Technology
These are really hard times for the linguistic signs. Should we work to save linguistic signs from the threats of modern AI? An exclusive article penned by Guido Vetere, Adjunct Professor of Artificial Intelligence at the Faculty of Applied Sciences and Technologies of the University of Guglielmo Marconi and leader of IBM’s Center for Advanced Studies.
These are hard times for linguistic signs. They are being hunted by increasingly powerful and aggressive computers. That intimate (albeit problematic) union of expressions, concepts, and realities that is at the core of our linguistic thinking is very uncomfortable for machines. When processed by algorithms, expressions—as the manifest part of the sign—are thus detached from their hidden (and problematic) conceptual part and instead treated as pure numbers. Units of meaning are disregarded and lexical semantics is considered a nuisance, the legacy of a dusty past. Some people are even thinking of getting rid of language altogether by connecting the brain directly to computers.
“When processed by algorithms, expressions—as the manifest part of the sign—are thus detached from their hidden (and problematic) conceptual part and instead treated as pure numbers.”
A vision of words as pure combinatorial elements is taking hold in computer science. When fed into machines, what people mean by their words is becoming quite irrelevant: what really matters to algorithms is how words unfold into plausible arrays. Artificial intelligence learns the laws of this unfolding by looking at millions of sentences taken from petabytes of available digitized text, using a statistical approach.
By learning how words combine in millions of sentences, computers can make a fairly accurate prediction of the next words I am going to write right now. They can also successfully evaluate how similar two sentences are, or whether a sentence may contain an answer to a question, and what that answer is likely to be. In particular, computers are increasingly able to translate from one language to another, provided that sufficiently large bilingual corpora are available.
By accomplishing these tasks using a mathematical basis, today’s computers dismiss linguistic signs altogether. After all, if they can succeed in sophisticated language tasks, why should they care about meanings, something that even linguists and philosophers struggle to grasp? What’s more, why should we blame computers for doing their job (i.e. computing) so well when they approach human language?
Incidentally, a sort of statistical account of meaning also exists in linguistics. It is rooted in the so-called “distributional hypothesis”, which was quite popular in the middle of the last century. “You shall know a word by the company it keeps,” as linguists like Firth or Harris used to say. In other words, the more semantically similar two words are, the more they tend to occur in similar sentences.
For instance, suppose a Martian linguist came to Earth and read sentences such as the following: “I went to school by bike”, “I went to the office by car”, “I went to the restaurant by taxi”. He or she (or whatever) could speculate that school, office, and restaurant (on the one hand) and bike, car, and taxi (on the other) are semantically similar. This would be a kind of statistical inference based on data—exactly what computers are good at today. Giant neural networks trained on huge textual corpora employed by major technology providers rely on this hypothesis, as do smaller networks available at scale for anyone to use. And they succeed in many tasks.
But is this a reason to say that we can get rid of any explicit representation of meaning when programming computers to understand human language? Some people, especially philosophers, say that this is not possible in principle: statistics cannot be promoted to semantics. But many, especially practitioners, say that the more we add data and computation, the more we approximate the effects of language understanding. In their view, if this approximation is good enough, we do not need anything else. In particular, we do not need any “theory of meaning”, whatever that is. As neural models grow and improve, this vision is gaining momentum.
The essence of the question, therefore, is whether the best possible statistical approximation of linguistic behaviors, based on the largest amount of textual data, would come so close to human understanding that any other representation would be useless, if not misleading.
“The essence of the question, therefore, is whether the best possible statistical approximation of linguistic behaviors, based on the largest amount of textual data, would come so close to human understanding that any other representation would be useless, if not misleading.”
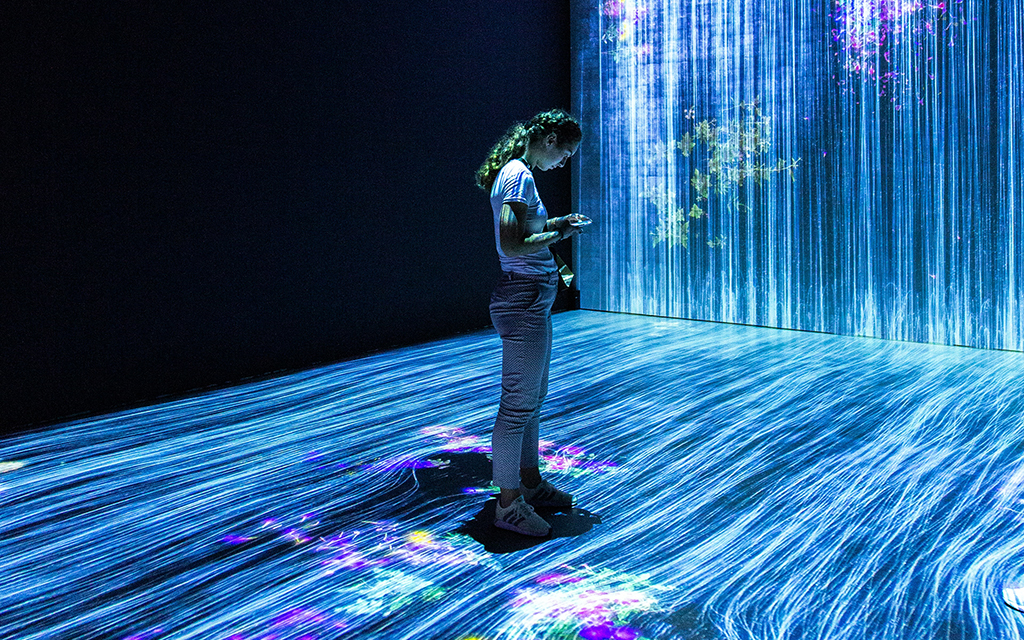
We know that human understanding, whatever it is, has little to do with statistics. There is no statistical likelihood demonstrating that, at dinner, we understand a phrase like “pass me the salt, please” as a request to bring the salt shaker to the requester. If we are aware of the situation, including the objects we have on hand, the people who are sitting with us, and what they should be doing in that context, the sentence will simply trigger the appropriate response on our part, without any uncertainty.
Language learning does not seem in any way statistical either. Children do not learn that cats are in the same class as dogs because they often happen to hear the word “cat” in the same kind of phrases where “dog” occurs. Instead, in real-world situations, they associate words with entities— even fictional or non-present ones—ask their parents many metalinguistic questions, and thus, over the years, build their knowledge of the language, the world (imagery included), and their interplay.
People who work in artificial intelligence know this story. Their point, however, is very strong: since representing linguistic knowledge is theoretically difficult and practically cumbersome, the best we can do with the means we have is to substitute that knowledge by exploiting data and calculations. This is almost unquestionable. However, I do not think that this can justify what I would call “quantitative illusion”, namely the idea that, given enough data and with more computational resources, a statistical surrogate could produce an equivalent of human language proficiency.
Judea Pearl received a Turing Award for his studies on causal models. He provided formal proof that statistical correlation cannot climb what he calls the “ladder of causality”. Put simply, a causal model is always a speculative human construction that cannot be derived from data, if only because, as statisticians know, “correlation is not causation”. In my opinion, these studies can be easily applied to language modeling and show that the computation of textual correlations cannot produce an equivalent of human linguistic knowledge.
The reason for this limitation is the systemic nature of linguistic signs, where, as Saussure said, “tout se tient” (everything is tied together). This nature is indeed causal, rather than correlative. The system of signs, in fact, is an integral part of the belief system on which social and personal life is based. Understanding language is the process of building interpretive hypotheses of what is being said, as we listen or read, given context and background. Beside linguistic expressions, this process of construction involves practical aspects, purposes, interests, feelings, emotions, and a lot of knowledge about personal and collective history, as well as the way the world is made (also known as “common sense”). On the other hand, the linguistic system itself is shaped as part of processes involving all the sensory, emotional, cultural, social, and cognitive dimensions of human beings.
“The reason for this limitation is the systemic nature of linguistic signs, where, as Saussure said, “tout se tient” (everything is tied together). This nature is indeed causal, rather than correlative. The system of signs, in fact, is an integral part of the belief system on which social and personal life is based.”
When we write, we encode linguistic signs on paper, a computer, or other media, but they are limited to their signifiers, i.e. their manifest part. However, the point where words hook onto their meaning is on the hidden side, a region in which subjective memories, non-linguistic knowledge, and even unconscious life play a crucial role. The reconstruction of the hidden part of the sign relies on the abductive reasoning (i.e. reasoning according to the best explanation) of the reader. Umberto Eco vividly elaborated on this in his Lector in fabula (1979). Texts are arrays of clues that mean nothing without the reader’s interpretation, just as our footprints in the sand mean nothing to crabs walking along the beach.
A computer reading a text is much like one of these crabs: it cannot figure out why the words are there; it just knows how their sequences are shaped. In fact, there are countless examples of the semantic failures of AI linguistic systems, even the most powerful ones, due to a lack of causal reasoning and “common sense” knowledge. However, sequence-based reasoning often does its job.
In general, one can say that today’s linguistic automata stop at the edge of interpretation. Within these limits, however, there are plenty of useful tasks to be carried out—all the better if we are aware of the limits. The controversial point is whether it is possible for them to overcome these limits with the brute force of data and calculation. I hope I have provided some suggestions why this possibility should be doubted. I do not believe that the linguistic sign is expendable in the AI of the future. On the contrary, as the founding element of causal cognitive models, it is the key ingredient of the abductive reasoning that underlies every interpretation, i.e. every understanding, human or otherwise.
If this stance makes sense, we should work to save linguistic signs from the threats of modern AI. In fact, many people are actively engaged in this rescue: the book “Rebooting AI” (2019) by Gary Marcus and Ernest Davis is all about this issue. This rescue is not only and not primarily a technical problem. On the contrary, it is about giving human beings back the place they deserve.
Photo credit: Katarzyna Pe and Mahdis Mousavi, Unsplash