Research
2023 Imminent Research Grants Winning project – Human Computer Interaction Category
Introduction
Machine Translation (MT) has become an integral part of modern translation workflows, and post-editing (PE) of MT outputs is a common practice in the language services industry. Translators often work with Computer-Aided Translation (CAT) tools that incorporate MT suggestions, improving productivity while maintaining high-quality standards.
In this context, quality estimation (QE) methods have been proposed to further direct the translators’ focus to machine-translated outputs needing revision. QE techniques are mainly applied to text paragraphs or sentences to obtain a score showing the overall translation quality of the MT output, similar to fuzzy matches in translation memories (TMs). Such coarse-grained QE methods are nowadays frequently employed in CAT workflows to determine whether an MT proposal should be presented to the translator or discarded. However, the potential of more fine-grained quality estimation techniques remains largely unexplored in professional settings.
To our knowledge, Unbabel’s Quality Intelligence API is the only industrial solution in this area.
Beyond segment-level assessment, word-level quality estimation presents a promising avenue for directing post-editors’ attention to specific problematic regions within a sentence. For example, consider the following machine-translated sentence:
“The company announced its new products line, which will be available in stores from start of next month.”
A word-level QE system might detect “products” and “from start of” as ungrammatical, prompting the post-editor to verify these specific phrases and correct potential errors.
While this task is vastly more challenging from a machine learning perspective due to its fine-grained nature, it could substantially benefit the post-editing process. Despite the potential advantages, little research has been done to assess the practical usefulness of word-level QE for professional translators. This project aims to bridge that gap by investigating how translation error predictions can be effectively presented in a translation interface and how their quality influences post-editors productivity and experience.
Exploiting the Internals of MT Models for Error Prediction
Word-level QE annotations are typically produced by models trained on large amounts of annotated data to mimic the choices of professional quality annotators, an approach commonly referred to as supervised word-level QE. While effective, this method is limited by the availability of high-quality training data and may not generalize well across different domains or language pairs. On the other hand, unsupervised word-level QE methods leverage internal information produced by the neural machine translation (NMT) model used to generate the outputs. Traditionally, much of this internal information is ignored during the translation generation process. However, recent studies have shown that so-called ‘model internals’ can be highly useful in identifying translation issues such as additions, omissions and hallucinations without additional training data or external QE models (Guerreiro et al., 2022, Dale et al., 2023) .
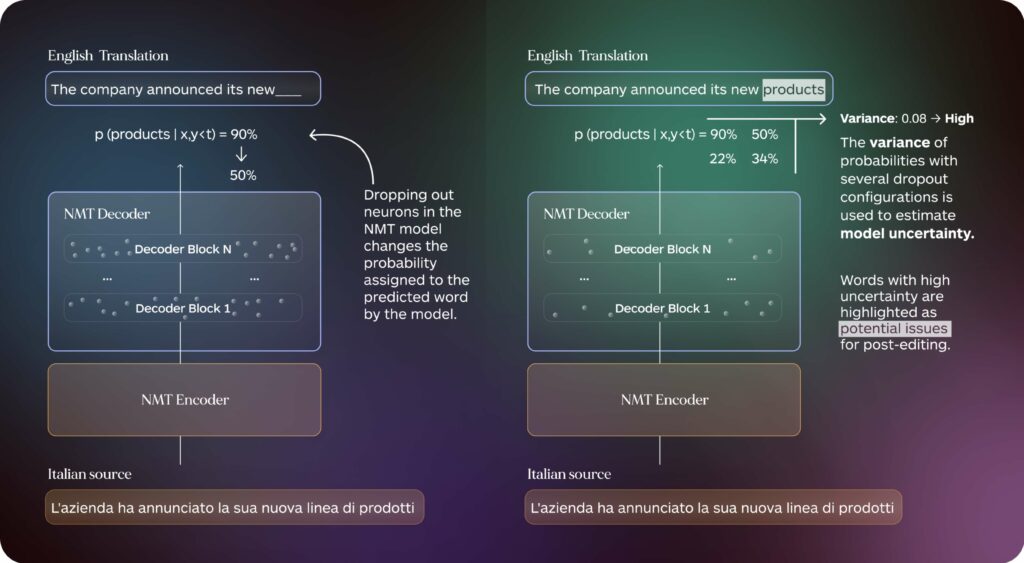
Figure 1: Overview of the process for creating unsupervised word-level QE highlights we used in our study.
This project aims to compare the accuracy of supervised and unsupervised approaches in detecting potential issues in MT outputs and to assess their downstream impact on post-editing productivity and enjoyability. More broadly, our research aims to improve the efficiency and satisfaction of human-machine collaboration in translation workflows.
Study Design
Setup
Our study, nicknamed “Quality Estimation for Post-Editing” (QE4PE), involved 24 professional translators, 12 per translation direction, post-editing a collection of 50 English documents machine-translated into Italian and Dutch. Texts were translated using NLLB 3.3B, a strong open-source multilingual machine translation system by Meta AI. The documents employed for our evaluation were selected from a mix of biomedical research abstracts and social media posts collected for the Workshop on Machine Translation’s (WMT) 2023 evaluation campaign.
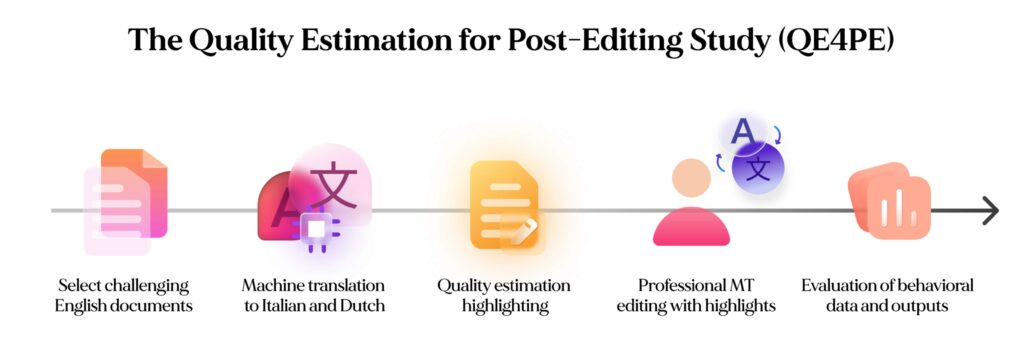
Figure 2: A schematic overview of the QE4PE study.
To evaluate the effectiveness of word-level QE, we developed a simple online interface that supports the editing of highlighted texts. This interface allowed us to present machine-translated content to the participants with QE-based highlights at two degrees of severity (minor and major), enabling us to track and analyze their interactions, editing patterns, and overall performance across different conditions.
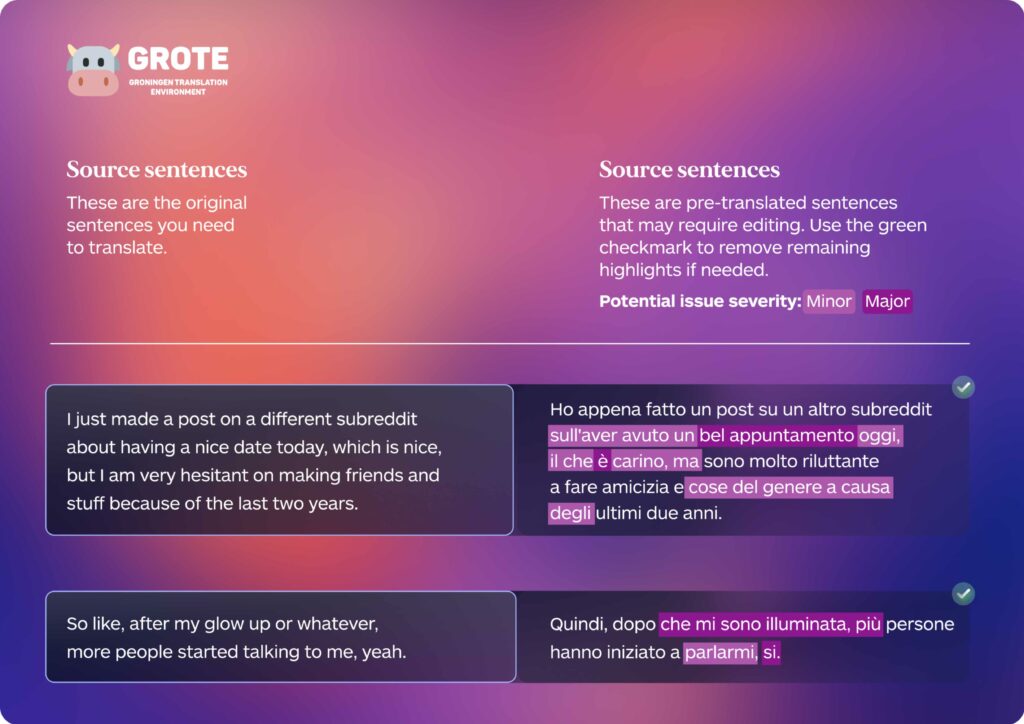
Figure 3: Example of the GroTE web interface used for this study, showing two machine-translated passages with highlighted spans marking potential issues.
Editing Modalities
After a pre-task aimed at familiarizing translators with the interface, the QE highlights and the type of data, we conducted the main task in four different settings to assess the downstream impact of quality highlights:
- No Highlights: The MT outputs requiring post-editing are presented without any highlighting. This setting acts as a baseline.
- Oracle Highlights: Highlights are added over spans in the MT output that were post-edited by at least 2 out of 3 professional translators in a previous editing stage. This can be regarded as a best-case scenario for systems trained on human edits.
- Supervised Highlights: Highlights are produced by XCOMET-XXL, the current state-of-the-artsystem trained for word-level QE.
- Unsupervised Highlights: Highlights are produced by selecting the spans for which the NLLB MT model showed higher uncertainty according to Monte Carlo Dropout probability variance, a popular technique to estimate uncertainty using model internals.
By comparing the results across these settings, we aim to quantify the impact of different QE approaches on post-editing productivity, the quality of highlights and final translations, and the translators’ subjective experience. This comprehensive evaluation will provide valuable insights into the practical benefits of integrating word-level QE into professional translation workflows.
Preliminary Results
While we are still in the process of completing our analyses on the collected data, here we present some initial findings based on the assessment of post-editing productivity, quality, and enjoyability across the four study conditions.
Productivity: Do Highlights Make Post-editors Faster?
We measured the average time translators spent editing machine-translated documents across the four settings to evaluate the impact of different QE highlights on post-editing productivity.
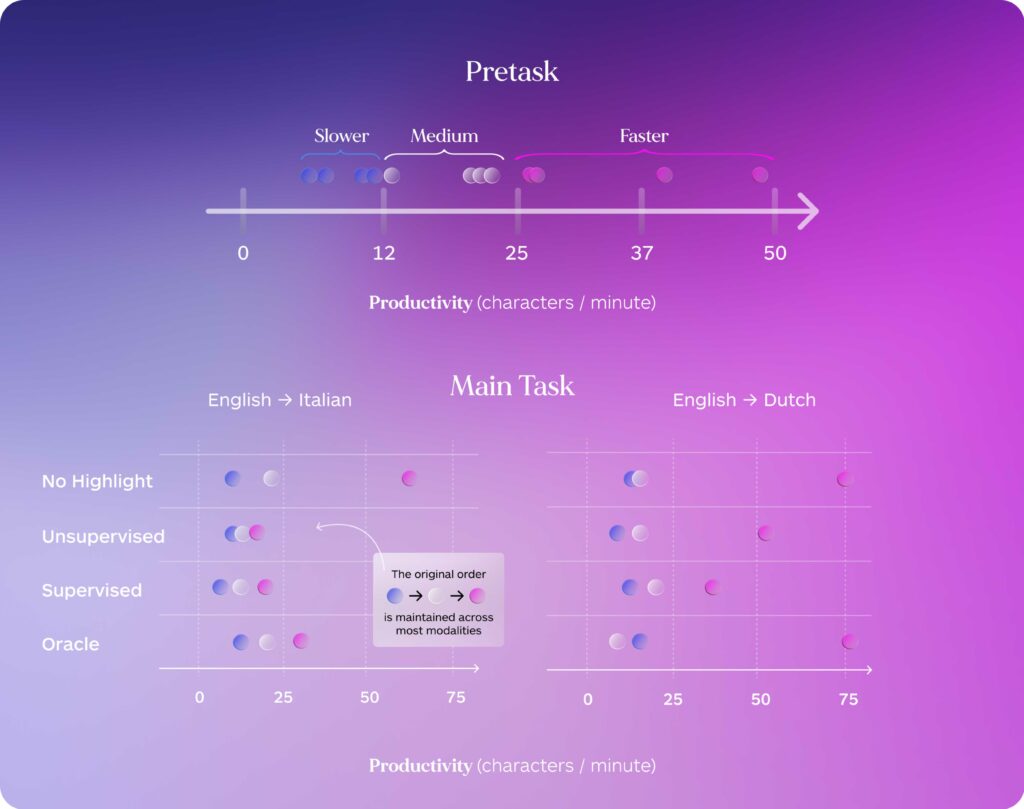
Figure 4: Top: Translators’ editing speed is initially assessed on a pretask, and each post-editor (represented by a colored dot) is assigned to an editing modalities according to their speed to maintain balanced groups. Bottom: Productivity across editing modalities for the two tested translation directions. Translators tend to maintain their productivity ordering (Slower → Medium → Faster) across all editing modalities, suggesting the effect of individual speed remains predominant, regardless of editing modality.
Our preliminary results show that highlight modality is less predictive of post-editing productivity compared to the individual editing speed of each translator. Figure 4 illustrates how translators that are found to be faster on a smaller subset of translations edited before the main task (Pretask) remain faster than their colleagues regardless of the editing modality. This highlights the need to account for individual editing speed differences when evaluating the productivity impact of word-level quality estimation.
Quality: Do Highlights Help Translators Detect Errors?
While a manual assessment of translation quality is currently ongoing, as a preliminary step we use the referenceless XCOMET metric as a proxy to evaluate the accuracy and fluency of the post-edited translations.
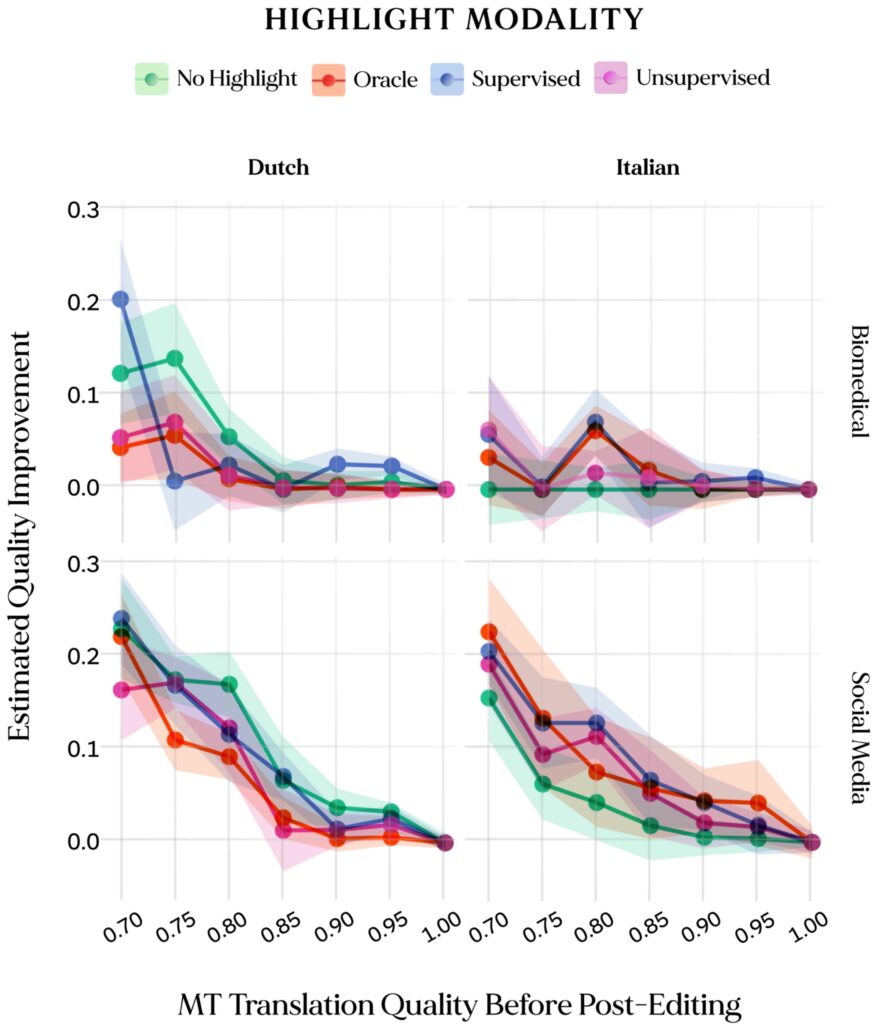
Figure 5: Translation quality improvements for post-edited texts starting from MT outputs of variable quality (x axis). Initial MT quality and post-editing improvements were estimated with XCOMET-XXL across two target languages (columns) and domains (rows). Scores are the median across all post-editors per modality (n=3 for each target language and modality combination).
Our preliminary investigation in the effect of editing modalities on output quality shows that overall quality of post-edited outputs across all highlight modalities remains in line with regular post editing without highlights, leading to improved quality only in some settings (e.g. in the Social Media and Biomedical domains for the English -> Italian translation direction).
From Figure 5, we also observe that, according to our proxy metric, quality gains from post-editing are consistently positive across all settings. We also note that gains for lower-quality MT outputs are especially prominent in the Social Media domain, with diminishing gains for higher-quality translations across all settings.
This suggests that gains stemming from the use of QE highlights might not be observed at a macroscopic level, but might become relevant for tricky cases with less evident errors.To validate this hypothesis, we evaluate a small set of examples containing manually-crafted critical errors that were inserted in some of the MT outputs, and were frequently highlighted by several word-level QE techniques. On this subset, we find that translators having access to highlights were 16-25% more likely to correct the critical errorsthan translators editing without highlights, suggesting that highlights can help attract the editor’s attention to problematic elements in the translation.
Enjoyability: Do Translators Like to Work with Word-Level Highlights?
We administered a post-task questionnaire to assess translators’ subjective experience, including their satisfaction with the various highlight types and overall ease of the post-editing process.
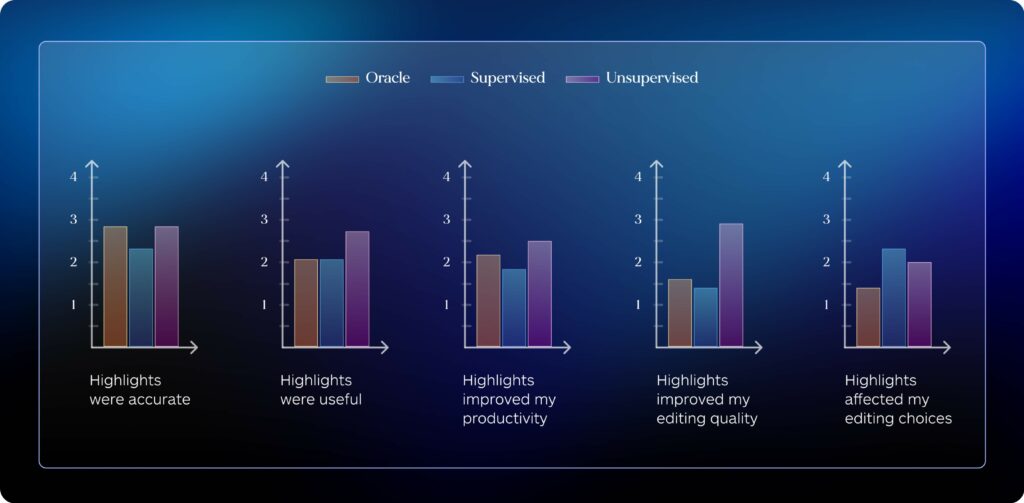
Figure 6: Ratings per editing modality for statements about highlights collected in the post-task questionnaire. Scores are averaged across all post-editors in each modality across both translation directions (n = 6 per modality), ranging between 1 (Strongly disagree) and 5 (Strongly agree).
While some translators found the presence of highlights useful (e.g. one translator stated that highlights “[…] helped me a lot, making the editing process faster and somehow easier.”), the general sentiment was that highlights were “[…] too confusing […]” and “[…] more of an eye distraction […]”, noting that generally they were “[…] not quite accurate enough to rely on them as a suggestion”. Importantly, these comments were found prevalent across all editing modalities, including editing with highlights based on previous human post-edits, despite the quality results highlighted in the previous section. This suggests that even error highlights obtained from several human editors might not fully capture problematic elements or more general mistakes (as one translator puts it, “[…] sometimes it happened that you had to edit not just the highlighted word but the entire sentence”), despite the high quality of initial translations.
The median XCOMET-XXL QE score of MT outputs across both translation directions was 0.952, with a standard deviation of 0.11
Interestingly, unsupervised highlights produced from MT model internals were generally rated more useful than their counterparts, including those derived from previous human post-edits (Human Edits in Figure 6). This suggests that the difference in quality between various word-level QE approaches might not be immediately evident, and improved usability might outweigh accuracy gains in the eyes of post-editors.
Conclusion
This concludes our introduction to the QE4PE and our preliminary findings regarding the impact of word-level highlights on productivity, quality and enjoyability for MT post-editing. Our preliminary findings paint a nuanced picture, showing the potential of word-level QE in improving the quality of post-edited outputs while also underscoring the need for further usability improvements to make this technique viable in professional translation workflows. Importantly, the findings of our study pertain to a specific translation direction for which high-quality MT is available, and our evaluation focused specifically on professional post-editors with extensive experience, which might benefit less from the guidance provided by word-level QE methods. In light of this, all contents of this study, including our online interface and the collected post-editing logs, will be made available to the research community to enable future assessments for different translation direction, with alternative editor profiles (e.g. professionals, translation students, L2 learners) and new QE techniques.
Impact
The project’s goal is two-fold, addressing both practical application and technical innovation in word-level quality estimation for machine translation. Our user study described in this article represents a first step for assessing the real-world impact of word-level QE in post-editing workflows and providing valuable insights into how translators interact with and benefit from fine-grained quality estimation cues, potentially reshaping best practices in the industry. As a next step, we will experiment with novel unsupervised techniques for word-level QE exploiting the internal information of neural machine translation models. We aim to create more faithful and explainable quality estimation methods that can be readily applied to new languages and domains without extensive retraining.
Team Members
Gabriele Sarti
PhD Student, University of Groningen
Gabriele’s doctoral project as a member of the InDeep Dutch consortium focuses on devising new approaches and applications of explainable AI in neural machine translation. His research is particularly concerned with bridging the gap between theoretical insights about the inner workings of neural network-based language models to improve human-AI collaboration. He was previously a research intern on the Amazon Translate team and worked as a research scientist at the Italian startup Aindo.
Arianna Bisazza
Associate Professor, University of Groningen
Arianna’s research aims to identify the intrinsic limitations of current language modeling paradigms and improve machine translation quality for challenging language pairs. She has been working towards better MT algorithms for fourteen years and was recently awarded sizeable grants for her research on language models interpretability and the development of conversational and cognitively-plausible learning systems by the Dutch Research Council.
Vilém Zouhar
PhD Student, ETH Zürich
Vilém researches non-mainstream machine translation, evaluation, quality estimation, and human-computer interaction. In recent studies with collaborators from the WMT community, he explored the pitfalls of automatic evaluation and their solution. His PhD project at ETH Zürich is about how to make human and automatic evaluation of machine translation and NLP in general more robust, higher-quality and economical.
Malvina Nissim
Full Professor, University of Groningen
Malvina is the chair of Computational Linguistics and Society at the University of Groningen, The Netherlands. Her research interests span several aspects of automatic text analysis and generation, with a recent focus on writing style and reformulation. She is the author of 100+ publications in international venues, regularly reviews for major conferences and journals, organizes and chairs large-scale scientific events, and is a member of the ACL Ethics Committee.
Ana Guerberof Arenas
Ana Guerberof Arenas
Associate Professor, University of Groningen
Ana was recently awarded an ERC Consolidator grant for the INCREC project, aiming to explore the creative process in literary and audio-visual translation and its intersection with technology. With more than 23 years of experience in the translation industry, she has authored several articles and chapters on MT post-editing, translator training, and ethical considerations in MT and AI.
Grzegorz Chrupała
Associate Professor, University of Tilburg
Grzegorz is interested in computation in biological and artificial systems and their connections. His research focuses primarily on computational models of learning (spoken) language in naturalistic multimodal settings and the analysis of representations emerging in deep learning architectures. He regularly serves as Senior Area Chair for major NLP and AI conferences such as ACL and EMNLP. He was one of the creators of the popular BlackboxNLP Workshop on Analyzing and Interpreting Neural Networks for NLP.
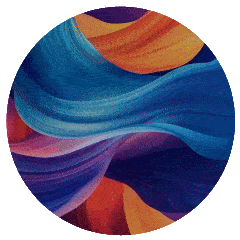
Imminent Science Spotlight
Academic lectures for language enthusiasts
Imminent Science Spotlight is a new section where the next wave of language, technology, and Imminent discoveries awaits you. Curated monthly by our team of forward-thinking researchers, this is your go-to space for the latest in academic insight on transformative topics like large language models (LLM), machine translation (MT), text-to-speech, and more. Every article is a deep dive into ideas on the brink of change—handpicked for those who crave what’s next, now.
Discover more here!