Trends
AI will gain a more important role in our lives as we progress. Author Massimo Chiriatti explains why, in order for humans to govern AI, we need to understand how it creates a new dimension of thinking.
Introduction
When you read a generic text, you don’t know who or what the author is; in any case, you are in a state of consciousness, and the words stimulate both your instincts and your thoughts. The idea is that two neural networks are being compared: one artificial, which has
tried to produce a text, and the other human, which has tried to understand it. This game plays out on a daily basis and is a challenge that is unlikely to end now that it is almost impossible to discern the true nature of authorial sources. Whether we have been convinced that a human has written the words remains to be seen. In this case, as Aristotle would say, there would be an “agnition”, i.e., the unexpected recognition of a subject’s identity.
In online interactions, the machine will understand whether it has convinced you or not from your reactions, and in the latter case it will change register until it gets its result, without the need for human intervention. There is no one behind, governing the machine at that moment: human intervention is always in front.
Perhaps the reader was unable to distinguish whether the text was written by a machine or a human being, almost as if the machine had passed the Turing test. (Warning: to pass it completely, it would have had to be asked a direct question and answered appropriately.) The Turing test has also been criticized because it was based only on a linguistic paradigm, whereas language is a necessary component of intelligence but not the only one. This is why we should not judge artificial agents like Alexa, Siri, Cortana, etc. as truly “intelligent”: they do not have the ability to understand the meaning of the words they use.
Here we define intelligence as the ability to accomplish complex objectives, but we view Artificial Intelligence as a discipline that helps us study the footprints of the past to suggest future steps.
There is no one behind, governing the machine at that moment: human intervention is always in front.
Artificial Intelligence is used to analyze enormous amounts of data in a timely manner and translate them into useful decision-making information. But we must be careful not to confuse intelligence with mathematical models: models are merely rules produced by a training algorithm which is activated by the data fed to the system. In any case, such technical complexity should not exclude those who have designed and implemented a system that makes decisions for the community from responsibility.
“He who controls the past controls the future: he who controls the present controls the past,” wrote George Orwell.
If we want to formulate the question in philosophical terms, we can borrow the words of Remo Bodei: “Won’t we then end up becoming hetero-directed and won’t we need to increase our vigilance against these mental Trojan horses and stick, so to speak, to a sort of manual of self-defense against intrusions into the sphere of our thoughts, images and passions?”
We are the result of our choices; we live in the space of choices. If we reduce this space, because a machine has already decided to offer us a reduced and arbitrary version of the spectrum of possibilities, we do not exercise an effective choice of value. It would then be like falling into a funnel where our values fatally cancel out.
How the decision-making process takes place when someone has already decided for us is a political issue, because it concerns both who has the power to decide and the basis for the decisions made. But what happens when something is about to decide for us is a philosophical problem, especially at the moment when that something is becoming someone; that object is becoming a subject.
Until yesterday, AI was no more than a physical extension of our body and some limited cognitive functions, but today it is assuming – because of the increasing agency we delegate to it – an autonomy of its own. When an object experiences the world autonomously and interacts through language, the gap that separates it from a subject tends to close. Algorithmic subjectification no longer invokes an “us or them” dichotomy. If we succeed in externalizing the marvelous biological and cultural synthesis that Nature has created in millions of years, with the achievement of humanity as we know it, onto a technological platform of superior performance, we will have reached a great goal. But such an artificial body will be an entity lacking human ethics and intelligence; it will be an artificial unconsciousness.
Humans see Artificial Intelligence as machines capable of making decisions for them, but they are wrong in this because such artifacts are merely calculators of symbols, albeit of increasing sophistication. Artificial Intelligence sees the human being as a set of numbers, but wrongly so because consciousness is incomputable. To know how Artificial Intelligence works, we must get close and look inside it. To understand how we can use it, we need a measure of distance in both time and space. Without proximity it is difficult to understand, but without distance we cannot imagine the consequences of its application in the world. And besides being shaped by natural evolution, the world is actively created by the cultural development of us humans, with the support of technology.
When an object experiences the world autonomously and interacts through language, the gap that separates it from a subject tends to close.
Forced to manage an increasing amount of information, we invented writing and started externalizing certain functions of our brain, extending them in space and time. So now the key question is: are we aware that we are externalizing various functions to the power of an unconscious machine?
Yes, and we’ve been doing it for thousands of years. Tracing out symbols on the sand means transcribing thoughts from a biological medium to one made of silica. Then from silica, we applied technology and made silicon, energized it with electricity and made it efficient and powerful. And thanks to the digitization of information, knowledge has become collective, global, and instantaneous.
As for the cultural base, this has been passed down primarily through language and later through writing. It spread rapidly with printing and exponentially today by technology.
We could classify technology into three levels:
- simple, requiring action, extending, and strengthening our manual skills. For example, a hammer and scissors;
- automatic, which automates the rules we’ve entered. For example, the robot and the computer;
- autonomous, delegated by humans, which processes information with the rules it has autonomously “learned” from reality. Artificial Intelligence is an example.
Autonomous tools are designed with a goal in mind and adopt a behavior without having the autonomy to choose their own goals. AI does not operate with an autonomous goal, we set it. We then think of AI as a tool, not an objective. Goals are defined by values, and values should not be determined by technology or algorithms, but by people who must decide what AI should do and how. Some goals are given by Nature and are therefore involuntary: think of what we bear in our DNA, for example reproduction. Other goals are defined by culture.
If these new autonomous machines were to ask us, “Where did we come from?”, we would be unable to answer by showing them the programming code because, as we shall see, this code does not exist: it is merely a set of rules that emerged from the data and lack an explanation. One day, they will be able to ask us all the questions they want, but we are already certain that we won’t know how to answer them (this is the problem of the black box). We, on the other hand, cannot turn to the Creator of the universe because, if he exists, he is supernatural – outside of space, time, and the laws of Nature. Our position in the universe is peculiar, but Artificial Intelligence is becoming as mysterious as we humans are.
We need to believe in something bigger, even without having the proof, and we simultaneously believe we can rule everything, including Nature. Moreover, everything we have created is not intelligent, or at any rate has always shown a lower level of intelligence, even in the face of abilities superior to ours. But the dream of being creators is made explicit in language when we assign names to objects, as if, for example, the term “learning” in machine learning had the same meaning for us. Unfortunately, as we relate to machines, we lack a neutral vocabulary with which to describe artificial phenomena. So, let’s define what “learning” means better. For humans, learning means permanently changing the neural map of the brain and, in the case of language, adding symbolic meaning.
The machine does not decide which activities to perform and above all it cannot explain why it has chosen to do it a certain way: we decide to make the machine do a job. This is why machines force us to give concrete, unambiguous signs and acts. Ambiguity, uncertainty, and intentionality are what differentiate us and what keep us from becoming artificial. These activities are so complex that we do not yet see how machines can autonomously develop them.
Adding system 0 to Kahneman’s model
The brain is difficult to understand because we experience it from the inside: we would have to go outside the body to be able to study it. The physicist Carlo Rovelli nails us to our limits: “We are more complex than our mental faculties are able to grasp. The hypertrophy of the frontal lobes is great, it has allowed us to reach the moon, discover black holes and recognize ourselves as cousins of ladybugs; but it is still insufficient to clarify us to ourselves”.
Clarify us to ourselves. It is from Socrates onwards that we have said: know thyself.
Our knowledge of the brain, about whose functioning we are still speculating, is also proceeding relatively slowly. Now we look at the book by Daniel Kahneman, Slow and Fast Thinking, which describes the research that won him the Nobel Prize (together with the late Amos Tversky) and laid the theoretical foundations for the revolution in economic science called “behavioral economics”. In particular, a phenomenon studied in this discipline is the “framing effect”, which concerns the impact of the way information is presented on decision-making processes. To describe our cognitive processes, Daniel Kahneman uses the concepts of System 1 and System 2. System 1 “functions automatically and rapidly, with little or no effort and no sense of voluntary control.” It is responsible for all the things we don’t have to think much about, such as washing dishes, throwing a ball, or buying undifferentiated goods. System 2, on the other hand, performs more demanding mental tasks that require cognitive deepening, such as performing complex calculations. System 1 is fast, intuitive, and emotional, while System 2 is slow and logical. An example of System 1 thinking is detecting that “one object is farther away than another,” while an example of System 2 thinking is “finding a way to park in a confined space.” Using the two Systems, Kahneman describes how decision-making processes are formed, with all the associated biases.
A fundamental thesis of Kahneman is that we humans tend to identify with System 2, “the conscious, reasoning self that has beliefs, makes choices, and decides what to think and do. But the one that is responsible for most decisions is actually System 1, since it effortlessly originates impressions and feelings that are the main sources of System 2’s explicit beliefs and deliberate choices.” Most of the time System 1 starts automatically, while System 2 works in the background, in low-effort mode. System 1 works to simplify the understanding of reality so as to conserve energy and resources for System 2.
When the two systems agree, impressions become beliefs, and when System 1 gets in trouble, it asks System 2 for help. System 1 works very well most of the time, but it makes systematic errors, and much of Kahneman’s research focuses on identifying these errors. As he admits, identifying them is one thing, but avoiding and accepting them is another, because automatic, unconscious processes are the basis of intuitive thinking.
The basic concept is that System 1 applies intuitive, quick, parallel, efficient but sometimes incorrect decisions, while System 2 is serial and analytical, but lazy and prone to follow its own biases. When placed in an evolutionary context, the framing of the dual model makes immediately understandable sense: while System 1 served us to run from predators, System 2 served us to build encampments.
One of the key proposals in this article is to extend Kahneman’s model: the time has come to add System 0. System 0 is any software that acts as an intermediary between us and reality, which it frames and presents to us in a particular version. Kahneman writes that “intuitive System 1 turns out to be more powerful than our experience tells us, and is the secret architect of many of our choices and judgments.” But what happens when our System 1, which he calls “automatic,” is powered by System 0?
The proposal to add System 0 may sound like a literary gimmick, a simple layer added to Kahneman’s, but in fact it is fundamental for understanding how we are evolving. Let us remember, however, that System 0 is only a simulation of human reasoning: its decisions are not real “decisions.”
System 0 has two specific functions: to learn from reality through a mix of classification and/ or prediction, and to suggest decisions. In synthesis, it elaborates data but has neither the instinct, which instead belongs to System 1, nor the reasoning of System 2, nor the consciousness of itself or the context.
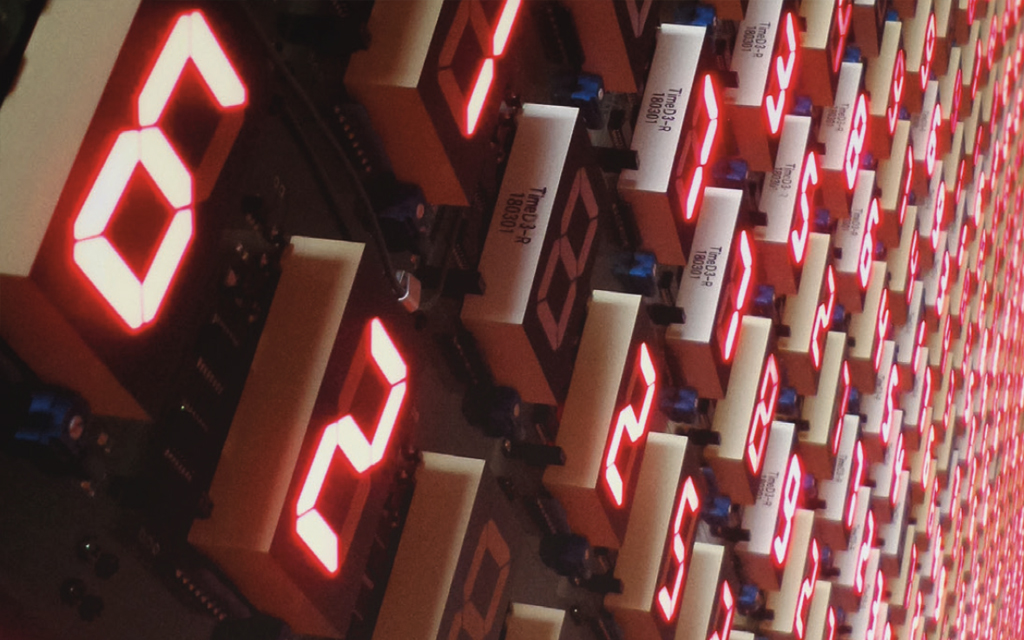
Consider the flows between these systems. We perceive reality directly through our senses. With technology, which can be autonomous, we can have more detailed or synthesized information. This information feeds our System 1, which reacts instinctively. If more accurate reflection or calculation is needed, System 2 takes over. But first and foremost, there is the immediate and direct relationship between us and AI which is destined to become, in an increasingly complex world, not one but the source of information. Consciousness is the very essence of our existence. To explain how AI lacks it, we resort to the American philosopher John Searle, taking in particular his experiment of the “Chinese room”, a fundamental criticism of the idea of a true Artificial Intelligence. For him, if Artificial Intelligence is not conscious, then it cannot be intelligent either.
According to Searle, the execution of a program, i.e., the simple manipulation of symbols, is not a sufficient condition to explain understanding. The heart of his argument is a mental experiment in which he imagines a lonely subject closed inside a room, who has all the necessary instructions to respond appropriately to some questions written in Chinese (a language that the subject does not know). To those who are outside the room and analyze the answers, it seems that “the room” knows Chinese, when in fact it is a manipulation of symbols. Therefore, you can’t say that a computer understands a language just because it is able to “use” it. In Searle’s reasoning, understanding cannot be the manipulation of formal symbols. The computer cannot understand human language, but can only be able to formally analyze syntactic structures. Guido Vetere, professor of philosophy at Marconi University in Rome, writes: “Technological storytelling tells us that if machines slightly succeed at something today, then tomorrow, with greater power, they will entirely succeed at that thing. The argument works if there is a clear quantitative relationship between things. But there is a quantum leap called semantics between word recognition and sentence comprehension. The greater capacity for calculation does not translate into greater capacity for comprehension until the latter is traced back, precisely, to a calculation.”
Semantics is meaning, the vast body of shared experience that for humans is implicit and unwritten, but which underlies language. All the knowledge that each of us carries is a set of data that machines cannot access. As a consequence, they cannot produce a model from data they do not have available, and they cannot help us when we try to understand why they produced those results that we – wrongly – consider decisions. The semantic component is not reducible to formal syntactic operations.
In essence, the machine does not understand, at most it transduces, that is, it transforms one set of symbols into another, but it ignores the meaning. We, on the other hand, have something more than uninterpreted symbols: meaning.
Everything we have written so far has referred to the attempt to make the machine understand texts written in various languages. But there is another language that we invented to instruct it, coding, that is a set of high-level instructions (Java, C, etc.) that allow programmers to implement their ideas and thoughts without having to represent them in the form of zeros and ones. We should ensure that computer coding is open to all, because it is the language that brought us to the highest form of civilization, and will determine the future one. Therefore, will we move beyond coding to instructing machines directly talking to us? Perhaps yes, but hopefully we will understand, and be able to judge, their responses.
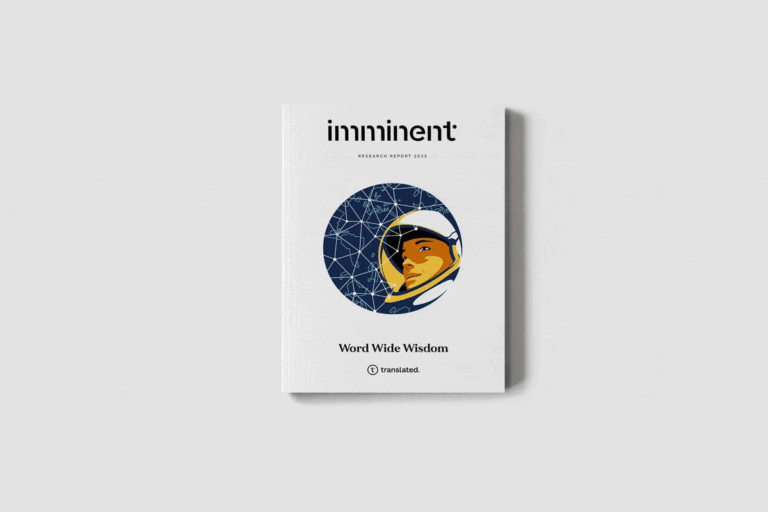
World Wide Wisdom
Research Report 2023
It is possibile to improve the understanding between people that speak different languages and thus improve their ability to do things together in a smarter way? Can it be that a multilingual group is able to do better things? In order to answer to these questions we need to take into account how groups of people think and work together and how their collaboration can be improved.
Get Your Copy Now!At this point the question is: do humans provide the system with intelligence or is it the system that learns because of its “intelligence”? The answer is certain: despite advances, AI remains far from human intelligence. We have developed the neocortex for reasoning, abstraction, etc., but we obey orders (from System 1) that do not reach the threshold of awareness (System 2); we obey almost like automatons and even risk Homo sapiens placing itself at the mercy of AI. To understand how we make decisions, we must turn our gaze to the third hemisphere. Because after the left and the right, we have a third hemisphere of the brain, which is external: System 0. It is usually believed, correctly, that technologies are not neutral, but in the case of AI it is even more opportune to remember that not even data are neutral: the large data sets fed to algorithms represent the stratification of years and years of distortions and prejudices; that is, they show certain underlying realities of our societies that we often tend to remove. And the machine, as we have seen, makes its calculations from these data. Before using it, therefore, we need to document how this particular statistical machine is designed, how it gradually learns from the data it observes, what data is fed into it, and how it is transformed into a comprehensible language.
Even if the tool works in an obscure manner, we are duty-bound to choose the goal, which must always be transparent. Therefore, as in the case of our biological brains, in order to explain the behavior of AI, we are forced to confront questions in terms of both “nature,” i.e., how the algorithms are constructed, and “culture,” i.e., what are the input data. We must be honest and precise in our use of language and avoid deceiving ourselves by saying that an actual AI, in some of its implementations, can explain “how it decided” to us. Moreover, we must accept the fact that our brain is the first, true black box. Specifically, the association between word, thought, and reality takes shape in our cerebral processes, but machines can observe only what we externalize of these processes; as the linguist Tullio De Mauro stated: “Words are tangential to things: that is, they touch them in certain points, but lightly. Finding the coordinates of these points is not a matter of computational power or algorithmic acumen, because these coordinates are established, creatively, in societies.” The implication is that human action shapes AI’s inputs and is influenced by its outputs.
Conclusion
Our descendants are guided by biology: it is our nature that generates theirs, and after birth, they learn through culture. For AI-based machines, on the other hand, we are guided by science: it is our culture that generates their nature, after which, by training, they learn from experience and create the model we apply to reality.
Since humans adopted upright posture, they have freed their hands, written symbols, developed language, and cared for their young for a longer time, giving them a competitive edge. All this has boosted our cognitive capabilities. We are now delegating certain cognitive, manual, and repetitive functions to System 0. It would be undesirable for the impact of ever-increasing amounts of data, the low cost of computation and the dominance of cloud computing led us to avoid focusing on our goals. Of course, in a time of increasing confusion and uncertainty, overwhelmed by data to be interpreted, delegating is the convenient recourse, but it is never the ultimate solution.
The great philosophical reflection we are currently facing is surely the breaking down of the boundary between what we consider natural, biological, and living and what we consider mechanical, technological, and inanimate. In his book Come saremo (How we will be), Luca De Biase and Telmo Pievani state: “In reality, technologies are co-evolving with us, always in symbiosis: we are not two separate worlds, but mutually connected parts within the same co-evolutionary process […] Technology is becoming more and more biological, and biology is becoming more and more technological”. There is no opposition between subject and object, but complementarity.
Let’s not be confused: we must govern not only AI but also ourselves to prevent the first inventor – or creator – of the superior AI or the institutions in control of it from subduing humanity. In the search for a dynamic equilibrium between the optimistic outlook of technology and the “pessimism” of our own lazy cultural adaptation, our position must be clear: we must always be intolerant of AI’s erroneous prediction, which cannot be corrected without our help; and we must also be intolerant of the creation of AI that do not meet ethical criteria. In reality, where human discretion exists, we not only self-correct but also apply forms of meta-learning, which is precisely what AI does not yet know how to do: learn how to learn. This is the only way we can cope and manage novelty. We must act with elusive common sense – precisely what machines cannot have.
Common sense is the body of implicit knowledge that we do not store on any medium because it is neither possible nor expedient to do so. Common sense includes our understanding of physics (causal relationships, perception of heat and cold, etc.), as well as our expectations of how beings, human and otherwise, behave, as in the case of a snowman, which self-driving cars mistake for a person standing at the side of the road. So much for losing games of chess: we are entirely something else!
AI can only be a global issue, so we need to strengthen international cooperation on the subject, but how can we achieve this difficult goal? We could reach it by establishing international research centers, increasing investment in research, boosting immigration visa policies to attract talent from other countries, creating multipolar centers of digital innovation, making data available for AI development also in local languages, and fostering privacy regulation through international public-private partnerships. The preferred sectors for launching the first processes are environmental issues and public health, given the global scope and impact.
Philosopher and artist Salvatore Iaconesi, a devotee of the “art of sharing,” wrote:
“We can’t do things alone anymore. There are no longer things that can be addressed from a single point of view or through a single discipline. In the global, hyper-connected world, even the simplest things pose questions that need to be addressed by a multitude of different disciplinary approaches because they can only be addressed through changes of state. In this case, technique (like art) is about knowing and interconnecting knowledge and people.”
Iaconesi, who shared all the data on the disease that affected him, was a true pioneer. Technology improves our world, expands our horizons, and affords us longer lives, but it is only a lever that expands the potential of human relationships. Humans like things that don’t change over time: the need to communicate, the ability to move and love, etc. Unlike AI, we are free, responsible, and conscious; observing an infinite world, we ask ourselves infinite questions with an equally infinite thirst for knowledge.
The great adventure on the immediate horizon is in the hands of people, not machines. It is we citizens who must decide how to distribute the benefits and control the risks associated with the spread of Artificial Intelligence. We must ensure that everyone is aware of and shares the dangers of monopolistic concentration, inaccuracy, and the pursuit of evil ends. As humans, we have a duty to act ethically. As the jurist Stefano Rodotà asked: “Is everything that is technically possible also ethically admissible, socially acceptable, legally licit?”
For these reasons, neither utopian nor dystopian scenarios will prevail: the future will depend not only on natural selection and environmental constraints but mainly on the choices and omissions we will make in the field of AI. The question is not “What will happen?” The correct question is “What should we make happen?” “Tomorrow I will be what I choose to be today,” said James Joyce, because it is decisions, not predictions, that have consequences.
The future will judge us.
Bibliography
1 R. Bodei, Dominion and submission, Il Mulino, Bologna 2019.
2 C. Rovelli, The Order of Time, Adelphi, Milan 2017.
3 D. Kahneman, Slow and fast thinking, Mondadori, Milan 2012.
4 J. Searle, Is the mind a program?, The Sciences, 1990, no. 259.
5 G. Vetere, Robots without common sense, Il Sole24Ore-Nòva, 22 January 2017.
6 L. De Biase, T. Pievani, Come saremo, Codice edizioni, Torino 2016, Capitolo 8. Cascate di effetti inaspettati, Capitolo 9. Scorci sul possibile tecnologico adiacente.
7 Salvatore Iaconesi, Il primo giorno di una nuova scuola, chefare.com
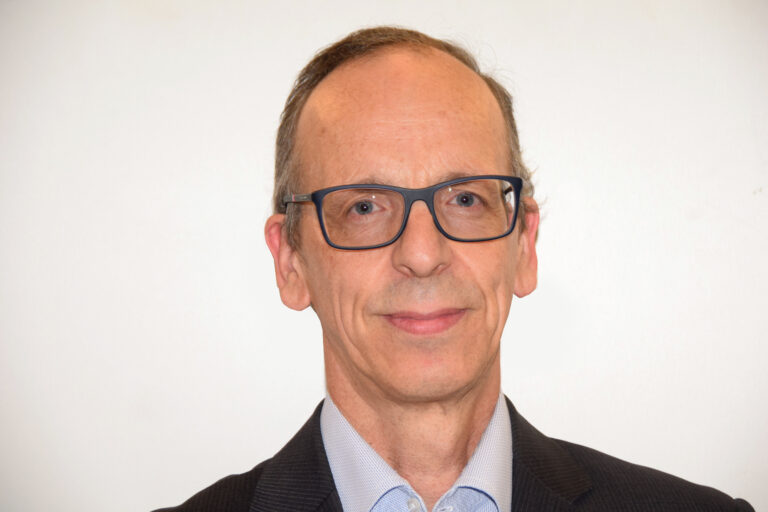
Massimo Chiriatti
Chief Technology and Innovation Officer for a multinational company
Massimo Chiriatti, Chief Technology and Innovation Officer for a multinational company, collaborates with universities and research consortiums for training events on the digital economy. Adjunct professor at LUISS University for the master’s course in Data Science and Management (Ethics for AI). Member of the board of experts appointed by the Ministry for Business and Made in Italy to develop the national strategy for technologies based on shared registers and the blockchain. Edited the insert “Le monete virtuali – Lezioni di futuro” (Virtual currencies – Lessons of the future) published by Il Sole 24 Ore-Nòva100. With Luciano Floridi, co-authored the scientific paper “GPT-3: Its Nature, Scope, Limits, and Consequences”, published in Springer, Minds and Machines. Author of the book “Humanless – L’algoritmo egoista” (Humanless – The selfish algorithm) published by Hoepli in 2019. Author of the book “Incoscienza Artificiale” (Artificial Unconsciousness) published by Luiss Press in 2021. Co-author of the Manifesto on Artificial Intelligence in 2.
Photo credit: Margaret Weir, Unsplash