Technology
The most comprehensive backup data to document the unrelenting progress we are seeing with adaptive MT technology in production use in professional translation settings.
Why progress in MT (machine translation) is a good proxy of progress with the technological singularity
For as long as machine technology has been around (now over 70 years) there have been regular claims made by developers of the technology reaching “human equivalence”. However, until today we have not had a claim that has satisfied practitioners in the professional translation industry, who are arguably the most knowledgeable critics around. For these users, the actual experience with MT has not been matched by the many extravagant claims made by MT developers over the years. This changes with the long-term study and translation production data presented by Translated at the AMTA conference which provides the missing elements: a huge industrial scale evidentiary sample validated by a large group of professional translators across multiple languages based on professional translation work done in real-world production scenarios.
The historical difficulty in providing acceptable proof does not mean that progress is not being made, but it is helpful to place these claims in proper context and perspective to better understand what the implications are for the professional and enterprise use of MT technology. The history of MT is unfortunately filled with empty promises.
The historical difficulty in providing acceptable proof does not mean that progress is not being made, but it is helpful to place these claims in proper context and perspective to better understand what the implications are for the professional and enterprise use of MT technology. The history of MT (machine translation) is unfortunately filled with empty promises. HMT (human language translation) is considered among the most difficult theoretical problems in AI, and thus we should not be surprised that it is a challenge that has not yielded completely to the continuing research efforts of MT technology experts over the decades.
HMT (human language translation) is considered among the most difficult theoretical problems in AI, and thus we should not be surprised that it is a challenge that has not yielded completely to the continuing research efforts of MT technology experts over the decades. Also, many experts have said that MT is a difficult enough challenge (AI-complete: because it requires a deep contextual understanding of the data, and the ability to make accurate predictions based on that data) that it is a good proxy for AGI (Artificial general intelligence is the ability of a machine process/ agent to understand or learn any intellectual task that a human being can) and thus progress with MT can also mean that we are that much closer to reaching AGI.
The Historical Lack of Compelling Evidence
MT researchers are forced to draw conclusions on research progress being made based on relatively small samples of non-representative data (from the professional translation industry perspective) that are evaluated by low-cost human “translators”. The Google Translate claims in 2016 are an example of a major technology developer making “human equivalence” claims based on limited data that was possible within the scope of the technology development process typical at the time. Namely, here are 200 sentences that amateur translators say are as good as human translation, thus we claim we have reached human equivalence with our MT.
Thus, while Google did indeed make substantial progress with its MT technology, the evidence it provided to make the extravagant claim lacked professional validation, was limited only to a small set of news domain sentence samples and was not representative of the diverse and broad scope of typical professional translation work which tends to be much more demanding and varied.
The problem from the perspective of the professional industry with these historical as-good-as-humans claims can be summarized as follows:
- Very small samples of non-representative data: Human equivalence is claimed on the basis of evaluations of a few news domain segments where non-professional translators were unable to discern meaningful differences between MT and human translations. The samples used to draw these conclusions were typically based on no more than a few hundred sentences.
- Automated quality metrics like BLEU were used to make performance claims: The small samples of human evaluation were generally supported by larger (a thousand or so) sentences where the quality was assessed by an automatic reference-based score. There are many problems with these automated quality scores as described here, and we now know that they miss much of the nuance and variation that is typical in human language, resulting in erroneous conclusions, and at best they are very rough approximations of competent human assessments. COMET and other metrics are slightly better quality approximation scores but still fall short of competent human assessments which are still the “gold standard” in assessing translation output quality. The assessments of barely bilingual translators found in Mechanical Turk settings and often used by MT researchers are likely to be quite different from expert professional translators whose reputations are defined by their work product. Competent human assessments (“gold standard”) are often at odds and different from the segments suggested as the best-scoring ones based on metrics like COMET or hLepor.
- Overreaching extrapolations: The limited evidence from these experiments was marketed as “human equivalence” by Google and others, and invariably resulted in disappointing professional translators and enterprise users who quickly witnessed the poor performance of these systems when they strayed away from news domain content. Though these claims were not deliberately deceptive, they were made to document progress from a perspective that was much narrower than the scope and coverage typical of professional translation work. There has never been a claim of improved MT quality performance based on the huge scale (across 2 billion segments) presented by Translated.
Translated finally provides compelling evidence
At the Association for Machine Translation in the Americas 2022 conference, Translated’s CEO, Marco Trombetti provided an initial preview of research they had been conducting over the last decade. The evidence of the steady progress can be quickly displayed in a single graph shown below and the research is presented in detail in the following article: This Is the Speed at Which We Are Approaching Singularity in AI.
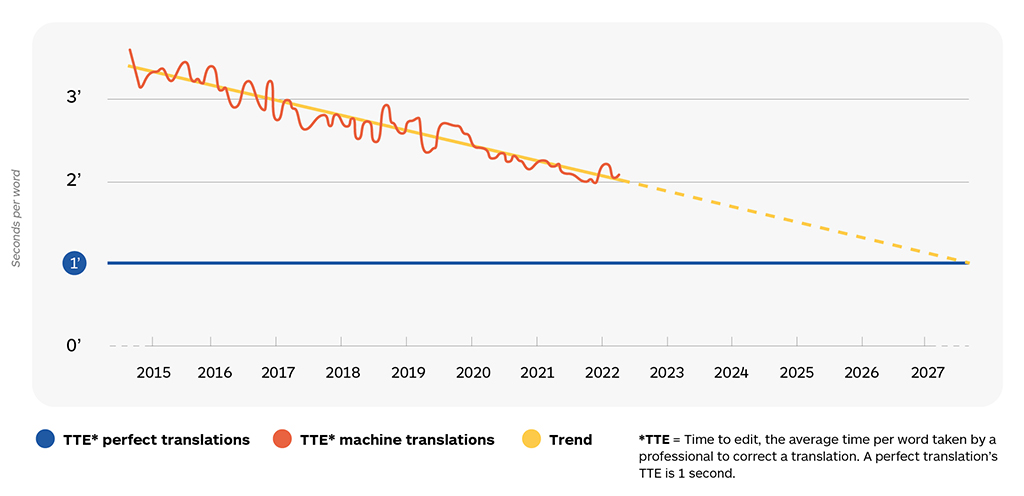
Source: Translated
The measurement used to describe ongoing progress with MT is Time To Edit (TTE). This is a measurement made during routine production translation work and represents the time required by the world’s highest-performing professional translators to check and correct MT-suggested translations. Translated makes extensive use of MT in their production translation work and has found that TTE is a much better proxy for MT quality than measures like Edit Distance, COMET, or BLEU. They have found that rather than using these automated score-based metrics, it is more accurate and reliable to use a measurement of the actual cognitive effort extended by professional translators during the performance of production work. Consistent scoring and quality measurement are challenging in the production setting because this is greatly influenced by varying content types, translator competence, and changing turnaround time expectations. A decade of careful monitoring of the production use of MT has yielded the data shown above. Translators were not coerced to use MT and it was only used when it was useful.
The history of MT is unfortunately filled with empty promises
The data are compelling because of the following reasons:
• The sheer scale of the measurements across actual production work is described in the link above. The chart focuses on measurements across 2 billion edits where long-term performance data was available.
• The chart represents what has been observed over seven years, across multiple languages, measuring the experience of professional translators making about 2 billion segment edits under real-life production deadlines and delivery expectations.
• Over 130,000 carefully selected professional translators contributed to the summary measurements shown on the chart.
• The segments used in the measurements are all no TM match segments as this represents the primary challenge in the professional use of MT.
• The broader ModernMT experience also shows that highly optimized MT systems for large enterprise clients are already outperforming the sample shown above which represents the most difficult use case of no TM match.
• A very definite linear trend shows that if the rate of progress continues as shown, it MAY be possible to produce MT segments that are as good as those produced by professional translators within this decade. This is the point of singularity at which the time top professionals spend checking a translation produced by the MT is not different from the time spent +checking a translation produced by their professional colleagues which may or may not require editing.
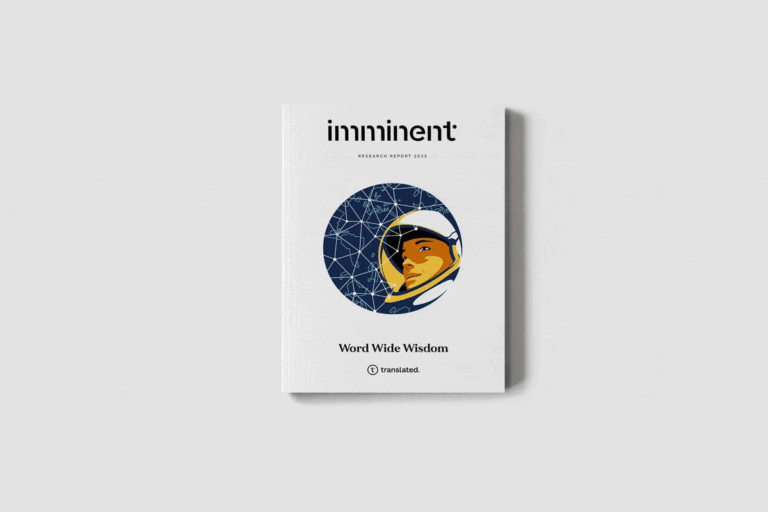
World Wide Wisdom
Research Report 2023
It is possibile to improve the understanding between people that speak different languages and thus improve their ability to do things together in a smarter way? Can it be that a multilingual group is able to do better things? In order to answer to these questions we need to take into account how groups of people think and work together and how their collaboration can be improved.
Get Your Copy Now!It is important to understand that the productivity progress shown here is highly dependent on the superior architecture of the underlying ModernMT technology which learns dynamically, and continuously, and improves on a daily basis based on ongoing corrective feedback from expert translators. ModernMT output has thus continued to steadily improve over time. It is also highly dependent on the operational efficiency of the overall translation production infrastructure at Translated.
The virtuous data improvement cycle that is created by engaged expert translators providing regular corrective feedback provides the right kind of data to drive ongoing improvements in MT output quality. This improvement rate is not easily replicated by public MT engines and periodic bulk customization processes that are typical in the industry.
The corrective input is professional peer revision during the translation process and this expert human input “has control,” and guides the ongoing improvement of the MT, not vice versa. While overall data, computing, and algorithms are critical technological foundations to ongoing success, expert feedback has a substantial impact on the performance improvements seen in MT output quality.
The final quality of translations delivered to customers is measured by a metric called EPT (errors per thousand words) which in most cases is 5 or even as low as 2 when two rounds of human review are used. The EPT rating provides a customer-validated objective measure of quality that is respected in the industry, even for purely human translation product when no MT is used.
There is a strong, symbiotic, and mutually beneficial relationship between the MT and the engaged expert translators who work with the technology. The process is quite different from typical clean-up-the-mess PEMT (post-editing machine translation) projects with customized static models where the feedback loop is virtually non-existent, and where the MT systems barely improve even with large volumes of post-edited data.
The problem with industry-standard automated metrics for MT quality assessment
It has become fashionable in the last few years to use automated MT quality measurement scores like BLEU, Edit Distance, hLepor, and COMET as a basis to select the “best” MT systems for production work. And some companies use different MT systems for different languages in an attempt to maximize MT contributions to production translation needs. These scores are all useful for MT system developers to tune and improve MT systems, however, globalization managers who use this approach may overlook some rather obvious shortcomings of this approach for MT selection purposes.
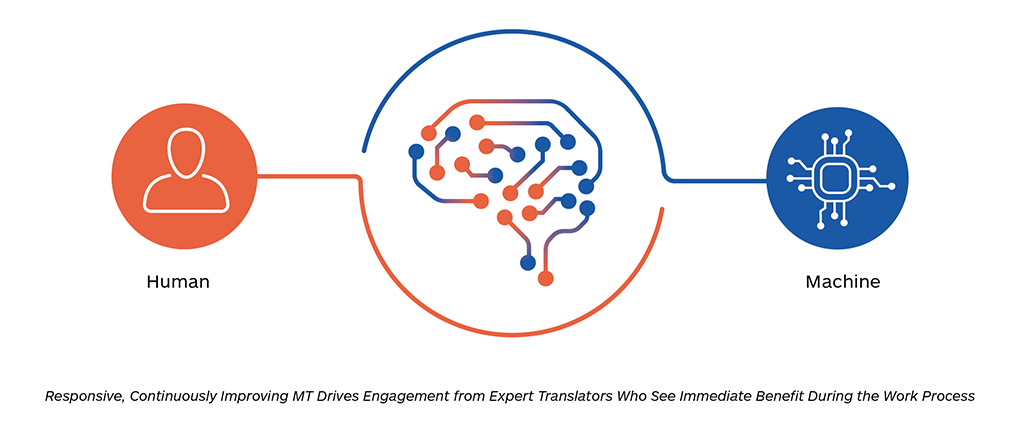
Here is a summary listing of the shortcomings of this best- MT-based-on-scores approach:
- These scores are typically based on measurements of static systems. The score is ONLY meaningful on a certain day with a certain test set and actual MT performance may be quite different from what the static score might suggest. The score is a measurement of a historical point and is generally not a reliable predictor of future performance.
- Most enterprises need to adapt the system to their specific content/domain and thus the ability of a system to rapidly, easily, and efficiently adapt to enterprise content is usually much more important than any score on a given day.
- These scores do not and can not factor in the daily performance improvements that would be typical of an adaptive, dynamically, and continuously improving system like ModernMT, which would most likely score higher every day it was actively used and provided with corrective feedback. Thus, they are of very limited value with such a system.
- These scores can vary significantly with the test set that is used to generate the score and scores can vary significantly as test sets are changed. The cost of generating robust and relevant test sets often compromises the testing process as the test process can be gamed.
- Most of these scores are only based on small test sets with only 500 or so sentences and the actual experience in production use on customer data could vary dramatically from what a score based on a tiny sample might suggest.
- Averaged over many millions of segments, TTE gives an accurate quality estimate with low variance and is a more reliable indicator of quality issues in production MT use. Machine translation researchers have had to rely on automated score-based quality estimates such as the edit distance, or reference-based quality scores like COMET and BLEU to get quick and dirty MT quality estimates because they have not yet had the opportunity to work with such large (millions of sentences) quantities of data collected and monitored in production settings.
- As enterprise use of MT evolves the needs and the expected capabilities of the system will also change and thus static scores become less and less relevant to the demands of changing needs.
- Also, such a score does not incorporate the importance of overall business requirements in an enterprise use scenario where other workflow-related, integration, and process-related factors may actually be much more important than small differences in scores.
- Leading-edge research presented at EMNLP 2022 and similar conferences provide evidence that COMET-optimized system rankings frequently do not match what “gold-standard” human assessments would suggest as optimal. Properly done human assessments are always more reliable in almost every area of NLP. The TTE measurements described above inherently allow us to capture human cognition impact and quality assessment at a massive scale in a way that no score or QE metric can today.
- Different MT systems respond to adaptation and customization efforts in different ways. The benefit or lack thereof from these efforts can vary greatly from system to system especially when a system is designed to primarily be a generic system. Adaptive MT systems like ModernMT are designed from the outset to be tuned easily and quickly with small amounts of data to fit a wide range of unique enterprise use cases. ModernMT is almost never used without some adaptation effort, unlike generic public MT systems like Google MT which are primarily used in a default generic mode.
A “single point quality score” based on publicly sourced sentences is simply not representative of the dynamically changing, customized, and modified potential of an active and evolving enterprise adaptive MT system that is designed to be continuously adapted to unique customer use case requirements.
When it is necessary to compare two MT systems in a buyer selection & evaluation process, doubleblind A/B human evaluations on actual client content would probably produce the most accurate and useful results that are also better understood by the executive and purchasing management. Additionally, MT systems are not static: the models are constantly being improved and evolving, and what was true yesterday in quality comparisons may not be true tomorrow. For these reasons, understanding how the data, algorithms, and human processes around the technology interact is usually more important than any static score-based comparison snapshot. A more detailed discussion of the overall MT system comparison issues is provided here.
A “single point quality score” based on publicly sourced sentences is simply not representative of the dynamically changing, customized, and modified potential of an active and evolving enterprise adaptive MT system that is designed to be continuously adapted to unique customer use case requirements.
Conducting accurate and consistent comparative testing of MT systems is difficult with either automated metrics or human assessments. We are aware that the industry struggles in its communications about translation quality with buyers. Both are easy to do badly and difficult to do well. However, in most cases, properly done human A/B tests will yield much more accurate results than automated metrics.
Questions to ask when looking at automated metrics: What specific data was used to calculate the score? How similar or different is it from my data? Can I see the data that was used? How easy or difficult is it to adapt this MT system to my specific linguistic style and preferences? How much effort is needed to teach this MT system to use my preferred style and language? Will I need ML experts to do this or can my translators drive this? Do small score differences really mean anything? What happens to these scores if I make changes to the test set? How quickly will this MT system improve as my translators provide daily corrections? Do my translators accept these score-based rankings if I show them the output from 3 different systems? Do my translators like working with this MT system? Will I be forced to use less qualified translators if I use this MT system as the best translators will prefer to decline?
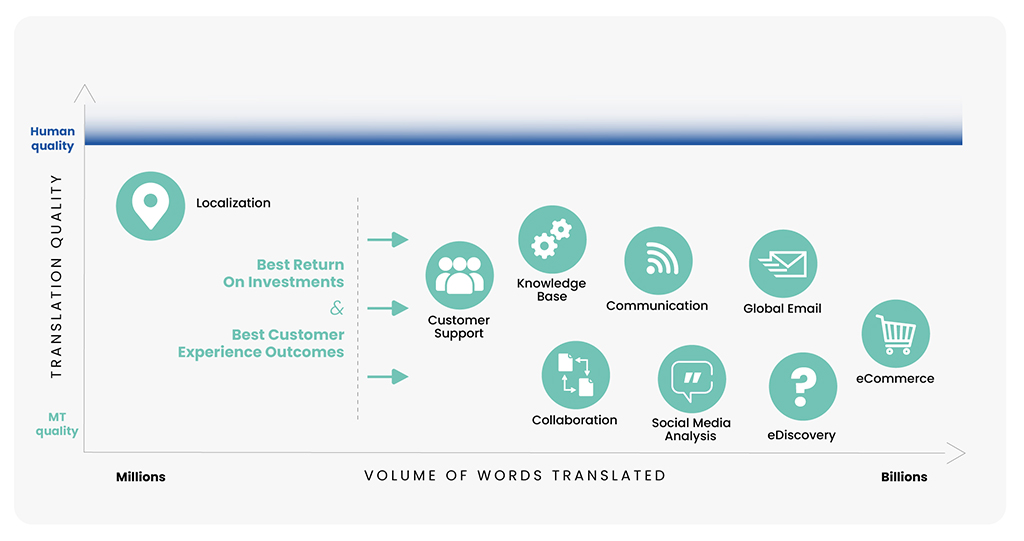
Source: Translated
The implications of continuously improving MT
Modern commerce is increasingly done with the support of online marketplaces and the importance of providing increasingly larger volumes of relevant content digitally to customers has become an important requirement for success.
As the volumes of content grow, the need for more translation also grows substantially. Gone are the days when it was enough for a global enterprise to provide limited, relatively static localization content.
Delivering superior customer experience (CX) requires much more content to be made available to global customers who have the same informational requirements as customers in the HQ country do. A deep and comprehensive digital presence that provides a broad range of relevant content to a buyer and global customer may be, even more, important to be successful in international markets.
The modern era requires huge volumes of content to support the increasingly digital buyer and customer journey. Thus, the need for high-quality, easily adapted machine translation grows in importance for any enterprise with global ambitions.
The success and relentless progress of the ModernMT technology described here make it an ideal foundation for building a rapidly growing base of multilingual content without compromising too much on the quality of translations delivered to delight global customers. This is critical technology needed to allow an enterprise to go multilingual at scale. This means that it is possible to translate billions of words a month at relatively high quality.
Adaptive continuously improving MT allows the Enterprise to go multilingual at billions of words/month scale. The availability of adaptive, highly responsive MT also enables new kinds of knowledge sharing to take place.
A case in point: Unicamullus Medical University in Rome experimented with using ModernMT to translate their medical journal into several new languages and test acceptance and usability. They were surprised to find that the MT quality was much better than expected. The success of the initial tests was promising enough to encourage it to expand the experiment and make valuable medical journal content available in 28 languages.
The project also allows human corrective feedback to be added to the publishing cycle when needed or requested. This machine-first and human-optimized approach is likely to become an increasingly important approach to large-scale translation needs when intelligent adaptive MT is the foundation.
It is quite likely that we will see possibly 1000X or more growth, in the content volume that is translated in the years to come, but also that we see a growing use of adaptive and responsive MT systems like ModernMT which are deeply integrated with active system-improving human feedback loops that can enable and drive this massive multilingual expansion.
There is increasing evidence that the best-performing AI systems across many areas in NLP have a well-engineered and tightly integrated human-in-the-loop to ensure optimal results in production use scenarios. The Translated experience with ModernMT is proof of what can happen when this is done well.
We should expect to see many more global companies translating hundreds of millions of words a month in the near future to serve their global customers. A future that will increasingly be machine-first and human-optimized.
The following article Translated CEO, Marco Trombetti, provides additional insight into the progress that we have witnessed with MT over a decade of careful observation and measurement. The interview highlights the many steps taken to ensure that all the measurements are useful KPIs in a professional translation services setting which has been and will continue to be the most demanding arena of performance for MT technology. Marco also points out that ModernMT and new Generative AI like ChatGPT are made of the same DNA, and that MT research has provided the critical technological building blocks used to make these LLMs (Large Language Models like ChatGPT) possible.
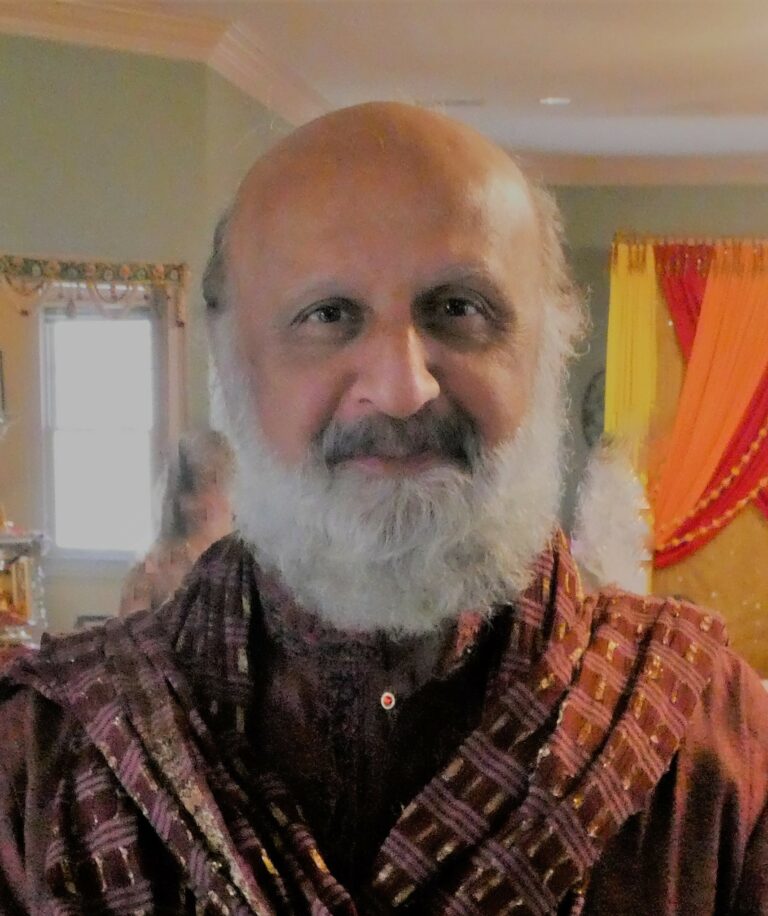
Kirti Vashee
Language technology evangelist at Translated
Kirti Vashee is a Language Technology Evangelist at Translated Srl, and was previously an Independent Consultant focusing on MT and Translation Technology. Kirti has been involved with MT for almost 20 years, starting with the pioneering use of Statistical MT in the early 2000s. He was formerly associated with several MT developers including Language Weaver, RWS/SDL, Systran, and Asia Online. He has long-term experience in the MT technology arena and prior to that worked for several software companies including, Lotus, Legato, & EMC. He is the moderator of the "Inner Circle" Automated Language Translation (MT) group with almost 11,000 members in LinkedIn, and also a former board member of AMTA (American Machine Translation Association). He is active on Twitter (@kvashee) and is the Editor and Chief Contributor to a respected blog that focuses on MT, AI and Translation Automation and Industry related issues.
Photo credit: Zak, Unsplash