Trends + Research
The internet has brought many changes and given a lot of opportunities for connecting with each other, hence it’s called a global village. One of the advantages of a global village is the ability for people to communicate with each other and this has been largely facilitated by the use of lingua francas such as English however, many other languages have less representation on the digital landscape. The Languages Technology for All (LT4All) conference held in 2019 organized by UNESCO notes that of the over 7500 languages found across the world, less than 100 are covered on the digital landscape and of those 100 less than 10 languages have a relative coverage of services and applications for language technologies. This shows that there is no equal representation of languages as some languages have more resources and tools resulting in better coverage and use more than others. Looking at the situation in most African countries, the languages of the former colonizers, are used as the lingua franca, whilst the native languages are reserved for informal situations. More linguistic resources that include machine readable texts have been produced for the lingua franca as opposed to the native languages. Languages resources represent the datasets which are used to build natural language technologies and these resources are the foundation from which any activity can be built upon. The United Nations General Assembly recently declared the 10-year period between 2022 and 2032 as the “International Decade of Indigenous Languages”. This was declared with an impetus to “preserve, revitalize and promote” indigenous languages and has been proposed due to the dire state of indigenous languages around the world.
There has been a lot of investments in the development and curation of linguistic resources like books has led to the classification of these lingua franca to be “high-resourced”. Although this has advanced language technologies in general, this has also come at the expense of the “low-resource languages” as there is less investment into technologies which make it easier for native speakers. When efforts have been made to work on these languages, the results have been good but not comparable to those obtained for the more widely studied languages. Most people who work on creating language technologies do not speak the low-resource languages, so they cannot really measure how effective these tools are. Low representation is the problem, that, as stated by LT4All, puts “native speakers of those under-resourced languages in a disadvantageous situation, creating a digital divide, and places their languages in danger of digital extinction, if not complete extinction”.
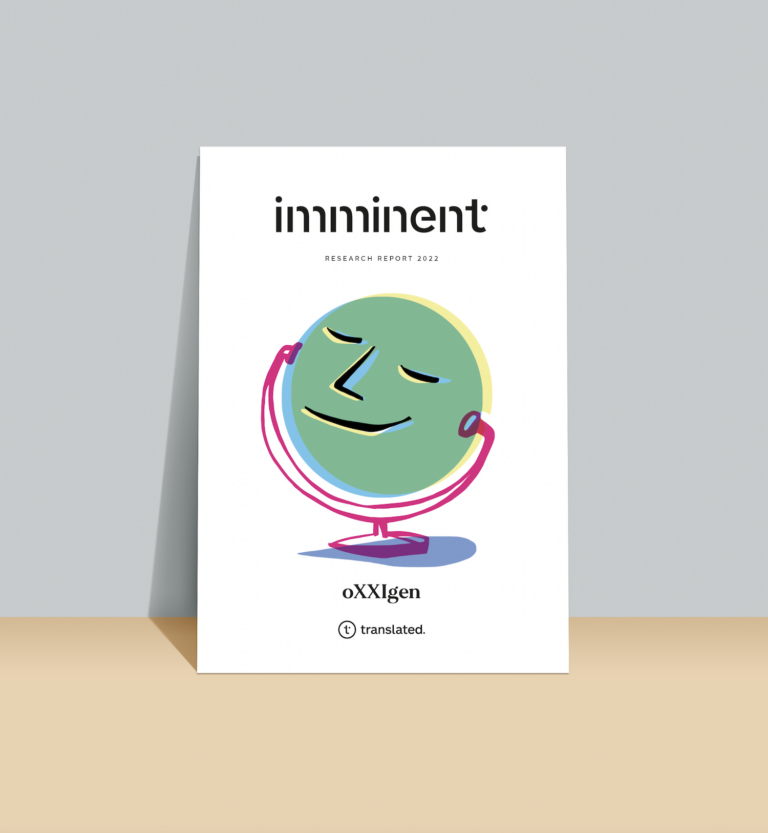
oXXIgen
Imminent Research Report 2022
GET INSPIRED with articles, research reports and country insights – created by our multicultural interdisciplinary community of experts with the common desire to look to the future.
Get your copy nowDue to the lack of attention for indigenous languages, there has recently been a new impetus and establishes language resources, training algorithms and language models as the three key ingredients which are fundamental for improvement and representation of languages as part of its digital strategy. Although training algorithms and languages models are very important this article will mainly focus on the language resources. For the perspective of African languages, (Martinus & Abbott, 2019) identify two main problems with resources which are availability and discoverability. With low availability of resources, there is lack of data that can be used to train algorithms for AI which makes it hard to work on these languages. For the few resources that are available, there are not easy to find which also renders them difficult to curate and work with. The issue of the lack of datasets is a foundational issue as every other process of building language technologies depends on it. On a broader perspective, the AI4D report notes that most African organizations do not have the required resources, protocols and infrastructure to support the creation of representative datasets. So, in essence there is need for a concerted effort to help create datasets as foundation for any language technology to be built.
Initiatives have sprung up which are working to address these issues with organizations such as Knowledge for All creating fellowships for the creation of datasets for low-resource African languages. This initiative has resulted in the creation of datasets for 9 languages, which are spoken across more than 20 countries in Africa. In 2020 an organization called the Lacuna fund was initiated which has the aim of funding the collaborative creation of datasets for the across computer vision for agriculture, health and most importantly language datasets for low resources African languages. These initiatives are very welcome as they help solve the biggest issue to working with language technologies. Furthermore, the people who speak these languages are the forefront of creating these resources and are able to utilize them in a way that benefits their own people.
I am big believer in the notion that native speakers of the languages should be on the forefront of creating tools and technologies as they are well acquainted with the intricacies of their own language. Some issues that come along with this are the issues which relate to barriers of entry into creating tools for language technologies. At most, people are afraid to take this up as there is an assumption that you need to be a technology expert to contribute to the creation of these technologies, which is actually not the case. This has been demonstrated for example by Masakhane community started in 2019 which is a “A grassroots NLP community for Africa, by Africans” and nurtures the idea of Africans being at the forefront of working on African NLP. Since its inception, one of the pivotal works they have done is a paper they published on participatory research (Nekoto et al., 2020) for African languages. This work resulted in a publication of benchmarks for 40 African languages for the Machine Translation (MT) task.
A participatory approach to research involves engagement of individuals who are not necessarily trained and skilled in research but are directly affected with the output of the research activities (Vaughn & Jacquez, 2020). With this approach participants become stakeholders as they get affected by outcomes of research and on the other hand are involved in working to find a solution to a problem. One area that can benefit from a participatory approach is the creation of language datasets for building language technologies. A participatory approach to building language technology tools is beneficial as it firstly lowers the barriers of entry. In this approach everyone does not need to be an expert at building and training models but they can be an expert at something that helps the creation of the tools. For example, for the MT process by Masakhane, five agents were identified who are involved in the process of building of machine translation models namely, content creators, translators, curators, language technologists and evaluators. Content creators prepare the content which is monolingual in their indigenous language. The translators have the role of translating this content into a different language such as English. The source text and the translated text are then selected and organized by a curator into a parallel dataset that can be used for the model building process. All these roles need expertise which is critical to the building of the datasets which contribute immensely to the process of model building. On the other side of the model creation process, there are language technologists and evaluators. The language technologists are responsible for the model building whilst the evaluators evaluate the trained models to make sure they are making quality translations. All these roles and processes they are involved in are better shown in figure 1 from the Masakhane publication. These roles are not concrete but can be fluid as one agent can wear many hats especially when they are proficient in the use of the native language. As an example, an agent can be a curator, in making sure that data is in a format that can be used for model building whilst at the same time be a language technologist who builds the models. This can go further into being an evaluator to make sure that the models are providing quality output.
Although the specific use case for the Masakhane paper was limited to one language technology which is machine translation, this approach is certainly useful in other uses cases. A lot of data is being amassed by private companies in local languages which can be useful when annotated for use cases such as disinformation and hate speech (Marivate, 2020). Other use cases such as information extraction from unstructured text through identifying named entities will benefit from a participatory approach.
Preservation of native languages needs a proactive approach by the native speakers instead of waiting for the tech companies to take up the initiative. The information age has opened up a lot of avenues to gain knowledge on how to effectively build tools and technologies which help bring the native languages onto the digital landscape. The barriers of entry have been lowered and everyone can take a part in contributing to preservation of native languages through a participatory approach. Creating resources will provide a ripple effect where language technologies can be built and these language technologies will allow people to use their native languages more. When people use their native languages more, it will lead to even more resources which ultimately improve the language technologies for those languages.
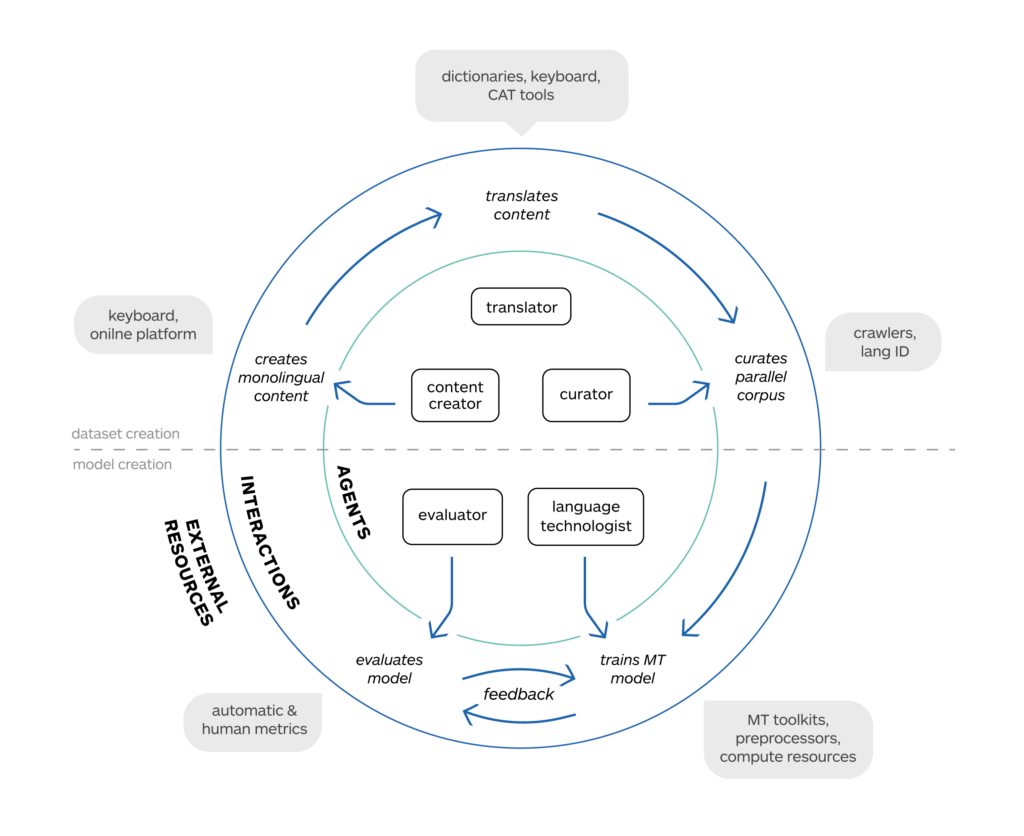
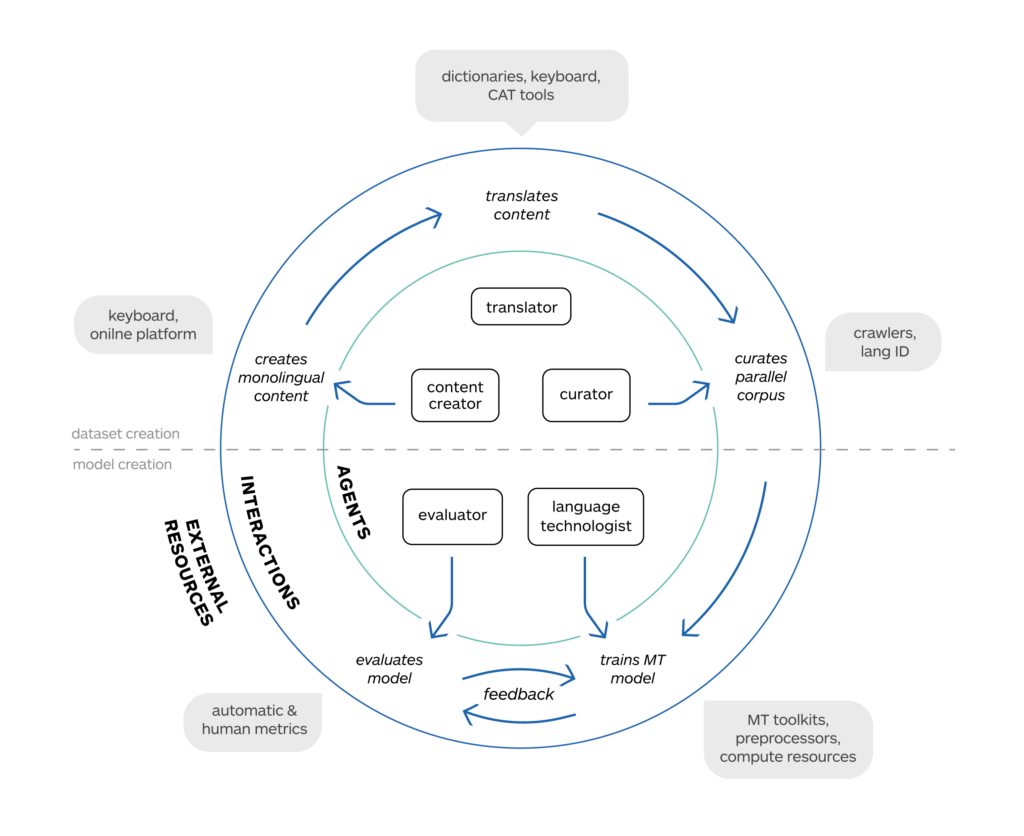
SHONA
Kuchengetedza kuburikidza nekutora chikamu
Dandemutande rakaunza shanduko dzakawanda uye rakapa mikana yakawanda yekubatana, nekudaro inonzi musha wepasi rose . .Chimwe chezvakanakira musha wepasi rose kugona kwevanhu kutaurirana pachavo uye izvi zvakafambiswa zvakanyanya nekushandiswa kwe mitauro yakaita seChirungu zvisinei, mimwe mitauro mizhinji ine humiriri hushoma pamadandemutande.. Musangano we Languages Technology for All (LT4All) wakaitwa muna 2019 wakarongwa neUNESCO unoona kuti pamitauro inopfuura mazana manomwe nemazana mashanu inowanikwa pasi rose, isingasviki zana ndiyo inotaridzwa padigital landscape uye pamitauro iyi zana, mitauro isingasviki gumi ndiyo ine zvikwanisiro zvekuti ishandiswe paunyanzvi hwemitauro . Izvi zvinoratidza kuti hapana kumiririrwa kwakaenzana kwemitauro sezvo mimwe mitauro iine zviwanikwa uye maturusi akawanda zvinoita kuti pave nekufambiswa kuri nani nekushandiswa zvakanyanya kupfuura mimwe. Tichitarisa mamiriro akaita zvinhu munyika zhinji dzemuAfrica, mitauro yevaimbova vapambi, inoshandiswa semitauro yekutaura, nepo mitauro yemo ichichengeterwa mamiriro asina kurongwa. Zvimwe zvinyorwa zvemitauro zvinosanganisira zvinyorwa zvinoverengeka nemuchina zvakagadzirirwa mitauro yevapambi kupfuura mitauro yemo. Zviwanikwa zvemitauro zvinomiririra dhataseti rinoshandiswa kuvaka matekinoroji emutauro wechisikirwo uye zviwanikwa izvi ndihwo hwaro panogona kuvakirwa chero chiitiko. United Nations General Assembly ichangobva kuzivisa nguva yemakore gumi pakati pa2022 na2032 se “Makore gumi eMitauro Yechivanhu”. Izvi zvakaziviswa nechisungo “chekuchengetedza, kumutsidzira nekusimudzira” mitauro yechivanhu uye zvakakurudzirwa nekuda kwemamiriro akaipisisa akaita mitauro yechivanhu pasi rose.
Pakave nekudyara kwakawanda mukusimudzira nekugadzirisa zviwanikwa zvemitauro senge mabhuku zvakakonzera kurongwa kwemitauro iyi kuti inzi “zviwanikwa-zvepamusoro”. Kunyangwe izvi zvavandudza matekinoroji emitauro jekerere, izvi zvauyawo nekutsikirirwa kwe “mitauro yechivanhu” sezvo paine kushomeka kwekudyarwa kwehunyanzvi hwekuita kuti zvive nyore kune vatauri vayo. Apo nhamburiko dzakaitwa dzokushanda pamitauro iyi, mhedzisiro yaive yakanaka asi isingaenzaniswi neyakawanikwa pamitauro inodzidzwa zvikuru. Vanhu vazhinji vanoshanda mukugadzira matekinoroji emitauro havataure mitauro yechivanhu, saka havagone kunyatso kuyera kuti maturusi aya anoshanda zvakanaka sei. Kumiririrwa kushoma ndiro dambudziko, sezvakataurwa neLT4All, inoti “vatauri vemitauro yechivanhu isinganyanyoshandiswa, mumamiriro ezvinhu asina kunaka, zvichigadzira kupatsanurwa kwedhigitari, uye kuisa mitauro yavo munjodzi yekutsakatika, kana kusiri kutsakatika zvachose”.
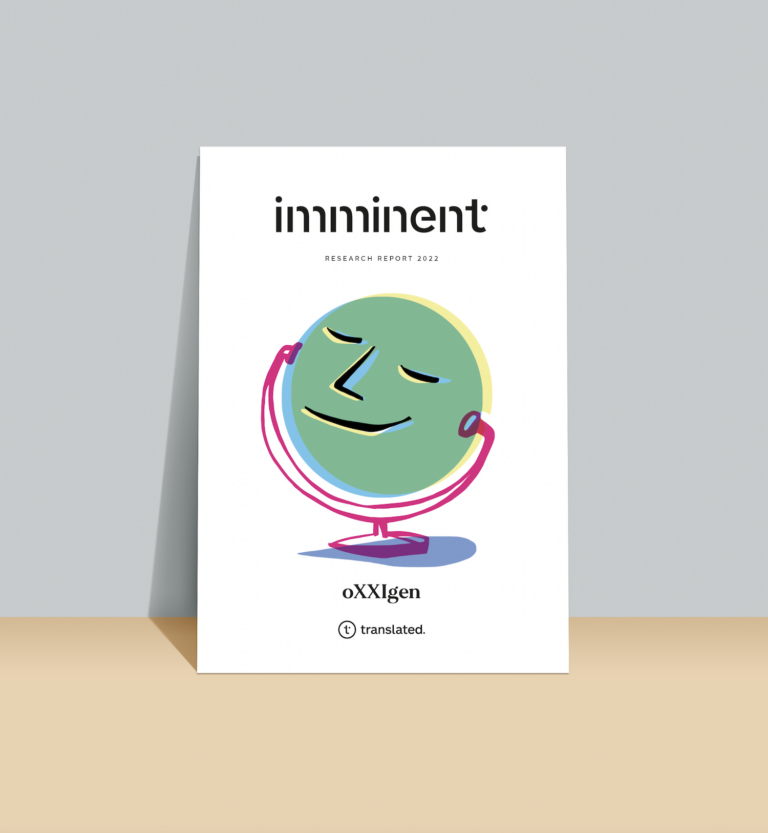
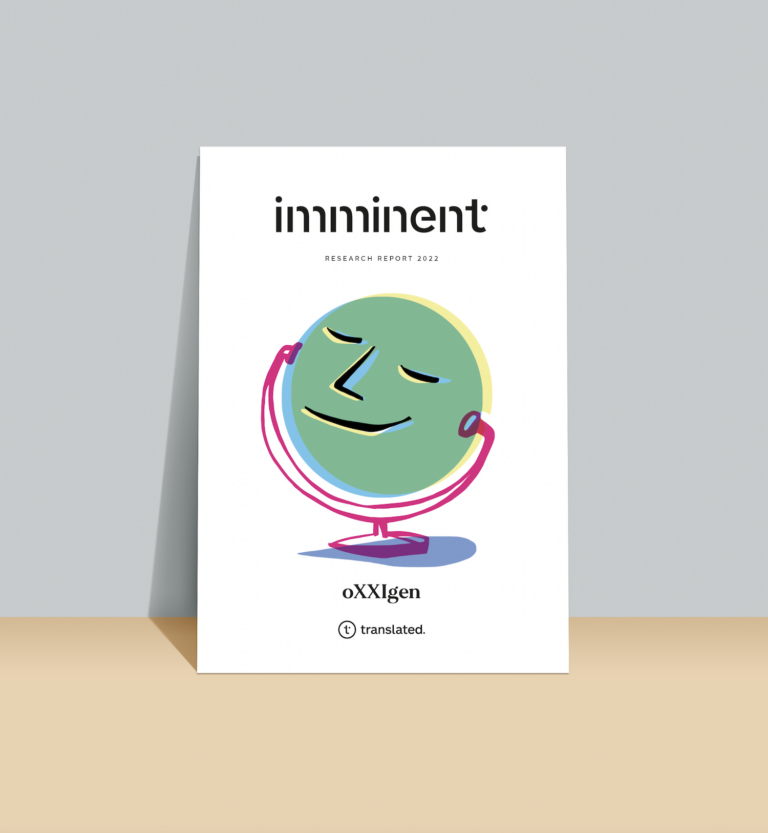
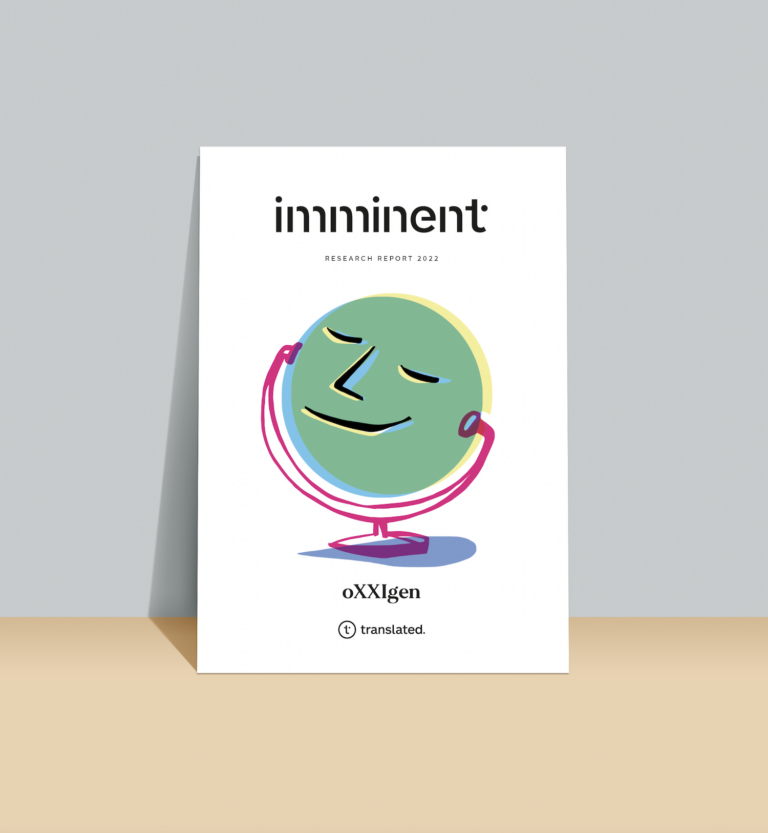
oXXIgen
Imminent Research Report 2022
GET INSPIRED with articles, research reports and country insights – created by our multicultural interdisciplinary community of experts with the common desire to look to the future.
Get your copy nowNekuda kwekusatariswa kwemitauro yechivanhu, nguva diki yapfuura pakava nesimba idzva nechido chekugadzira michina yemitauro yechivanhu muAfrica. Mitemo ye EU ye tekinorogi yemitauro inogadza zviwanikwa zvemitauro, kudzidzisa maalgorithms uye mhando dzemitauro , sezvinhu zvitatu zvakakosha zvekuvandudza nekumiririra mitauro sechikamu chenzira yedhijitari. Kunyangwe kudzidzisa maalgorithms ne mhando dzemitauro kwakakosha chinyorwa chino chinonyanyo tarisa pane zviwanikwa zvemutauro. Pamaonero emitauro yemuAfrica, (Martinus & Abbott, 2019) vanonongedza matambudziko makuru maviri ane zviwanikwa anoti mawanikirwo uye maonekerwo. Nekuwanikwa kwakaderera kwezviwanikwa, pane kushomeka kweruzivo runogona kushandiswa kudzidzisa maalgorithms kune vose izvo zvinoita kuti zviome kushanda pamitauro iyi. Pazviwanikwa zvishoma zviripo, hazvisi nyore kuwana izvo zvakare zvinoita kuti zviome kugadzirisa uye kushanda nazvo. Nyaya yekushaikwa kwemadataset inyaya inehwaro hukuru sezvo mamwe maitiro ekuvaka mazano emitauro anobva pairi. Pamaonero akavandudzwa, gwaro reAI4D rinoona kuti masangano mazhinji emuAfrica haana zviwanikwa zvinodikanwa, maprotocol uye zvivakwa zvekutsigira kuumbwa kweruzivo runomiririra. Saka, muchidimbu panodiwa kuedza kwakubatirana kubatsira kugadzira dhataseti sehwaro hwechero tekinoroji yemutauro ichavakwa.
Mazano amuka ayo ari kushanda kugadzirisa nyaya idzi nemasangano akaita seKnowledge for All creating fellowships , kugadzira madhataseti emitauro yechivanhu yemuAfrica. Chirongwa ichi chakonzera kuti pave ne kuumbwa kwemadataseti emitauro mipfumbamwe , iyo inotaurwa munyika dzinopfuura makumi maviri muAfrica. Muna 2020 sangano rinonzi Lacuna fund rakavambwa iro rine donzvo rekupa mari mukubatanidzwa kwedataseti echiono chekombiuta chezvekurima, hutano uye zvakanyanya kukosha madhatabhesi emitauro yechivanhu yemuAfrica. Zvirongwa izvi zvinogamuchirwa zvikuru sezvo zvichibatsira kugadzirisa nyaya huru pakushanda neruzivo rwemitauro. Pamusoro pezvo, vanhu vanotaura mitauro iyi ndivo vari pamberi mukugadzira zviwanikwa izvi uye vanokwanisa kuzvishandisa nenzira inobatsira vanhu vavo.
Ndinotenda zvikuru mupfungwa yekuti vatauri vemitauro iyi vanofanira kunge vari pamberi pakugadzira maturusi neruzivo rwemichina sezvo vachinyatsoziva hunyanzvi hwemitauro yavo. Dzimwe nyaya dzinouya neizvi inyaya dzine chekuita nezvipingaidzo zvekupinda mukugadzira maturusi ehunyanzvi hwemitauro. Kazhinji, vanhu vanotya kutora izvi kumusoro sezvo paine fungidziro yekuti iwe unofanirwa kuve nyanzvi yehunyanzvi kuti ubatsire mukugadzirwa kwehunyanzvi uhu, izvo zvisiri izvo. Izvi zvakaratidzwa semuenzaniso nenharaunda yeMasakhane, yakatanga muna 2019 inova “Nzvimbo yeNLP yeAfrica, nemaAfricans” uye inosimudzira pfungwa yekuti maAfrica ave pamberi pekushanda paAfrican NLP. Kubva payakavambwa, rimwe remabasa akakosha avakaita igwaro ravakaburitsa patsvagiridzo yemubatanidzwa (Nekoto et al., 2020) yemitauro yemuAfrica. Iri basa rakakonzera kuburitswa kwezviyero zvemitauro makumi mana yemuAfrica yeMachine Translation (MT) task.
Maitirwo ekutora chikamu patsvagiridzo anosanganisira kubatanidzwa kwevanhu vasina kudzidziswa uye nehunyanzvi mukutsvakurudza asi vanokanganiswa nekubuda kwezviitwa zvetsvakurudzo (Vaughn & Jacquez, 2020). Nenzira iyi vapinda muchirongwa vanove nechikamu sezvo vachikanganiswa nezvakabuda mutsvagiridzo uye nerumwe rutivi vanobatanidzwa mukushanda kutsvaga mhinduro kudambudziko. Imwe nzvimbo inogona kubatsirika kubva kunzira yekutora chikamu ndeyekugadzira madhataseti ehunyanzvi hwemitauro. Nzira yekutora chikamu pakuvaka maturusi ehunyanzvi hwemutauro inobatsira sezvo inotanga kudzikisa zvipingamupinyi zvekupinda. Nenzira iyi munhu wese haafanire kuve nyanzvi pakuvaka uye kudzidzisa nzira asi vanogona kuve nyanzvi pane chimwe chinhu chinobatsira kugadzirwa kwezvishandiso. Semuenzaniso, pachirongwa cheMT chakaitwa naMasakhane, vamiririri vashanu vakatsvagwa vari kuita basa rekuvaka michina yekududzira nzira inoti, vagadziri venyaya, vadudziri, vachengeti,
nyanzvi dzemutauro uye vaongorori. Vagadziri venyaya vanogadzira nyaya dzine mutauro mumwe chete mumitauro yavo. Vaturikiri vane basa rekuturikira nyaya mumutauro yakasiyana seweChirungu. Kwakabva zvinyorwa uye zvinyorwa zvakaturikirwa zvinobva zvasarudzwa nekurongwa nemuchengeti kuisa munerimwe dataseti rinogona kushandiswa pakuita nzira yekuvaka. Ese mabasa aya anoda hunyanzvi hunova hwakakosha pakuvaka dhataseti anonobatsira zvakanyanya mukuita kwekuvaka nzira. Kune rumwe rutivi rwemavakirwo enzira, kune nyanzvi dzemitauro uye vaongorori. Vanamazvikokota vemutauro ndivo vane basa rekuvaka nzira apo vaongorori vanoongorora nzira kuti vave nechokwadi kuti vari kuturikira zvemhando yepamusoro. Ese mabasa aya nemaitiro avanobatika maari anoratidzwa zviri nani mumufananidzo 1 kubva mubhuku reMasakhane. Aya mabasa haana kusimba asi anogona kubatanidzwa sezvo mumiririri mumwe chete anogona kupfeka nguwani zhinji kunyanya kana aine ruzivo mukushandisa mutauro weko. Semuenzaniso, mumiririri anogona kuve muchengeti, mukuita chokwadi chekuti ruzivo ruri muchimiro chinogona kushandiswa pakuvaka nzira panguva imwe cheteyo ari nyanzvi yemutauro anovaka nzira. Izvi zvinogona kuenderera mberi kuti ave muongorori kuti ave nechokwadi chekuti nzira dzirikubuda zvakanaka.
Kunyangwe kushandiswa kwemuenzaniso webepa reMasakhane kwaive kwakaganhurirwa kune mutauro mumwe chete unova kududzira kwemuchini, nzira iyi inobatsira mune imwe mienzaniso. Ruzivo ruzhinji ruri kuunganidzwa nemakambani akazvimirira mumitauro yemo izvo zvinogona kubatsira kana zvichitsanangurwa semuenzaniso inosanganisira kunyeperana uye kushandisa mutauro weruvengo (Marivate, 2020). imwe miyenzaniso yakadai se sekutorwa kwenyaya kubva muzvinyorwa zvisina kurongeka kuburikidza nekunongedzerwa kwemasangano anozivikanwa anozobatsirikana mukutora chikamu.
Kuchengetedzwa kwemitauro yemuno kunoda nzira inobatika nevatauri veko pane kumirira kuti makambani ehunyanzvi kuti atore matanho. Zera reruzivo rakavhura nzira dzakawanda dzekuwana ruzivo rwekuvaka nemazvo maturusi uye matekinoroji anobatsira kuunza mitauro yemuno padhijitari. Zvimhingamupinyi zvekupinda zvakadzikiswa uye munhu wese anogona kutora chikamu mukubatsira kuchengetedzwa kwemitauro yemuno kuburikidza nenzira yekutora chikamu . Kugadzira zviwanikwa kuchapa mhedzisiro apo matekinoroji emitauro anogona kuvakwa uye matekinoroji emitauro aya anobvumira vanhu kushandisa mitauro yavo yekuzvarwa zvakanyanya. Kana vanhu vakashandisa mitauro yavo yekuzvarwa zvakanyanya, zvinovatungamira kuzviwanikwa zvakatowanda izvo zvinozovandudza ruzivo rwemitauro yemitauro iyoyo.
Bibliography
Marivate, V. (2020). WHY AFRICAN NATURAL LANGUAGE PROCESSING NOW ? A VIEW FROM SOUTH AFRICA # AFRICANLP Reflections on how machines learn to unearth. Mapungubwe Institute Od Strategic Reflection (MISTRA), November, 1–24.
Martinus, L., & Abbott, J. Z. (2019). A Focus on Neural Machine Translation for African Languages.
Photo credits: Trust Tru Katsande, Unsplash