Technology
Natural language technologies bring AI closer than ever to human intellect, but a semantic issue might stand in the way. An exclusive article penned by Guido Vetere, Adjunct Professor of Artificial Intelligence at the Faculty of Applied Sciences and Technologies of the University of Guglielmo Marconi and leader of IBM’s Center for Advanced Studies.
2020 will be remembered in part as a year in which natural language technologies took another significant leap forward. This is thanks to OpenAI, a Silicon Valley non-profit research company founded in 2015 by Elon Musk and now financed by Microsoft (among others), whose stated mission is to ‘democratise’ Artificial Intelligence (AI) – in other words, to make its benefits accessible to all. In May of this year, OpenAI released the third version of its Generative Pre-Trained Transformer (GPT-3), a neural language system capable of answering questions, developing topics, having a dialogue and translating, in ways that are often indistinguishable from a human. And it is not just the system’s performance that is startling; it is also the fact that this performance is obtained without having to train it for each specific purpose.
We are therefore looking at general linguistic intelligence, similar in this respect to a human’s. Its capabilities are such that the developers themselves have warned that the system could be used to facilitate activities posing a threat to society. In fact, just a few weeks after it was launched, a student in California had already started a blog automatically generated by GPT-3. Fortunately, it turned out to be harmless. A short time later, the Guardian published a bogus article written by the AI, intended as a stern warning.
Natural language (NLP) technologies are one of the primary sectors of AI, not just because of their immense economic potential, but also because they directly tackle the issue of human intellect.
Natural language (NLP) technologies are one of the primary sectors of AI, not just because of their immense economic potential, but also because they directly tackle the issue of human intellect. All intelligent technologies are in some way reminiscent of the cognitive processes of our species: for example, consider autonomous driving, where it is important to be able to classify the shapes visible from the vehicle in a way similar to the normal process of the person behind the wheel. Language, however, is the very way in which our consciousness represents the objects that our senses deliver to us. In fact, according to some theories, it plays an active role in the identification of these objects. It would be no exaggeration to say that the Artificial Intelligence project, outlined by Alan Turing in the 1950s, essentially revolves around creating full linguistic intelligence.
The capabilities of GPT-3 might lead us to think that the ‘singularity’ heralded fifteen years ago by Ray Kurzweil – i.e. the point at which robots become so powerful as to exhibit something comparable to human consciousness – is indeed imminent. Upon closer inspection, however, this AI reveals how far we still are from that moment and tells us something about the directions that research may take in the near future. But it also tells us something very important about the future of the indissoluble relationship that has been established between human societies and information technology.Â
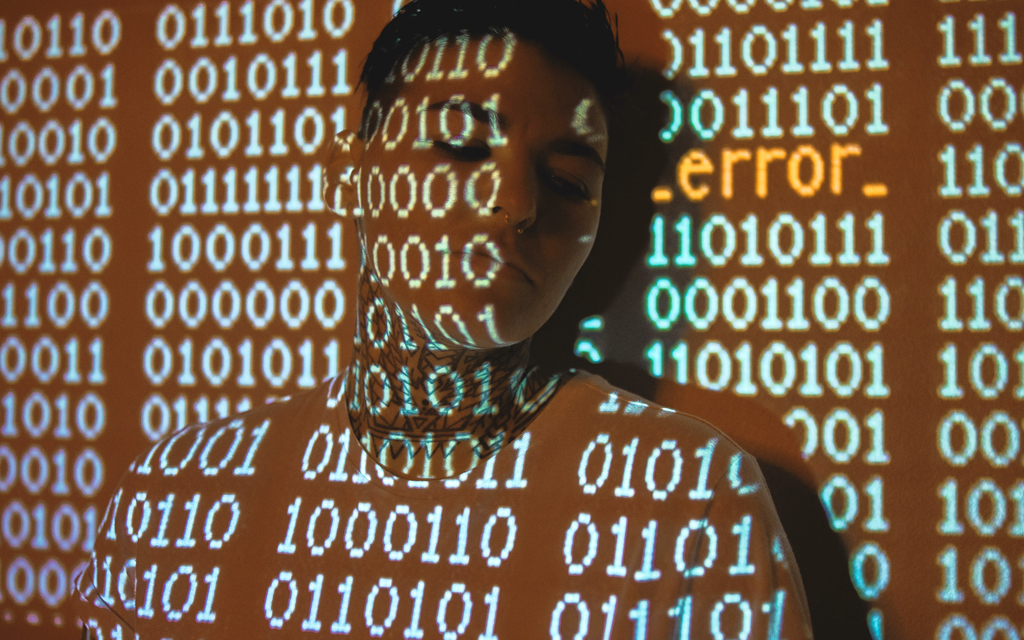
GPT-3 is a neural network with 170 billion connections, trained using petabytes of text found on the web in various languages. Like many neural language technologies developed recently (notably by Google, among others), GPT-3 makes use of what specialists call ‘unsupervised learning’: the system learns by reading texts that are submitted to it as they are, without any human annotation. A highly simplified summary of the procedure is as follows: a word is ‘masked’ in each sentence, and the neural network must learn to guess what the word is based on all the similar sentences it can observe. The way in which the sentences are codified for the use of the algorithms plays a decisive role, and the search for these transformations is the defining issue for this kind of research. The result is a ‘linguistic model’ in which each word is associated in a sophisticated manner with the contexts in which it is used. Generating a text based on the model means producing the most likely sentences on the basis of the observed examples; in other words, producing sentences that sound as similar as possible to the typical ways in which language is used in the training texts.
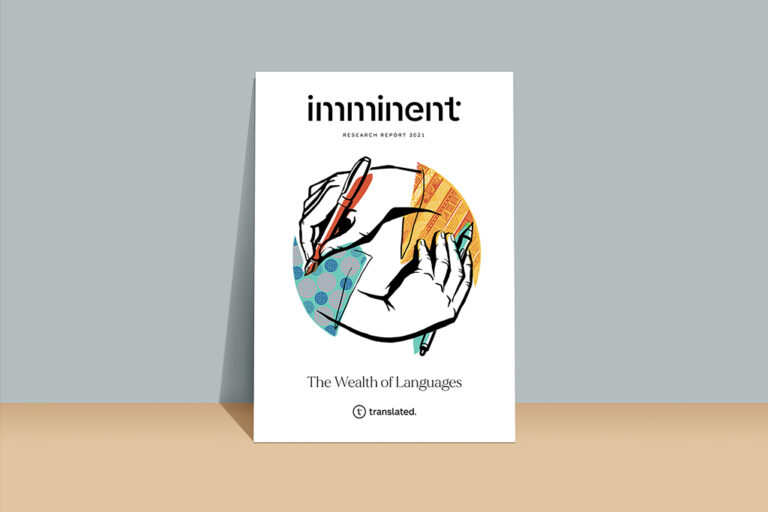
Imminent Annual Report 2021
Fit your business in global shape. Get your copy of Imminent Annual Research Report 2021. And let us know what you think.
Get your copy nowGPT-3’s training process is a colossal undertaking that few would be able to replicate, even just for the fact that it requires millions of dollars’ worth of computing infrastructure and electricity. Its language model is not distributed for free, even for non-commercial purposes: it can only be activated on the servers of an IT giant. In this particular case, the giant is Microsoft, which will enjoy exclusive use of OpenAI’s technology to enhance its services. Moreover, deploying and using a neural network of this size would be by no means an easy task: few organisations would have the computational resources to employ it efficiently. For the moment, therefore, the democratisation that OpenAI claims as its mission does nothing more than further consolidate the existing dictatorships of the info-sphere.
Neural network designers who approach language see it as a space combining strings such as words, roots, morphemes, or even individual alphabetical characters. These elements are not symbolic (sýmbolon, bringing together), since to machines they mean nothing more than numbers do. In fact, the only things these automata can learn are the syntactic relationships (from sýntaksis, arrangement) that these numbers exhibit in the sequences where they occur, i.e. in the texts. Together, these combinations form an immense algebraic space. The view of mathematicians is that the shadows of meaning are cast in this space; there is even a theory that these shadows account for the conceptual content of words better than dictionaries can.
In the days of classical AI (the 1980s), John Searle observed that a machine programmed to translate from a totally unknown language (Chinese, in his example) was like a person locked in a room who received messages in Chinese from the outside world and used a correspondence table (i.e. a sort of dictionary) to translate them, despite understanding nothing of what he or she was reading. For the AI of today, the dictionary does not even exist, and there is no longer anyone in the room. There is only the immense combinatorial space full of data, and enormous computing power.
The idea underpinning the linguistic technologies of today might appear a technocratic delusion. However, some argue that it has its roots in twentieth-century linguistic thought. Representation based on embedding, as is typical of neural networks, shares similarities with the distributional hypothesis generally attributed to Harris, namely the observation that words appearing in the same contexts carry similar meanings. For each word, in effect, an embedding is (roughly speaking) a set of other words that frequently appear in the samecombinations. Wittgenstein’s notion of a language-game has also been evoked to emphasise how, when it comes to meaning, a data-based approach can be more accurate than classical lexicography and its computerised counterparts. Indeed, Wittgenstein examined the meaning of words in terms of their use, something which can be tracked in texts to a certain extent and thus quantified (with the right algorithms).
However, neither Harris nor Wittgenstein ever suggested doing away with dictionaries. Besides, it is highly unlikely that any analysis of the combinations of words in texts, even if very sophisticated, could convey the explanatory richness of a dictionary entry. In reality, in linguistics, distributionalism is more of a method for testing hypotheses and is especially useful for the study of non-written languages – certainly not for building theories. As for language-games, these occur in real-life situations where a word acquires meaning when it comes into contact with entities, events, and interlocutors. Written documents present a very partial and mediated view of this coupling between phrases and situations, a view which is in any case incomprehensible without concrete experience and knowledge of the world.Â
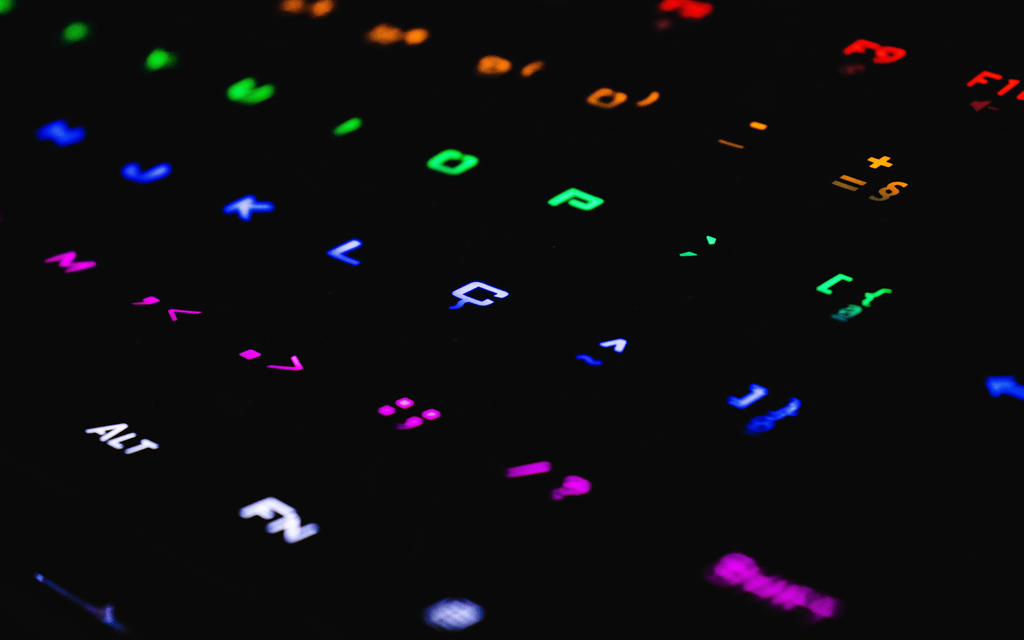
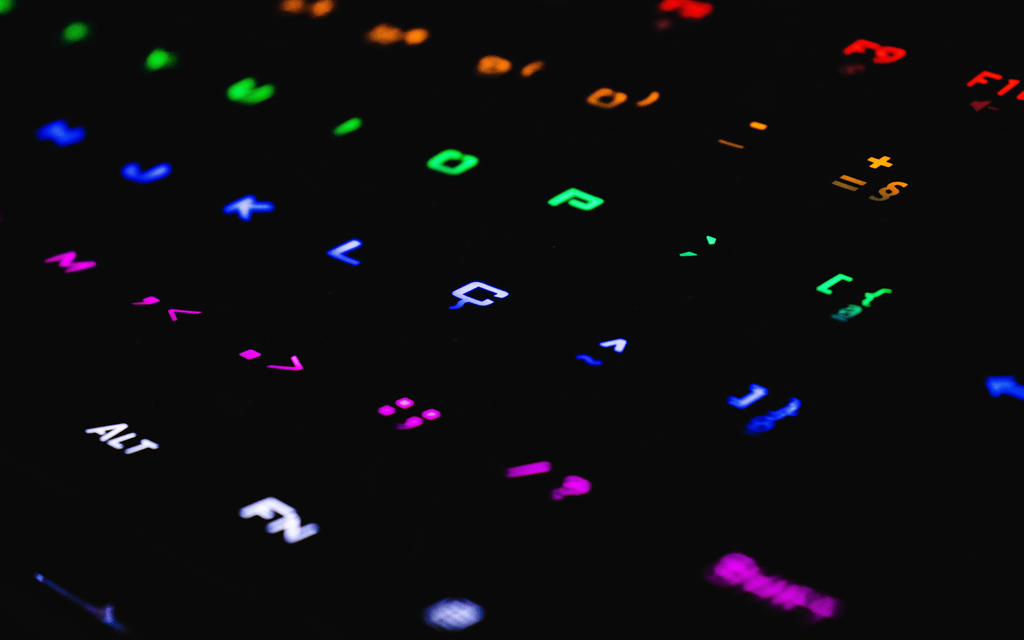
With the data and the computing power currently available to us, the linear algebraic methods of neural networks are very advantageous from a technical standpoint for certain specific purposes; this makes the quest to justify them using classical linguistics and philosophy somewhat suspect. In fact, according to the semiology constructed by Ferdinand de Saussure at the turn of the last century, built on ancient foundations, a linguistic sign is a unit where something (the signifier) stands for something else (the signified), and the signifier says nothing regarding the meaning if not by virtue precisely of the sign itself (the principle of arbitrariness). The sign, therefore, cannot be divided into its components, just as a molecule cannot be reduced to the sum of its atoms, ignoring the bond established between them. Not surprisingly, neural linguistic systems effectively evaluate the similarity between sentences, while relationships of logic and entailment that require in-depth semantic knowledge seem substantially beyond their reach.
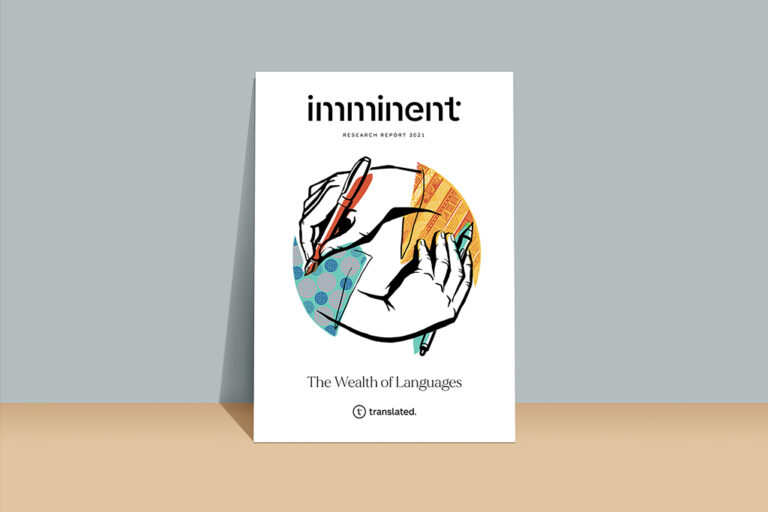
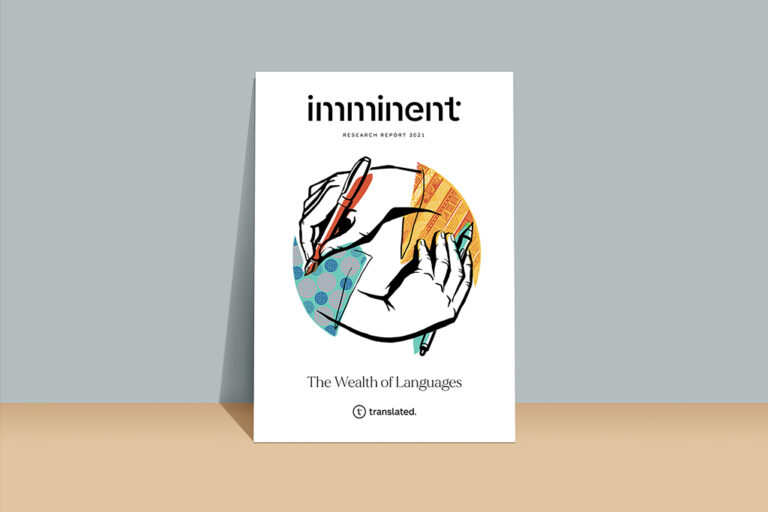
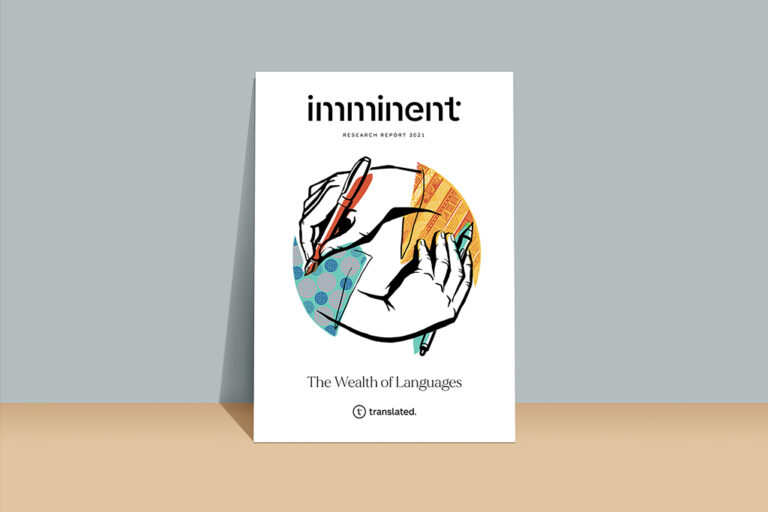
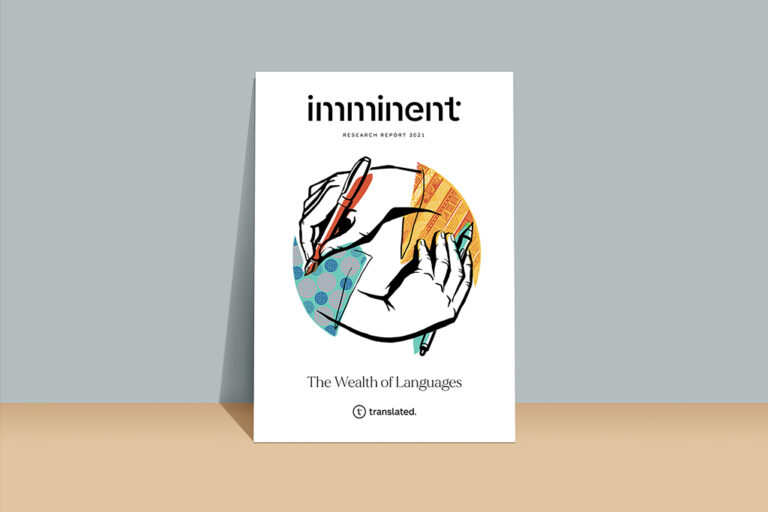
Imminent Annual Report 2021
Fit your business in global shape. Get your copy of Imminent Annual Research Report 2021. And let us know what you think.
Get your copy nowIn any case, it is worth clarifying that the primary objective of linguistic AI is not to trace the arrangement of meanings from the arrangement of signifiers; in general, linguistic AI does not aim to explain how or why this operation can be carried out. Rather, it pragmatically exploits the fact that often, within certain limits and for certain purposes, this process actually works. While physics and chemistry have clear answers to the question of what holds atoms and molecules together, AI makes no claims with regard to the unity of linguistic signs – perhaps implying that this information is dispensable. Moreover, if AI did want to seriously tackle the topic of semantics, it would have to wade into the philosophical jungle of the theory of meaning, where it is difficult to survive armed only with data and linear algebra
“Moreover, if AI did want to seriously tackle the topic of semantics, it would have to wade into the philosophical jungle of the theory of meaning, where it is difficult to survive armed only with data and linear algebra.”
In fact, the meaning of words was a central problem in twentieth-century philosophy, which was to a large extent a philosophy of language. Meaning – i.e. the fact that words, one way or another, in the situations or texts in which they occur, refer more or less regularly to the same thing for the majority of speakers – is such an immersive phenomenon that it seems obvious and very simple to us. On the contrary: this phenomenon brings into play the relationships between the individual and society, between creativity and imitation, between imagination and reality, between chance and necessity, between will and justification, between belief and knowledge, between aesthetics and pragmatics, between deduction and intuition – in short, it brings into play all the dialectics that make humanity what it is. The desire to reduce all this to algebra is somewhat reminiscent of the alchemists who, thanks to the coniunctio oppositorum, believed that they could turn lead into gold.
However, the desire to think of language as a calculation has always been very strong, even in times when this calculation had no benefit: we need only mention Leibniz. It is therefore no surprise that the linguistic triumphs of AI today, in addition to significant economic interest, also trigger a certain intellectual excitement alongside apprehension and sombre predictions. Whether or not this excitement and apprehension are justified, the fact remains that building an automaton like GPT-3 does not produce a theory of language, just as building the dome of the Pantheon did not provide the Romans with any scientific notion of statics. Without a good understanding of the contours of the technology and the limitations of AI methodologies, it is only a matter of time before we are yet again disillusioned.
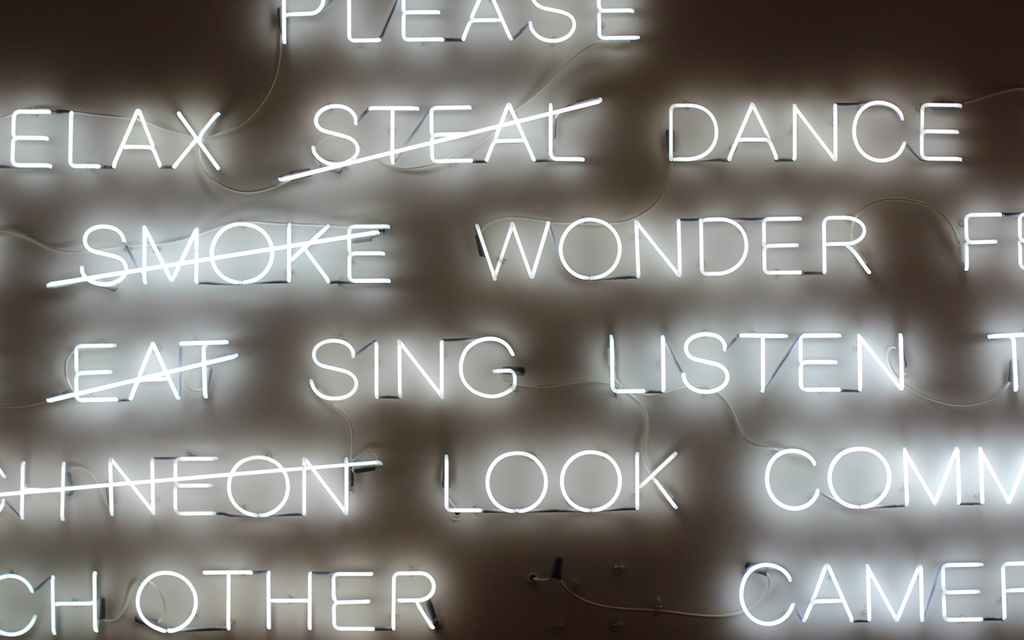
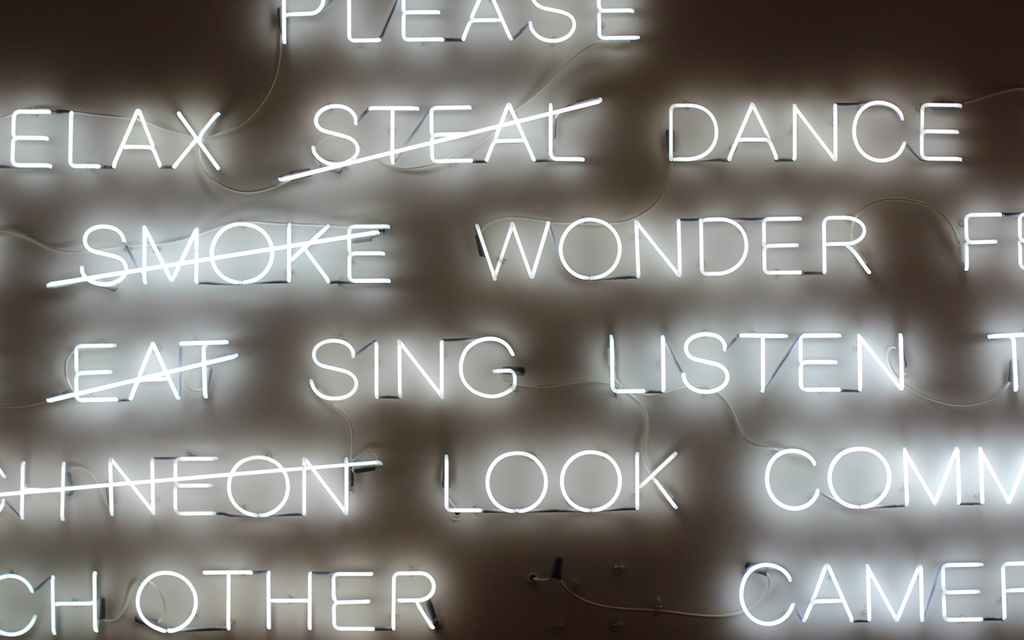
Although they may be adept at imitating our language, unsupervised neural networks like GPT-3 are unable to move from surface-level expression to conceptual content – in other words, to carry out the basic function of language. This becomes clear as soon as we carry out systematic testing. Without this ability, it is very difficult for an AI to get to grips with the innumerable puzzles of our linguistic life; those thousands of little mysteries that we solve every day without even thinking about them thanks to our experience of the world. For example, in the newspaper headline ‘Stolen painting found by tree’, we understand that it was not the tree that discovered the stolen goods, but rather that ‘by’ in this case indicates ‘near to’. Although some people, such as Yoshua Bengio, believe that this kind of reasoning (known as ‘common sense reasoning’) can be achieved by enhancing existing neural networks, many, like Gary Marcus, argue that there are actually intrinsic reasons for their inabilities in this area.
“The original sin of the unsupervised linguistic neural networks that astound us today is clear: the splitting of the semantic atom. Without taking into account words in their entirety as signs, AI will never achieve total mastery of language.”
The original sin of the unsupervised linguistic neural networks that astound us today is clear: the splitting of the semantic atom. Without taking into account words in their entirety as signs, AI will never achieve total mastery of language. With GPT-3, we have probably reached the limit of what can be done with syntactic/statistical methods. Subsequent (and even larger and more expensive) versions of the neural network may improve in some respects, but they will not go beyond the limit established by the very approach they adopt: an approach that does not delve into the substance of signification processes.
Classical AI, with its ‘semantic networks’, started out with a perspective diametrically opposed to current trends: the approach of codifying meanings one by one and then interpreting texts in light of these explicit hypotheses. This ancient practice still endures, especially in certain niches and for the tasks where we cannot do without it; in general, though, it has been rejected as it is so difficult and laborious to implement. However, a great deal of research today is focused on the use of next-generation conceptual models (known as ‘ontologies’) and their integration with powerful machine learning technology, precisely to compensate for the semantic ignorance of neural networks. This approach, in addition to developing superior operational capabilities, would also offer the benefit of auditability: a hugely important requirement when entrusting ethically sensitive tasks to machines.
But this initiative also appears to be limited by what Quine called the inscrutability of reference: even if a linguistic concept is formally codified, the fact remains that we cannot fully regulate or investigate the way in which it may be interpreted by the different humans and automata that populate our interconnected global communities. In recent decades, a small community of computer scientists and philosophers have applied the principles of formal ontology, established by Husserl at the beginning of the twentieth century, to ensure that these conceptual models are semantically cogent, such that they would lead to the same interpretations in different user communities. Their efforts, however, have counted for little. In fact, the ontologies that have spread across the web have remained attached to the lexicon, laden down as it is with human uncertainty. We must recognise this.
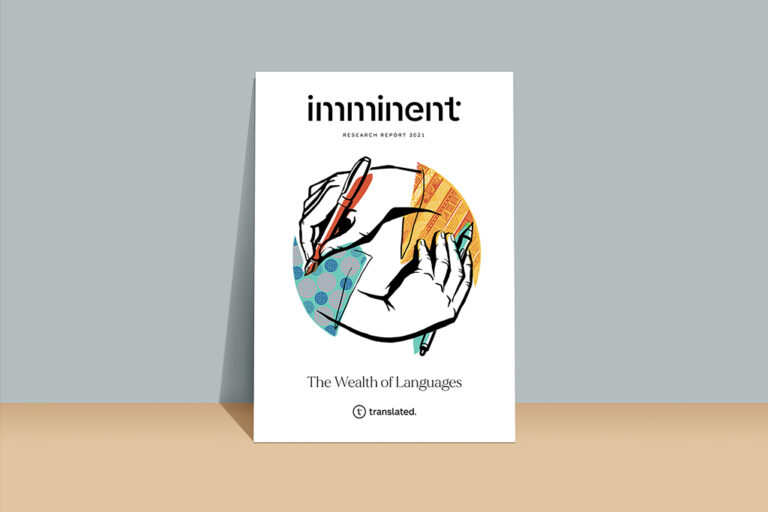
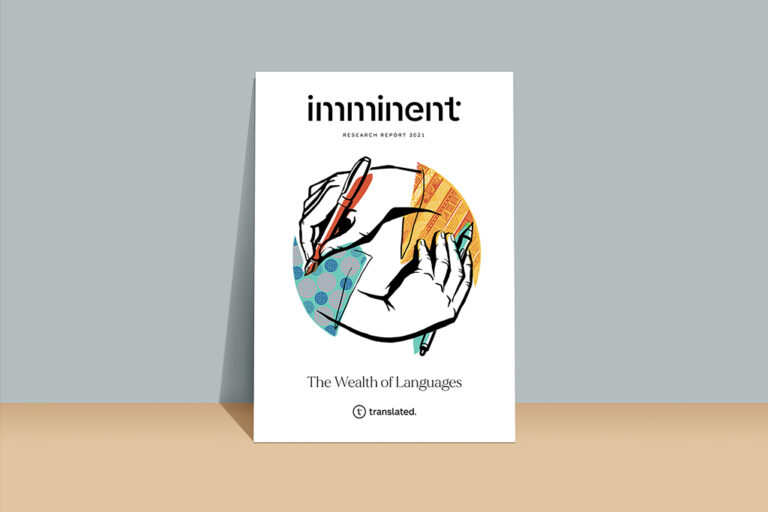
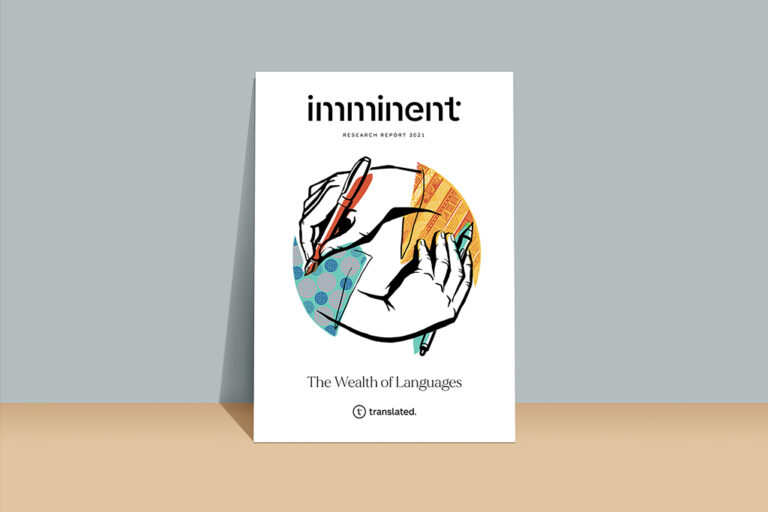
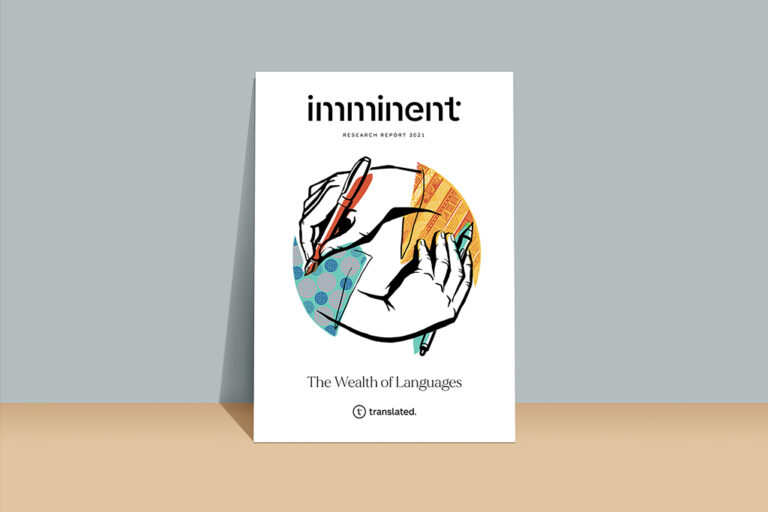
Imminent Annual Report 2021
Fit your business in global shape. Get your copy of Imminent Annual Research Report 2021. And let us know what you think.
Get your copy nowParadoxically, the spectacular progress of linguistic automata – with GPT-3 the current pinnacle – reveals the limits of neural engineering. Moreover, the explicit representation of knowledge is struggling to escape the doldrums of lexical semantics. Far from having solved the mysteries of language thanks to our engineering, we must therefore look to the future aware of two things: on the one hand, texts – even all the texts in the world – cannot help automata establish ‘linguistic awareness’, whatever this is; on the other hand, there is no metaphysics that can somehow be injected into machines, bringing to life their relationship with the world. “An awareness of these limits lies at the heart of a humanistic approach to technology that has been gaining ground for several years now. This philosophy must work in the infosphere, and the latter must grow according to this philosophy, namely with an awareness that it is developing within open problems – problems to which technology offers solutions rather than answers.
“An awareness of these limits lies at the heart of a humanistic approach to technology that has been gaining ground for several years now.”
Language Models are Few-Shot Learners
A college kid’s fake, AI-generated blog fooled tens of thousands
A robot wrote this entire article. Are you scared yet, human?
Computing Machinery and Intelligence
R. Kurzweil, The Singularity Is Near, 2005
H. Hoijer, The Sapir Whorf hypothesis, in Language in Culture, University of Chicago Press, 1954
J. Searle, Minds, Brains, and Programs, in Behavioral and Brain Sciences (1980)
Z. Harris, Distributional structure, in Word(1954)
Google Translate is a manifestation of Wittgenstein’s theory of language, Quartz, 2019
F. de Saussure, Cours de linguistique générale, 1916
Stanford Encyclopedia of Philosophy, Theories of Meaning
W. V. O. Quine, Word and Object, MIT Press, 1960
G. Vetere, Formal Ontology to the Proof of Facts, in Ontology Makes Sense, IOS Press 2019
L. Floridi, The Logic of Information. A Theory of Philosophy as Conceptual Design, Oxford University Press 2019
Photo credits: Franki Chamaki, Unsplash / Jayson Hinrichs, Unsplash / Antonino Visalli, Unsplash /
Lauren Peng, Unsplash