Technology
Language is a complex social construct that never stops evolving, so AI can only partially address it. Guido Vetere emphasizes the need for a more comprehensive approach to account for the complexity of human linguistic understanding.
The interweaving of expressions, ideas, and reality that philosophers and linguists call “sign” is being pushed out of today’s AI. When processed by neural algorithms, the manifest part of the sign (signifier), i.e., utterances or writings, is detached from the other parts and treated like a number. Sentences thus become numeric strings. The neural linguistic mechanisms that are currently amazing us (including the famous ChatGPT) convey a vision of words as pure combinatorial elements. When fed into neural networks, what people actually mean with their words becomes quite irrelevant: what really
matters to algorithms is how words unfold into predictable arrays. AI structures learn the laws characterizing this process by looking at millions of sentences taken from petabytes of available digitized text in a way which, however sophisticated it may be, is ultimately statistical in nature.
By learning how words combine in millions of sentences, computers can make a reasonably accurate prediction of the subsequent words in a phrase, or can successfully estimate the similarity between two sentences, or whether a passage contains an answer to a question, and what the answer is likely to be. In particular, computers are increasingly able to translate from one language to another, as long as a vast amount of multilingual text is fed into the machine. Lexical semantics, i.e., the speculative study of meanings, is mostly considered a nuisance by those involved in modern AI, as if it were a relic of the dusty past of “semantic networks.” But is the notion of “sign” really dispensable when approaching linguistic tasks – translation in particular – with algorithms?
The neural linguistic mechanisms that are currently amazing us (including the famous ChatGPT) convey
a vision of words as pure combinatorial elements
Of course, it is more than legitimate to achieve linguistic goals, even complex ones such as translation, without any appeals to the notion of meaning. Indeed, linguists and philosophers have struggled to come up with a general “theory of meaning” for centuries, with more controversy than agreement. Moreover, statistical accounts of meaning are known in linguistics as well. Such approaches are rooted in the so-called “distributional hypothesis,” that became popular in the middle of the last century with the slogan: “you shall know a word by the company it keeps” (John R. Firth, A synopsis of linguistic theory, 1957). To grasp the idea, one can start by observing that the more semantically similar two words are, the more they tend to appear in similar sentences. This is an obvious consequence of what is called “synonymy,” meaning the possibility of replacing words in sentences without substantively changing their inherent truth. But the distributional hypothesis posits exactly the converse: words that frequently occur in the same context may, to some extent, be considered synonyms. From a logical point of view, this is certainly a very different claim: it amounts to the induction of truth-preserving equivalences based on distributions, i.e., (roughly) recurrent patterns. Taken literally, this claim sounds like a very dangerous logical fallacy. However, analyzing “big data” volumes reveals that distributions may effectively reveal, by induction, some vestiges of semantic relationships. Of course, such vestiges must be carefully handled: most of the notorious biases that affect neural language models arise from raw inductivism applied to uncontrollable data beyond any rational and social control.
Along with distributionism, contemporary scholars of J.R. Firth and mostly inspired by the works of Burrhus Skinner (1904- 1990) proposed a purely behavioral view of language, which went even further in attempting to eliminate theories of meaning altogether. According to behaviorists, understanding is merely the ability to associate appropriate responses to linguistic stimuli. Another very questionable tenet, indeed.
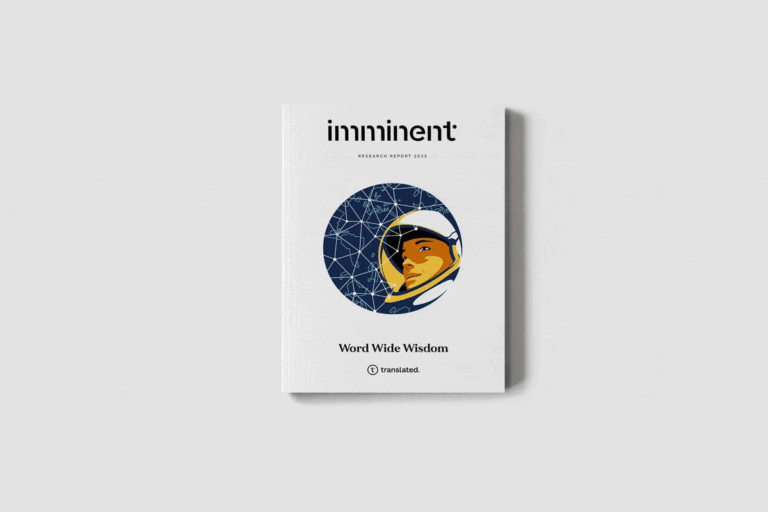
World Wide Wisdom
Research Report 2023
It is possibile to improve the understanding between people that speak different languages and thus improve their ability to do things together in a smarter way? Can it be that a multilingual group is able to do better things? In order to answer to these questions we need to take into account how groups of people think and work together and how their collaboration can be improved.
Get Your Copy Now!Nevertheless, nearly a century after their formulation, distributionism and behaviorism are paving the way for modern language technologies. The AI mainstream asserts that the more we add data and computation, the more we approximate the practical effects of human understanding, and that is all there is to it: we expect associative capabilities based on observed patterns from machines today. Many people, including philosophers, linguists, and computer scientists, are now struggling to sound the warning that erasing rational accounts of linguistic signs is not possible in principle: associative statistics cannot be promoted to the role of semantics entirely at once. Yet this debate is nothing new. At the beginning of his career, Noam Chomsky (1928 -) dismissed behavioral approaches to language (specifically those of Skinner) by pointing out that human understanding, whatever it is, has little to do with stimulus-response associations. There is no likelihood that we might understand a phrase like “the cat is on the mat” as a statement about the pet’s whereabouts instead of a statement on, say, quantum physics: we look at the cat straightaway. Neither does the language-learning process have a statistical dimension. Children do not learn that cats are in the same class as dogs (e.g., pets) because they often happen to hear the word “cat” in the same kind of phrases where “dog” occurs. Instead, they occasionally connect expressions with entities and situations, even fictional or absent ones, in real situations by playing with words or querying adults with metalinguistic questions driven by needs, feelings, and intuitions. According to this structure, they accumulate systematic knowledge of the world-language interplay over the years.
Language-learning is a complex social activity, as emerged from the findings of Russian cognitivist Lev Vygotsky (1896-1934) a century ago: it is not reducible to stimulus reinforcement, nor to statistical distributions (nor, for completeness, to human brain hardware, as Chomsky argued). In our time Judea Pearl, who received a Turing award for his studies on causal models, formally established that statistical correlation cannot climb what he calls the “ladder of causality” (Judea Pearl and Dana Mackenzie, “The Book of Why”, 2018). Simply put, a causal model is always a speculative human construction that cannot be derived from data, if only because, as statisticians like to say, “correlation is not causation.” It is easy to see how these studies apply to language and to show that the computation of textual correlations cannot produce any equivalent of human linguistic knowledge.
Indeed, understanding has a great deal to do with the formulation of interpretative hypotheses, which in turn have to do with how the world is made, as well as with the concrete situation of the utterances we hear or the imagery evoked by the text we are reading – in short: the causality of the world under the lens of subjective experience. In his writings, Umberto Eco vividly illustrated the interpretation of linguistic signs and its limitations, as an abductive (i.e., hypothetical) reasoning requiring a substantial body of both contextual and encyclopedic knowledge. Understanding everyday speech is quite similarto understanding linguistic works of art. Eco’s semiotic investigations specifically concern translation work (“Dire quasi la stessa cosa”, 2003).
Abductive reasoning fuels the interpretation of every single word in a sentence, and this reasoning concerns qualities rather than quantities. On the other hand, modern linguistic AI relies on quantities instead of qualities. So how is it possible that AI’s “incorrect” approach to language delivers results as spectacular as human-level chat or near-perfect translations? And how are language technologies going to deal with such a paradox? Perhaps we can seek an answer in a famous analogy Ludwig Wittgenstein drew in his Philosophical Investigations (1953): “Our language can be seen as an ancient city: a maze of little streets and squares, of old and new houses, and of houses with additions from various periods; and this surrounded by a multitude of new boroughs with straight regular streets and uniform houses.” In essence, language is not a coherent and homogeneous territory: rather, it is a set of places which, while sharing some fundamental properties, differ significantly in many respects, especially regarding functionality. The language of a novel comprises the same syntax as a loan agreement and largely the same lexicon, but the reasoning needed to enjoy the novel has little to do with that needed to fulfill the contract. The purpose of a novel is, above all, to draw people into imagery, while a contract must coldly denote things and states of affairs. Vagueness and ambiguity, and especially metaphors, may be desired effects for poets, but they are poison for lawyers. It is reasonable to consider that in the space of all the various and diverse linguistic purposes, there are many relevant regions where semantically blind language technologies can be successful without endangering humankind at all (some of the “generative” platforms that have recently burst onto the scene do not seem so harmless, but that’s another story).
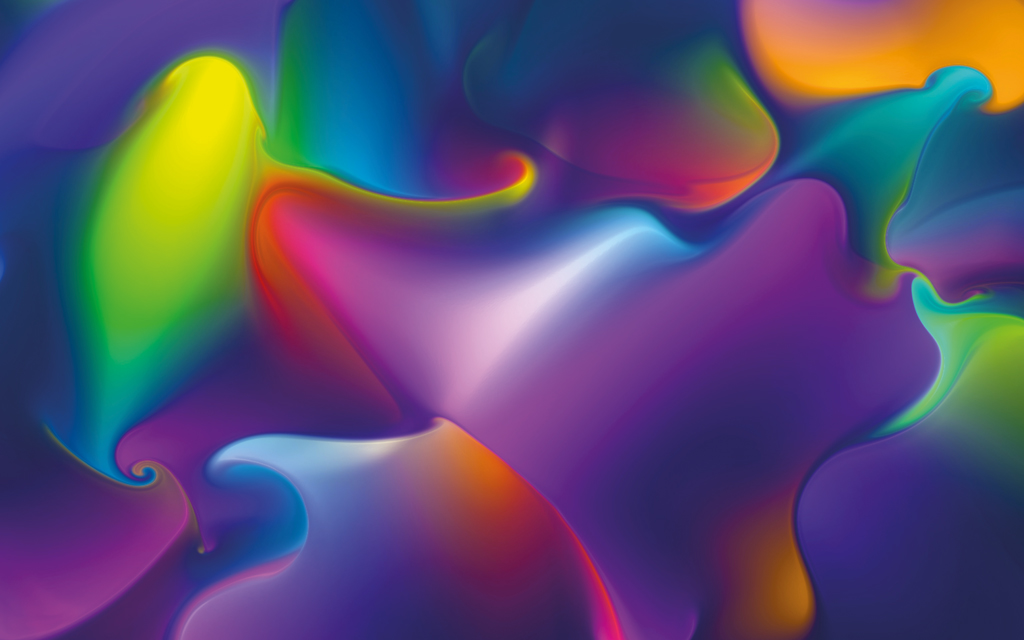
Machine translation is probably a field where AI technologies can be unleashed without restraint, as in fact they are. The task of transforming an array of numbers (i.e., a sentence, as the machine sees it) into another array of numbers that is equivalent under a certain function (i.e., keeping quite the same meaning) is ethically non-demanding, as long as the function injects nothing malicious or inappropriate. As for quality, it’s not that a machine can translate Proust into Italian better than, say, Natalia Ginzburg, but that millions of texts are produced every day that Natalia Ginzburg, if she were still alive, wouldn’t be willing to translate. And it’s not that a machine can solve all the translation puzzles that professional translators are often involved in, but that the solution of these puzzles can be statistically approximated for most business texts. We learned from Ferdinand de Saussure (1857-1913) that the relationship between expression (signifiant) and meaning (signifié) is arbitrary: each language, therefore, has a specific semantic imprint, and the mapping of these imprints is often hazy, especially for languages belonging to different families. But even within the same language, what a speaker ontologically commits to when uttering a sentence is largely inscrutable, as Willard V.O. Quine (1908 – 2000) pointed out, and any act of understanding is always a sort of “radical” (“arbitrary”) translation (Word and Object, 1960). If comprehension and translation are so ineffable even for humans, why should we hesitate when delegating tasks to automated approximations? Why not use (educated) statistics to achieve our objectives instead of reasoning?
The neural linguistic mechanisms that are currently amazing us (including the famous ChatGPT) convey
a vision of words as pure combinatorial elements
Language-learning is a complex social activity: it is not reducible to stimulus reinforcement, nor to statistical distributions.
This line of reasoning appears quite solid. Yet it has its limits, and when the translation game becomes complex, it is easy to see that the sign as the underlying unit of semantics cannot be entirely eliminated without risk. There are technical reasons for this. Today’s machine translation is a sequence of plausible transformations of source-language strings into target-language strings, where plausibility is gained from sophisticated statistics and tons of textual data. But texts do not encode all that is required to interpret them As Jacques Der- rida (1930-2004) said, “il n’y a pas de horstexte.” This famous aphorism is often mistranslated as “there is nothing outside the text.” Actually, Derrida intended quite the opposite: all the facts of the world, including ideological ones, concur in the interpretation of any text, so there is (virtually) nothing that can be excluded from the process of understanding, be it human or not. Linguistic data are always unsaturated; humans must always envisage what the text entails. Metaphors are key examples. To decide whether an expression like “cherry picking” should be taken literally (harvesting small red fruits) or as a metaphoric “frozen” sentence (in a discussion, choosing only the most beneficial examples from what is available), one must know the context in which the sentence is framed. Still today, Google translates “cherry picking is an argumentative fallacy” as “la raccolta delle ciliegie è un errore argomentativo” in Italian (“cherry harvesting is an argumentative fallacy”). To avoid such errors, given two available meanings (the literal and the metaphoric one), the machine should understand how the predicative complement (“argumentative fallacy”) fits the subject (“cherry picking”). To do this, semantic frames (i.e., sign structures) are needed: “argumentative fallacy” should trigger a frame in which fruit simply fails to align with the context. Some AI people seem to think that by providing petabytes of text and scaling neural networks to billions of parameters, such disambiguation puzzles can be solved by chance on a neural-statistical basis. But that is akin to believing that since the roof is closer to the sky than the cave, we can build a skyscraper to get to the moon. It’s a quantitative illusion. No data-driven statistical algorithm can ever hope to match the ephemeral creativity of human language, which enables us to utter and interpret metaphors on the fly. No legacy texts can determine the linguistic images we have yet to invent. At least, that’s the hope
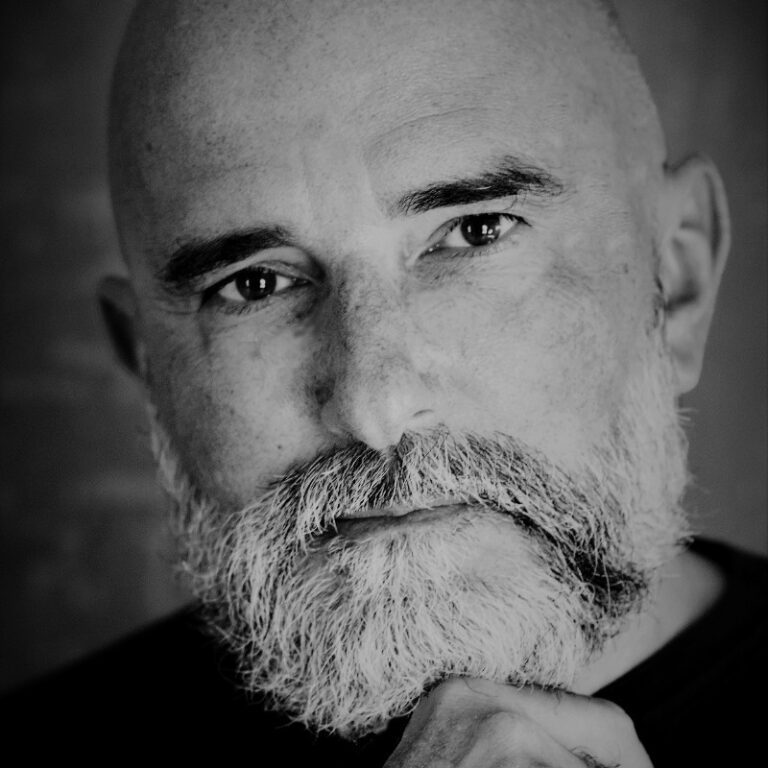
Guido Vetere
Knowledge and Language Research
Guido Vetere graduated in Philosophy of Language under the guidance of Prof. Tullio De Mauro at Rome Sapienza University, with a dissertation on computational linguistics. Then he spent most of his career at IBM Italia, where he has been leading the Center for Advanced Studies in Rome and Trento. He is now Adjunct Professor of Artificial Intelligence at the Faculty of Applied Sciences and Technologies of the University of Guglielmo Marconi in Italy and head of Isagog Srl, an Italian AI startup. His research interests range from logic, knowledge representation and automated reasoning to computational linguistic models and resources.
Photo credit: Donny Jiang, Unsplash