post-editors with extensive experience, which might benefit less from the guidance provided by word-level QE methods. In light of this, all contents of this study, including our online interface and the collected post-editing logs, will be made available to the research community to enable future assessments for different translation direction, with alternative editor profiles (e.g. professionals, translation students, L2 learners) and new QE techniques.
Impact
The project’s goal is two-fold, addressing both practical application and technical innovation in word-level quality estimation for machine translation. Our user study described in this article represents a first step for assessing the real-world impact of word-level QE in post-editing workflows and providing valuable insights into how translators interact with and benefit from fine-grained quality estimation cues, potentially reshaping best practices in the industry. As a next step, we will experiment with novel unsupervised techniques for word-level QE exploiting the internal information of neural machine translation models. We aim to create more faithful and explainable quality estimation methods that can be readily applied to new languages and domains without extensive retraining.
Team Members
Gabriele Sarti
PhD Student, University of Groningen
Gabriele’s doctoral project as a member of the InDeep Dutch consortium focuses on devising new approaches and applications of explainable AI in neural machine translation. His research is particularly concerned with bridging the gap between theoretical insights about the inner workings of neural network-based language models to improve human-AI collaboration. He was previously a research intern on the Amazon Translate team and worked as a research scientist at the Italian startup Aindo.
Arianna Bisazza
Associate Professor, University of Groningen
Arianna’s research aims to identify the intrinsic limitations of current language modeling paradigms and improve machine translation quality for challenging language pairs. She has been working towards better MT algorithms for fourteen years and was recently awarded sizeable grants for her research on language models interpretability and the development of conversational and cognitively-plausible learning systems by the Dutch Research Council.
Vilém Zouhar
PhD Student, ETH Zürich
Vilém researches non-mainstream machine translation, evaluation, quality estimation, and human-computer interaction. In recent studies with collaborators from the WMT community, he explored the pitfalls of automatic evaluation and their solution. His PhD project at ETH Zürich is about how to make human and automatic evaluation of machine translation and NLP in general more robust, higher-quality and economical.
Malvina Nissim
Full Professor, University of Groningen
Malvina is the chair of Computational Linguistics and Society at the University of Groningen, The Netherlands. Her research interests span several aspects of automatic text analysis and generation, with a recent focus on writing style and reformulation. She is the author of 100+ publications in international venues, regularly reviews for major conferences and journals, organizes and chairs large-scale scientific events, and is a member of the ACL Ethics Committee.
Ana Guerberof Arenas
Ana Guerberof Arenas
Associate Professor, University of Groningen
Ana was recently awarded an ERC Consolidator grant for the INCREC project, aiming to explore the creative process in literary and audio-visual translation and its intersection with technology. With more than 23 years of experience in the translation industry, she has authored several articles and chapters on MT post-editing, translator training, and ethical considerations in MT and AI.
Grzegorz Chrupała
Associate Professor, University of Tilburg
Grzegorz is interested in computation in biological and artificial systems and their connections. His research focuses primarily on computational models of learning (spoken) language in naturalistic multimodal settings and the analysis of representations emerging in deep learning architectures. He regularly serves as Senior Area Chair for major NLP and AI conferences such as ACL and EMNLP. He was one of the creators of the popular BlackboxNLP Workshop on Analyzing and Interpreting Neural Networks for NLP.
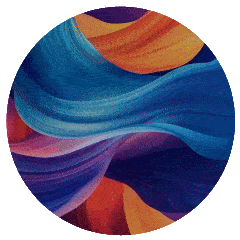
Imminent Science Spotlight
Academic lectures for language enthusiasts
Imminent Science Spotlight is a new section where the next wave of language, technology, and Imminent discoveries awaits you. Curated monthly by our team of forward-thinking researchers, this is your go-to space for the latest in academic insight on transformative topics like large language models (LLM), machine translation (MT), text-to-speech, and more. Every article is a deep dive into ideas on the brink of change—handpicked for those who crave what’s next, now.
Discover more here!Winning Projects from the Latest Edition

Human-Computer Interaction
The impact of Speech Synthesis on cognitive load, productivity, and quality during post-editing machine translation (PEMT).
Dragoș Ciobanu
University of Wien
The increased fluency of neural machine translation (NMT) output recorded in certain language pairs and domains justifies its large-scale deployment, yet professional translators are still cautious about adopting this technology. Among their main concerns is the already-documented “NMT fluency trap” that causes translators to miss significant Accuracy errors masked by the NMT output’s high fluency.
The Human and Artificial Intelligence in Translation (HAITrans) research group at the University of Vienna has been investigating the potential of speech technologies—synthesis and recognition—to improve the quality of professional and trainee translators’ work. This project will specifically build on experiments involving speech synthesis in the revision and post-editing processes, which show a superior level of Accuracy error detection and correction when synthesis is present. Given findings that revising with speech synthesis does not have a negative impact on revisers’ cognitive load, the researchers will use our eye-tracking lab to investigate cognitive load and productivity when post-editing with sound versus in silence. Should the PEMT findings mirror our work on revision, the expectation is that translators will feel reassured that, when integrating speech synthesis into their PEMT workflows, this technology will help them avoid the NMT fluency trap without compromising productivity or increasing cognitive load.
Neuroscience of language
The neuroscience of translation. Novel and dead metaphors processing in native and second-language speakers.
Martina Ardizzi & Valentina Cuccio
University of Parma
Language development, production, and comprehension cannot be divorced from lived, corporeal experience to the point that they are literally embodied (Cuccio & Gallese, 2018). A growing body of work has indeed implicated the sensorimotor system in semantic comprehension of native language. Unfortunately, the embodied character of a second language has been severely under-investigated. The present project will fill this gap by testing, in a functional magnetic resonance imaging (fMRI) study, how the comprehension of dead and novel metaphors involves the sensorimotor system in native and second-language speakers. Recent findings have shown that dead metaphors—the ones repeated ad nauseam whose literal meaning is no longer accessed—are processed outside the sensorimotor system (Yang & Shu, 2016). The expectation is that dead metaphors will recruit the sensorimotor system differently in native speakers compared to second-language speakers. A multidisciplinary approach merging the competencies of Valentina Cuccio (PI, Philosopher, Assistant Professor, University of Messina) author of recent theoretical and empirical advances in the embodied approach to language especially in metaphors understanding, with the skills of Martina Ardizzi (co-PI, Neuroscientist, Assistant Professor, University of Parma) expert in fMRI specifically applied to the role of the sensorimotor system in cognition. The NET project will lead to two scientific papers published in open-access journals. Furthermore, the novel application of an embodied approach to translation may provide new insights on how to improve the disembodied AI translations. Indeed, although worldwide there are thousands of different languages, speakers universally share the same corporeal experiences that could ultimately ground linguistic meaning.
Machine learning algorithms for translation
Incremental parallel inference for machine translation
Andrera Santilli
La Sapienza, University of Rome
Machine translation works with a de facto standard neural network called Transformer, published in 2017 by a team at Google Brain. The traditional way of producing new sentences from the Transformer is one word at a time, left to right; this is hard to speed up and parallelize. Andrea Santilli and his PhD supervisor Emanuele Rodolà, at the Sapienza University of Rome, are specialists in neural network architectures. They spotted that a similar problem is solved in image generation by using “incremental parallel processing,” a technique that refines an image progressively rather than generating it pixel by pixel, yielding speedups of 2–24×. They propose to port this method to Transformers, using clever linear algebra tricks to make it happen. At Translated, we hope that this technique and other similar ones makes machine translation less expensive and therefore accessible to a greater number of use cases, and ultimately more people.
Language Data
YorùbáVoice
Kọ́lá Túbọ̀sún
Independent Researcher
Yoruba is one of the most widely spoken languages in Africa, with 46 million first- and second-language speakers. Yet there is hardly any language technology available in Yoruba to help them, especially illiterate or visually impaired people, who would benefit most. Translated’s vision is to build a world where everyone can understand and be understood. In this project, the team will work on “everyone,” developing speech technology in Yorùbá. The team is headed by Kola Tubosun, the founder of the YorubaNames, and four computer scientists and language enthusiasts with an excellent scientific track record, with publications at Interspeech, ACL, EMNLP, LREC, and ICLR. As a first action, aligned voice and text resources will be recorded professionally in a quality usable to produce text-to-speech systems. After donating this data to the Mozilla Common Voice repository under a Creative Commons license, further speech data will be collected from volunteers online. To increase the quality of the text, the team has already developed a diacritic restoration engine.
Language Economics
T-Index
Luciano Pietronero, Andrea Zaccaria, Giordano de Marzo
Enrico Fermi Research Center Team
Understanding which countries and languages dominate online sales is a key question for any company wishing to translate its website. The goal of this research project is to complement the T-Index by developing new tools capable of identifying emerging markets and opportunities, thereby predicting which languages will become more relevant in the future for a specific product in a specific country. As a first step, the team will rely on the Economic Fitness and Complexity algorithm to determine which countries will undergo major economic expansion in the next few years. Leveraging network science and machine learning techniques to predict the products and services that growing economies will start to import.

Human-Computer Interaction
Humanity of Speech
Pauline Larrouy-Maestri and team
Max Planck Institute
Synthetic speech is everywhere, from our living room to the communication channels that connect humans all over the world. Text-to-speech (TTS) tools and AI voice generators aim to create intelligible, realistic sounds to be understood by humans. Whereas intelligibility is generally accomplished, the voices do not sound natural and lack “humanity,” which affects users’ engagement in human-computer interaction. In this project, the team aims to understand what a “human” voice is—a crucial issue in all domains relative to language, such as computer, psychological, biological, and social sciences. To do so, they will 1) investigate the timbral and prosodic features that are used by listeners to identify human speech, and 2) determine how “humanness” is categorized and transmitted. Concretely, we (my group and I) plan to run a series of online experiments using methods from psychophysics. The research will focus both on the speech signal—through extensive acoustic analyses and manipulation of samples—as well as on the cognitive and social processes involved. As can be seen on Pauline’s website (https://pauline-lm.github.io/), she is a senior researcher at the Max Planck Institute, with a master’s in speech science, PhD in cognitive psychology, and postdoctoral work in neuroscience. The extensive expertise of my team in the topics involved in the proposed project (see the articles about emotional prosody, auditory perception, acoustics, voice, etc., published in high-impact, peer-reviewed journals) makes us the ideal candidates to successfully investigate the “humanity of speech.”
Usability of explainable error detection for post-editing neural machine translation
Gabriele Sarti
University of Groningen
Predictive uncertainty and other information extracted from MT models provide reasonable estimates of word-level translation quality. However, there is a lack of public studies investigating the impact of error detection methods on post-editing performance in real-world settings. We propose to conduct a user study with professional translators for two language directions sourced from our recent DivEMT dataset. The team aims to assess whether and how error span highlights can improve post-editing productivity while preserving translation quality. The research will focus on the influence of highlight quality by comparing (un)supervised techniques with best-case estimates using gold human edits, using productivity and enjoyability metrics for evaluation. The findings will be made available in an international publication alongside a public release of code and data. Such direction would be relevant to Translated to validate the applicability of error-detection techniques aimed at improving human-machine collaboration in translation workflows. The proposal is a reality check for research in interpretability and quality estimation, and will likely impact future research in these areas. Moreover, positive outcomes could drive innovation in post-editing practices for the industry. The project will be led by Gabriele Sarti and Arianna Bisazza, as part of his InDeep PhD project on user-centric interpretability for machine translation. The advisory team will include Ana Guerberof-Arenas, and Malvina Nissim, internationally recognized researchers in translation studies and natural language processing.
Machine Learning algorithms for machine translation
Open-sourcing a recent text to speech paper
Phillip Wang
Independent Researcher
Open-source implementations of scientific papers are one of the essential means by which progress in deep learning is achieved today. Corporate players have stopped open-sourcing recent text-to-speech (TTS) model architectures, often not even trained models. Instead, they tend to publish a scientific paper, sometimes with details in additional material, and an accompanying demo with pre-generated audio snippets. The proposal is to improve this situation by implementing a recent TTS paper such as Voicebox, open-sourcing our architecture. In addition, as much as possible, we would like to collect training data, train the model, and demonstrate that the open-sourced architecture performs well, for example by illustrating notable features or approximately reproducing some performance results (e.g., CMOS).
NEUROSCIENCE OF LANGUAGE
Tracking interhemispheric interactions and neural plasticity between frontal areas in the bilingual brain
Simone Battaglia
Alma Mater Studiorum – University of Bologna
Which is the human brain network that supports excellence in simultaneous spoken-language interpretation? Although there is still no clear answer to this question, recent research in neuroscience has suggested that the dorsolateral prefrontal cortex (dlPFC) is consistently involved in bilingual language use and cognitive control, including working memory (WM), which, in turn, is particularly important for simultaneous interpretation and translation. Importantly, preliminary evidence has shown that functional connectivity between prefrontal regions correlates with efficiently processing a second language. The present project proposal aims to characterize space-time features of interhemispheric interactions between left and right dlPFC in bilingual healthy adults divided into two groups of professional simultaneous interpreters and non-expert bilingual individuals. In these two groups, we will use cutting-edge neurophysiological methods for testing the dynamics of cortico-cortical connectivity, namely TMS-EEG co-registration, focusing on bilateral dlPFC connectivity. The procedure will make it possible to non-invasively stimulate the dlPFC and track signal propagation, to characterize the link between different aspects of language processing, executive functions, and bilateral dlPFC connectivity. The team of neuroscientists and linguists will provide novel insights into the neural mechanisms of interhemispheric communication in the bilingual brain and characterize the pattern of connectivity associated with proficient simultaneous interpretation.

HUMAN COMPUTER INTERACTION
How can MT and PE help literature cross borders and reach wider audiences: A Case Study
Vilelmini Sosoni
Ionian University
Researchers studied the usability and creativity of machine translation (MT) in literary texts focusing on translators’ perceptions and readers’ responses. But what do authors think? Is post-editing of MT output an answer to having more literature translated, especially from lesser-used languages into dominant languages? The study seeks to answer this question by focusing on the book Tango in Blue Nights (2024), a flash story collection about love written by Vassilis Manoussakis, a Greek author, researcher, and translator. The book is translated from Greek into English using Translated’s ModernMT system and is then post-edited by second-year Modern Greek students at Boston University who are native English speakers and have near-native proficiency in Greek. They follow detailed post-editing (PE) guidelines developed for literary texts by the researchers. The author analyzes the post-edited version and establishes whether it is fit for publication and how it can be improved. A stylometric analysis is conducted. The study is the first of its kind and wishes to showcase the importance of MT for the dissemination of literature written in lesser-used languages and provide a post-editing protocol for the translation of literary texts.
Neuroscience of Language
Realtime Multilingual Translation from Brain Dynamics
Weihao Xia
University College London
This project, Realtime Multilingual Translation from Brain Dynamics, is to convert brain waves into multiple natural languages. The goal is to develop a novel brain-computer interface capable of open-vocabulary electroencephalographic (EEG)-to-multilingual translation, facilitating seamless communication. The idea is to align EEG waves with pre-aligned embedding vectors from Multilingual Large Language Models (LLMs). The multi-languages are aligned in the vector space, allowing us to train the model with only a text corpus in one language. EEG signals are real-time and non-invasive but exhibit significant individual variances. The challenges lie in the EEG–language alignment and across-user generalization. The learned brain representations are then decoded into the desired language using LLMs such as BLOOM that produces coherent text almost indistinguishable from text written by humans. Currently, the primary application targets individuals who are unable to speak or type. However, in the future, as brain signals increasingly serve as the control factor for electrical devices, the potential applications will expand to encompass a broader range of scenarios.
Machine learning algorithms for translation
Language Models Are More Than Classifiers: Rethinking Interpretability in the Presence of Intrinsic Uncertainty
Julius Cheng
University of Cambridge
Language translation is an intrinsically ambiguous task, where one sentence has many possible translations. This fact, combined with the practice of training neural language models (LMs) with large bitext corpora, leads to the well-documented phenomenon that these models allocate probability mass to many semantically similar yet lexically diverse sentences. Consequently, decoding objectives like minimum Bayes risk (MBR), which aggregate information across the entire output distribution, produce higher-quality outputs than beam search. Research on interpretability and explainability for natural language generation (NLG) has to date almost exclusively focused on generating explanations for a single prediction, yet LMs have many plausible high probability predictions. Julius’s team proposes to adapt interpretability to this context by asking the question, “do similar predictions have similar explanations?” They will answer this by comparing explanations generated by interpretability methods such as attention-based interpretability, layerwise relevance propagation, and gradient-based attribution across predictions. The goal of this project is to advance research in interpretability for NLG, deepen our understanding of the generalization capabilities of LMs, and develop new methods for MBR decoding.
LANGUAGE DATA
Curvature-based Machine Translation Dataset Curation
Michalis Korakakis
University of Cambridge
Despite recent advances in neural machine translation, data quality continues to play a crucial role in model performance, robustness, and fairness. However, current approaches to curating machine translation datasets rely on domain-specific heuristics, and assume that datasets contain only one specific type of problematic instances, such as noise. Consequently, these methods fail to systematically analyze how various types of training instances—such as noisy, atypical, and underrepresented instances—affect model behavior. To address this, Michalis’s team proposes to introduce a data curation method that identifies different types of training instances within a dataset by examining the curvature of the loss landscape around an instance—i.e., the magnitude of the eigenvalues of the Hessian of the loss with respect to that instance. Unlike previous approaches, the proposed method offers a comprehensive framework that provides insights into machine translation datasets independent of model architecture and weight initialisation. It is also applicable to any language pair and monolingual translation tasks such as text summarisation.
Language economicsLanguage economics
Development of a Multilingual Machine Translator for Philippine Languages
Curvature-based Machine Translation Dataset Curation
Charibeth Cheng
De La Salle University
The Philippines is an archipelagic country consisting of more than 7,000 islands, and this has contributed to its vast linguistic diversity. The Philippines is home to 175 living, indigenous languages, with Filipino designated as the national language. Within formal education, 28 indigenous languages serve as mediums of instruction, alongside English, which holds official status in business, government, and academia. The Philippines’ diverse linguistic landscape underscores the need for effective communication bridges. The project aims to develop a multilingual machine translation system for at least seven Philippine languages, aligning with efforts to standardize and preserve indigenous languages. Multilingual machine-translation systems serve as vital bridges between speakers of different languages, fostering cultural inclusivity and bolstering educational and socioeconomic progress nationwide. This project aims to develop a multilingual machine translation system capable of translating text across at least seven Philippine languages.
Specifically, this project will focus on the following:
1. Collect and curate linguistic data sets in collaboration with linguistic experts and native speakers to ensure the accuracy and reliability of the translation system.
2. Implement machine-learning algorithms and natural language-processing techniques to train the translation model, considering the low-resource nature of Philippine languages.
3. Evaluate the efficacy of the translation system developed using standardized metrics and human evaluation.
The 2025 call is open. Submit your project.
Imminent was founded to help innovators who share the goal of making it easier for everyone living in our multilingual world to understand and be understood by everyone else. Imminent builds a bridge between the world of research and
the corporate world by supporting research through scientific publications, interviews, and annual grants, funding ground- breaking projects in the language industry.
With the Imminent Research Grants project, each year, Immi- nent allocates $100,000 to fund five original research projects with grants of $20,000 each to explore the most advanced frontiers in the world of language services. Imminent expects the call to appeal to startuppers, researchers, innovators, authors, university labs, organizations and companies.
The 2025 call is open.