Each year, our research center Imminent awards €100,000 research grants to promote the most innovative research projects in the linguistic field. Below you can find the presentation of the projects awarded.
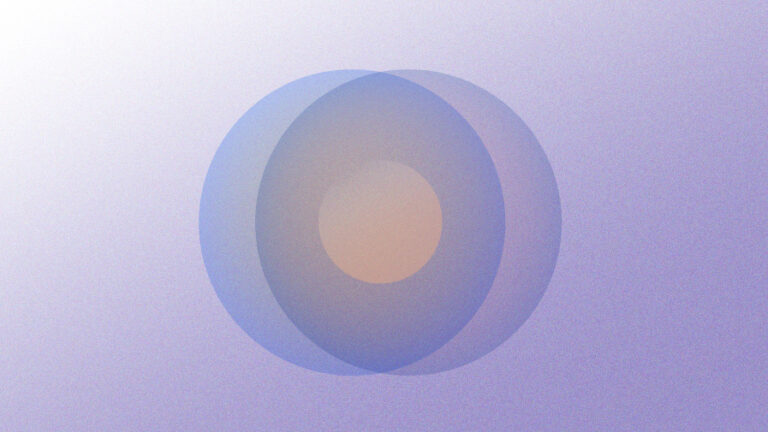
Imminent Research Grants
€100,000 to fund language technology innovators
Imminent was founded to help innovators who share the goal of making it easier for everyone living in our multilingual world to understand and be understood by all others. Each year, Imminent allocate €100,000 to fund five original research projects to explore the most advanced frontiers in the world of language services. Topics: Language economics – Linguistic data – Machine learning algorithms for translation – Human-computer interaction – The neuroscience of language.
Apply nowResearch area – Linguistic Data
Curvature-based Machine Translation Dataset Curation
Michalis Korakakis University of Cambridge
Despite recent advances in neural machine translation, data quality continues to play a crucial role in model performance, robustness, and fairness. However, current approaches to curating machine translation datasets rely on domain-specific heuristics, and assume that datasets contain only one specific type of problematic instances, such as noise. Consequently, these methods fail to systematically analyze how various types of training instances—such as noisy, atypical, and underrepresented instances—affect model behavior.
To address this Michalis’s team proposes to introduce a data curation method that identifies different types of training instances within a dataset by examining the curvature of the loss landscape around an instance—i.e., the magnitude of the eigenvalues of the Hessian of the loss with respect to that instance. Unlike previous approaches, the proposed method offers a comprehensive framework that provides insights into machine translation datasets independent of model architecture and weight initialization. Additionally, it is applicable to any language pair and monolingual translation tasks such as text summarization.
Research area – Language economics
Development of a Multilingual Machine Translator for Philippine Languages
Charibeth Cheng De La Salle University
The Philippines is an archipelagic country consisting of more than 7000 thousand islands, and this has contributed to its vast linguistic diversity. Our country is home to 175 living, indigenous languages, with Filipino designated as the national language. Within formal education, 28 indigenous languages serve as mediums of instruction, alongside English, which holds official status in business, government, and academia.
The Philippines’ diverse linguistic landscape underscores the need for effective communication bridges. Our project aims to develop a multilingual machine translation system for at least 7 Philippine languages, aligning with efforts to standardize and preserve indigenous languages. Multilingual machine translation systems serve as vital bridges between speakers of different languages, fostering cultural inclusivity and bolstering educational and socioeconomic progress nationwide.
This project aims to develop a multilingual machine translation system capable of translating text across at least 7 Philippine languages.
Specifically, this project will focus on the following:
1. Collect and curate linguistic data sets in collaboration with linguistic experts and naive speakers to ensure the accuracy and reliability of the translation system.
2. Implement machine learning algorithms and natural language processing techniques to train the translation model, considering the low-resource nature of Philippine languages.
3. Evaluate the efficacy of the developed translation system using standardized metrics and human evaluation.
Research area – Neuroscience of Language
Realtime Multilingual Translation from Brain Dynamics
Weihao Xia University College London
This project, Realtime Multilingual Translation from Brain Dynamics, is to convert brain waves into multiple natural languages. The goal is to develop a novel brain-computer interface capable of open-vocabulary electroencephalographic (EEG)-to-multilingual translation, facilitating seamless communication. The idea is to align EEG waves with pre-aligned embedding vectors from Multilingual Large Language Models (LLMs). The multi-languages are aligned in the vector space, allowing us to train the model with only a text corpus in one language. EEG signals are real-time and non-invasive but exhibit significant individual variances. The challenges lie in the EEG-language alignment and across-user generalization. The learned brain representations are then decoded into the desired language using LLMs such as BLOOM that produces coherent text that is almost indistinguishable from text written by humans.
Currently, the primary application targets individuals who are unable to speak or type. However, in the future, as brain signals increasingly serve as the control factor for electrical devices, the potential applications will expand to encompass a broader range of scenarios.
Research area – Human-Computer Interaction
How can MT and PE help literature cross borders and reach wider audiences: A Case Study
Vilelmini Sosoni Ionian University
Researchers studied the usability and creativity of machine translation (MT) in literary texts focusing on translators’ perceptions and readers’ response. But what do authors think? Is post-editing of MT output an answer to having more literature translated especially from lesser-used languages into dominant languages? The study seeks to answer this question by focusing on the book Tango in Blue Nights (2024), a flash story collection about love written by Vassilis Manoussakis, a Greek author, researcher and translator. The book is translated from Greek into English using Translated’s ModernMT system and is then post-edited by 2nd year Modern Greek students at Boston University who are native English speakers and have near native capacity in Greek. They follow detailed PE guidelines developed for literary texts by the researchers.
The author analyses the post-edited version and establishes whether it is fit for publication and how it can be improved. A stylometric analysis is conducted. The study is the first of its kind and wishes to showcase the importance of MT for the dissemination of literature written in lesser-used languages and provide a post-editing protocol for the translation of literary texts.
Research area – Machine learning algorithms for translation
Language Models Are More Than Classifiers: Rethinking Interpretability in the Presence of Intrinsic Uncertainty
Julius Cheng Univesity of Cambridge
Language translation is an intrinsically ambiguous task, where one sentence has many possible translations. This fact, combined with the practice of training neural language models (LMs) with large bitext corpora, leads to the well-documented phenomenon that these models allocate probability mass to many semantically similar yet lexically diverse sentences. Consequently, decoding objectives like minimum Bayes risk (MBR), which aggregate information across the entire output distribution, produce higher quality outputs than beam search.
Research on interpretability and explainability for natural language generation (NLG) has thus far almost exclusively focused on generating explanations for a single prediction, yet LMs have many plausible high probability predictions. Julius’s team proposes to to adapt interpretability to this context by asking the question, “do similar predictions have similar explanations?” they will answer this by comparing explanations generated by interpretability methods such as attention-based interpretability, layerwise relevance propagation, and gradient-based attribution across predictions.
The goal of this project is to advance research in interpretability for NLG, deepen our understanding of the generalization capabilities of LMs, as well as develop new methods for MBR decoding.